Deciphering molecular atlas of Alzheimer’s disease: A comprehensive bioinformatic analysis of gene expression and protein interaction networks
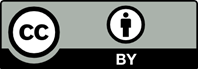
Alzheimer’s disease (AD) represents a formidable challenge in the realm of neurodegenerative research due to its complex pathology. Despite tremendous scientific endeavors, the intricate molecular underpinnings of AD remain incompletely understood, necessitating a multidimensional approach to decipher its complexity. In this study, we analyzed differential gene expression, gene ontology (GO) enrichment, and protein–protein interaction (PPI) networks using advanced bioinformatics tools to dissect the molecular landscape of AD. Initially, our research identified 732 differentially expressed genes (DEGs), which provided a comprehensive view of the genetic disruptions associated with AD. The results of subsequent GO enrichment analyses revealed that DEGs were enriched in several critical biological processes, predominantly including translation, neuroinflammation, and synaptic functionality, underscoring the multifaceted nature of AD pathology. The PPI network analysis further unveiled the central role of ribosomal proteins, such as RPL12, RPL15, RPL18, RPL19, RPL27, RPL35, RPL36, RPS16, RPS19, and RPS9, establishing a novel link between protein synthesis disruptions and the molecular mechanisms of AD. These results not only deepen the understanding of molecular mechanisms underlying AD but also illuminate potential therapeutic pathways and biomarkers for AD. Overall, our comprehensive bioinformatics exploration unraveled the complex molecular mechanisms that govern AD pathogenesis and highlighted promising new targets for the diagnosis and treatment of AD, creating a foundational framework for future research on AD.
- Hutton M, Hardy J. The presenilins and Alzheimer’s disease. Hum Mol Genet. 1997;6(10):1639-1646. doi: 10.1093/hmg/6.10.1639
- Bush AI. The metallobiology of Alzheimer’s disease. Trends Neurosci. 2003;26(4):207-214. doi: 10.1016/S0166-2236(03)00067-5
- DeTure MA, Dickson DW. The neuropathological diagnosis of Alzheimer’s disease. Mol Neurodegener. 2019;14(1):32. doi: 10.1186/s13024-019-0333-5
- Goedert M, Spillantini MG. A century of Alzheimer’s disease. Science. 2006;314(5800):777-781. doi: 10.1126/science.1132814
- Jucker M, Walker LC. Alzheimer’s disease: From immunotherapy to immunoprevention. Cell. 2023;186(20):4260-4270. doi: 10.1016/j.cell.2023.08.021
- Ballard C, Gauthier S, Corbett A, Brayne C, Aarsland D, Jones E. Alzheimer’s disease. Lancet. 2011;377(9770): 1019-1031. doi: 10.1016/S0140-6736(10)61349-9
- Asher S, Priefer R. Alzheimer’s disease failed clinical trials. Life Sci. 2022;306:120861. doi: 10.1016/j.lfs.2022.120861
- Scheltens P, De Strooper B, Kivipelto M, et al. Alzheimer’s disease. Lancet. 2021;397(10284):1577-1590. doi: 10.1016/S0140-6736(20)32205-4
- Trejo-Lopez JA, Yachnis AT, Prokop S. Neuropathology of Alzheimer’s Disease. Neurotherapeutics. 2022;19(1):173-185. doi: 10.1007/s13311-021-01146-y
- Zhang H, Zhang, Y, Li, Y, et al. Bioinformatics and network pharmacology identify the therapeutic role and potential mechanism of melatonin in AD and Rosacea. Front Immunol. 2021;12:756550. doi: 10.3389/fimmu.2021.756550
