Comparisons of Different Cluster Analysis Methods with Application to Mizoram Rainfall Data
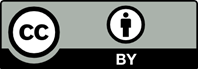
Analysing rainfall patterns in Mizoram from 1998 to 2017 reveals diverse trends. The highest average rainfall occurred in 2004, reaching 292.8 mm, while 2014 marked the lowest at 151.77 mm. Siaha District experienced the highest average rainfall (3020.2 mm), while Champhai District had the lowest (1663 mm). In 2017, the Kendall method showed correlations between temperature and relative humidity, rainfall and relative humidity, but not between rainfall and temperature. Cluster analysis, a technique partitioning datasets into cohesive groups, was applied to Mizoram’s district-wise rainfall data using single, complete, and average linkage methods. The Single Linkage Method formed one large cluster with under 26% similarity and the shortest distances between data points. The complete linkage method divided districts into two clusters with under 26% similarity and maximal inter-cluster distance. The Average Linkage Method merged all districts into one cluster with under 26% similarity and minimised inter-cluster distances. Comparing the techniques, Single and Complete Linkage Methods proved most effective for Mizoram’s district-wise rainfall data. With only eight districts, forming additional clusters remained limited. This analysis highlights the significance of rainfall patterns in agricultural ecosystems and the utility of statistical methodologies like cluster analysis in understanding long-term trends.
Alam, M.S. and S. Paul (2020). A comparative analysis of clustering algorithms to identify the homogeneous rainfall gauge stations of Bangladesh. J Appl Stat, 47: 1460-1481. https://doi.org/10.1080/02664763.2019.1675606
Gadgil, S. and R. Narayana Iyengar (1980). Cluster analysis of rainfall stations of the Indian peninsula. Quarterly Journal of the Royal Meteorological Society, 106: 873- 886. https://doi.org/10.1002/qj.49710645016
Gupta, S.C. and V.K. Kapoor (2002). Fundamentals of mathematical statistics : A modern approach. Sultan Chand.
Lallianthanga, R.K., n.d. Climate Profile of Mizoram.
Lin, F.R., Wu, N.J. and T.K. Tsay (2017). Applications of cluster analysis and pattern recognition for typhoon hourly rainfall forecast. Advances in Meteorology, 2017(2): 1-17. https://doi.org/10.1155/2017/5019646
Machiwal, D., Kumar, S., Meena, H.M., Santra, P., Singh, R.K. and D.V. Singh (2019). Clustering of rainfall stations and distinguishing influential factors using PCA and HCA techniques over the western dry region of India. Meteorological Applications 26: 300-311. https://doi. org/10.1002/met.1763
Meteorological Data of Mizoram 2017. P R E F A C E, n.d. Directorate of Economics & Statistics, Planning & Programme Implementation. Department Government of Mizoram
Michaelides, S.C., Pattichis, C.S. and G. Kleovoulou (2001). Classification of rainfall variability by using artificial neural networks. International Journal of Climatology, 21: 1401-1414. https://doi.org/10.1002/joc.702
Muñozmu˜muñoz-Díaz, D. and F.S. Rodrigo (2004). Spatiotemporal patterns of seasonal rainfall in Spain (1912- 2000) using cluster and principal component analysis: Comparison. Annales Geophysicae, 22(5): 1435-1448.
Nasseri, M. and B. Zahraie (2011). Application of simple clustering on space-time mapping of mean monthly rainfall pattern. International Journal of Climatology, 31: 732-741. https://doi.org/10.1002/joc.2109
Pansera, W. and B.M. Gomes (2005). Clustering rainfall stations aiming regional frequency analysis. Journal of Food Agriculture and Environment, 11(2): 877-885.
Santos, C.A.G., Neto, R.M.B., da Silva, R.M. and S.G.F. Costa (2019). Cluster analysis applied to spatiotemporal variability of monthly precipitation over Paraíba state using tropical rainfall measuring mission (TRMM) data. Remote Sens (Basel), 11(6): 637. https://doi.org/10.3390/ rs11060637
Teodoro, P.E., de Oliveira-Júnior, J.F., da Cunha, E.R., Correa, C.C.G., Torres, F.E., Bacani, V.M., Gois, G. and L.P. Ribeiro (2016). Cluster analysis applied to the spatial and temporal variability of monthly rainfall in Mato Grosso do Sul State, Brazil. Meteorology and Atmospheric Physics, 128: 197–209. https://doi.org/10.1007/s00703- 015-0408-y
Yashwant, S. and S.L. Sananse (2007). Comparisons of different methods of cluster analysis with application to rainfall data. International Journal of Innovative Research in Science, Engineering and Technology. 4(11): 10861- 10872. https://doi.org/10.15680/IJIRSET.2015.0411087