Artificial Neural Network Model to Forecast the Concentration of Pollutants Over Delhi: Skill Assessment of Learning Rules
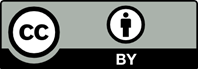
Air pollution has been reported to persuade climate as well as health significantly and is a matter of concern. The scientific endeavour should thus, be to develop forecast or warning system to predict the concentration of pollutants with considerable accuracy so that the calamities associated with pollution can be minimized, if not eradicated. The purpose of the study is to develop an Artificial Neural Network (ANN) model with different learning rules to predict the concentration of pollutants over Delhi (28° 38¢N, 77° 12¢E), India for the year 2009. Two types of learning rules are implemented in this study to forecast the concentration of different pollutants. The result reveals that the forecast accuracy of a particular pollutant depends on the type of the learning rule of the ANN model. The result of the study further reveals that the non-linear perceptron is better for forecasting the concentration of sulphur dioxide (SO2), carbon monoxide (CO), suspended particulate matter (SPM) and ozone (O3) whereas delta learning is better for forecasting nitrogen dioxide (NO2). The percentage errors in forecast with different learning rules of the ANN model are compared for all the pollutants. The result shows that the concentration of SO2 can be predicted over Delhi with maximum accuracy using nonlinear perceptron.
Aneja, V.P., Agarwal, A., Roelle, A.P., Phillips, S.B., Tong, Q., Watkinson, N. and Richard Yablonsky (2001). Measurements and analysis of criteria pollutants in New Delhi, India. Environment International, 27: 35–42.
Annand, W.J.D. and A.M. Hudson (1981). Meteorological effects on smoke and sulphur dioxide concentrations in the Manchester area. Atmos. Environ., 115: 799–806.
Chaudhuri, S. (2006). Artificial Neural Network Model to forecast maximum wind speed associated with severe thunderstorms. Vatabaran, AFAC J. of Meteorol., 30(1):14–19.
Chaudhuri, S. (2007). Artificial Neural Network Model to Forecast Ozone Concentration over Kolkata. Sci & Cult.,73: 9–10, 303–307.
Comrie, A.C. (1997). Comparing neural network and regression models for RSPM forecasting. Journal of Air and Waste Management Association, 47: 653–663.
Díaz-Robles, L.A., Ortega, J.C., Fu, J.S., Reed, G.D., Chow, J.C., Watson. J.G. and J.A. Moncada Herrera (2008). A hybrid ARIMA and artificial neural networks model to forecast particulate matter in urban areas: The case of Temuco, Chile, 42(35): 8331–8340.
Elsom, D.M. and T.J. Chandler (1978). Meteorological controls upon ground level smoke and sulphur dioxide in two urban areas of the United Kingdom. Atmos. Environ., 12: 1543–1554.
Hagan, Martin T., Demuth Howard, B. and H. Beale Mark (1996). ISBN: 0-9717321-0-8. Neural Network Design, 25: 2–6.
Hornik, K. (1991). Approximation capabilities of multi-layer neural networks. Neural Networks, 4: 251–257.
Jacobson, M.Z. (1998). Fundamentals of Atmospheric Modelling, Cambridge University Press, NewYork, p. 656.
Jain, S.L., Arya, B.C., Kumar Arun, Ghude, S.D. and P.S. Kulkarni (2005). Observational study of surface ozone at
New Delhi, India. International Journal of Remote Sensing,26: 3515–3524.
Kampa, M. and Castanas (2008). Human health effects of air pollution. Environ. Pollution, 151: 362–367.
Pal, S.K. and S. Mitra (1999). Neuro–Fuzzy Pattern Recognition. Wiley Interscience Publication, New York.
Patterson, D.W. (1996). Artificial Neural Networks: Theory and Applications. Prentice Hall, Englewoods Cliffs.
Perez, P., Trier, A. and J. Reyes (2000). Prediction of PM2.5 concentrations several hours in advance using neural networks in Santiago, Chile. Atmospheric Environment, 34: 1189–1196.
Rajaskaran, S. and G.A. Vijayalakshmi Pai (2003). Neural Networks, Fuzzy Logic and Genetic algorithms. In: Synthesis and Applications, Prentice Hall, New Delhi.
Sang Hyun Sohn, Sea Cheon Oh and Yeong-Koo Yeo (1999). Prediction of air pollutants by using an artificial neural network. Dept. of Chem. Eng., Hanyang University, Seoul, Korea, 133–791.
Schwartz, S.E. (1988). Are global cloud albedo and climate controlled by marine phytoplankton? Nature, 336: 441–445.
Wigley, T.M.L. (1989). Possible climate change due to SO2- derived cloud condensation nuclei. Nature, 339: 365–367.