- Horgusluoglu E, Neff R, Song WM, et al. Integrative metabolomics-genomics approach reveals key metabolic pathways and regulators of Alzheimer’s disease. Alzheimers Dement. 2022;18(6):1260-1278. doi: 10.1002/alz.12468
- Clark NR, Hu KS, Feldmann AS, et al. The characteristic direction: A geometrical approach to identify differentially expressed genes. BMC Bioinformatics. 2014;15:79. doi: 10.1186/1471-2105-15-79
- Broberg P. Statistical methods for ranking differentially expressed genes. Genome Biol. 2003;4(6):R41. doi: 10.1186/gb-2003-4-6-r41
- Chen S, Chai X, Wu X. Bioinformatical analysis of the key differentially expressed genes and associations with immune cell infiltration in development of endometriosis. BMC Genom Data. 2022;23(1):20. doi: 10.1186/s12863-022-01036-y
- Boyle EI, Weng S, Gollub J, et al. GO: TermFinder—open source software for accessing Gene Ontology information and finding significantly enriched Gene Ontology terms associated with a list of genes. Bioinformatics. 2004;20(18):3710-3715. doi: 10.1093/bioinformatics/bth456
- Von Mering C, Huynen M, Jaeggi D, Schmidt S, Bork P, Snel, B. STRING: A database of predicted functional associations between proteins. Nucleic Acids Res. 2003;31(1):258-261. doi: 10.1093/nar/gkg034
- Szklarczyk D, Kirsch R, Koutrouli M, et al. The STRING database in 2023: Protein-protein association networks and functional enrichment analyses for any sequenced genome of interest. Nucleic Acids Res. 2023;51(D1):D638-D646. doi: 10.1093/nar/gkac1000
- Yan T, Ding F, Zhao Y. Integrated identification of key genes and pathways in Alzheimer’s disease via comprehensive bioinformatical analyses. Hereditas. 2019;156:25. doi: 10.1186/s41065-019-0101-0
- Fiscon G, Sibilio P, Funari A, Conte F, Paci P. Identification of potential repurposable drugs in Alzheimer’s disease exploiting a bioinformatics analysis. J Pers Med. 2022;12(10):1731. doi: 10.3390/jpm12101731
- Liu W, Chen S, Rao X, Yang Y, Chen X, Yu L. The inflammatory gene PYCARD of the entorhinal cortex as an early diagnostic target for Alzheimer’s disease. Biomedicines. 2023;11(1):194. doi: 10.3390/biomedicines11010194
- Shi L, Jones WD, Jensen RV, et al. The balance of reproducibility, sensitivity, and specificity of lists of differentially expressed genes in microarray studies. BMC Bioinformatics. 2008;9 Suppl 9:S10. doi: 10.1186/1471-2105-9-S9-S10
- Wei B, Wang L, Du C, Hu G, et al. Identification of differentially expressed genes regulated by transcription factors in glioblastomas by bioinformatics analysis. Mol Med Rep. 2015;11(4):2548-2554. doi: 10.3892/mmr.2014.3094
- Yang X, Zhu S, Li L, et al. Identification of differentially expressed genes and signaling pathways in ovarian cancer by integrated bioinformatics analysis. Onco Targets Ther. 2018;11:1457-1474. doi: 10.2147/OTT.S152238
- Fowler DM, Araya CL, Gerard W, Fields S. Enrich: Software for analysis of protein function by enrichment and depletion of variants. Bioinformatics. 2011;27(24):3430-3431. doi: 10.1093/bioinformatics/btr577
- Ding Z, Wei Q, Kihara D. Computing and visualizing gene function similarity and coherence with NaviGO. Methods Mol Biol. 2018;1807:113-130. doi: 10.1007/978-1-4939-8561-6_9
- Chin CH, Chen SH, Wu HH, Ho CW, Ko MT, Lin CY. cytoHubba: Identifying hub objects and sub-networks from complex interactome. BMC Syst Biol. 2014;8 Suppl 4:S11. doi: 10.1186/1752-0509-8-S4-S11
- Chang L, Zhou G, Soufan O, Xia J. miRNet 2.0: Network-based visual analytics for miRNA functional analysis and systems biology. Nucleic Acids Res. 2020;48(W1):W244-W251. doi: 10.1093/nar/gkaa467
- Zhang J, Lou W. A Key mRNA-miRNA-lncRNA competing endogenous RNA triple sub-network linked to diagnosis and prognosis of hepatocellular carcinoma. Front Oncol. 2020;10:340. doi: 10.3389/fonc.2020.00340
- Feng X, Bai Z, Wang J, et al. Robust gene dysregulation in Alzheimer’s disease brains. J Alzheimers Dis., 2014;41(2):587-597. doi: 10.3233/JAD-140147
- Kakati T, Bhattacharyya DK, Barah P, Kalita JK. Comparison of methods for differential co-expression analysis for disease biomarker prediction. Comput Biol Med. 2019;113:103380. doi: 10.1016/j.compbiomed.2019.103380
- Asgari N, Akbari MT, Zare S, Babamohammadi G. Positive association of apolipoprotein E4 polymorphism with recurrent pregnancy loss in Iranian patients. J Assist Reprod Genet. 2013;30(2):265-268. doi: 10.1007/s10815-012-9897-5
- Cha DJ, Mengel D, Mustapic M, et al. miR-212 and miR-132 are downregulated in neurally derived plasma exosomes of Alzheimer’s patients. Front Neurosci. 2019;13:1208. doi: 10.3389/fnins.2019.01208
- Hernández-Ortega K, Garcia-Esparcia P, Gil L, Lucas JJ, Ferrer I. Altered machinery of protein synthesis in Alzheimer’s: From the nucleolus to the ribosome. Brain Pathol. 2016;26(5):593-605. doi: 10.1111/bpa.12335
- Gui H, Gong Q, Jiang J, Liu M, Li H. Identification of the Hub genes in Alzheimer’s disease. Comput Math Methods Med. 2021;2021:6329041. doi: 10.1155/2021/6329041
- Feng L, Wang G, Song Q, et al. Proteomics revealed an association between ribosome-associated proteins and amyloid beta deposition in Alzheimer’s disease. Metab Brain Dis. 2024;39(2):263-282. doi: 10.1007/s11011-023-01330-3
- Wang W, Nag S, Zhang X, et al. Ribosomal proteins and human diseases: Pathogenesis, molecular mechanisms, and therapeutic implications. Med Res Rev. 2015;35(2):225-285. doi: 10.1002/med.21327
- Wilson DN, Cate JH. The structure and function of the eukaryotic ribosome. Cold Spring Harb Perspect Biol. 2012;4(5):a011536. doi: 10.1101/cshperspect.a011536
- Pelekoudas-Oikonomou F, Zachos G, Papaioannou M, et al. Blockchain-based security mechanisms for IoMT edge networks in IoMT-based healthcare monitoring systems. Sensors (Basel). 2022;22(7):2449. doi: 10.3390/s22072449
- Ito S, Yagi R, Ogata S, et al. Proteomic alterations in the brain and blood-brain barrier during brain Aβ accumulation in an APP knock-in mouse model of Alzheimer’s disease. Fluids Barriers CNS. 2023;20(1):66. doi: 10.1186/s12987-023-00466-9
- Suzuki M, Tezuka K, Handa T, et al. Upregulation of ribosome complexes at the blood-brain barrier in Alzheimer’s disease patients. J Cereb Blood Flow Metab. 2022;42(11):2134-2150. doi: 10.1177/0271678X221111602
- Luo S, Tamada A, Saikawa Y, Wang Y, Yu Q, Hisatsune T. P2Y1R silencing in astrocytes protected neuroinflammation and cognitive decline in a mouse model of Alzheimer’s disease. Aging Dis. 2024;15(4):1969-1988. doi: 10.14336/AD.2023.1006
- Sowell RA, Owen JB, Butterfield DA. Proteomics in animal models of Alzheimer’s and Parkinson’s diseases. Ageing Res Rev. 2009;8(1):1-17. doi: 10.1016/j.arr.2008.07.003
- Zhou X, Huang K, Wang Y, et al. Evaluation of therapeutic effects of tetramethylpyrazine nitrone in Alzheimer’s disease mouse model and proteomics analysis. Front Pharmacol. 2023;14:1082602. doi: 10.3389/fphar.2023.1082602