Optimal Reactive Power Dispatch by Success History Based Adaptive Differential Evolution Salp Swarm Algorithm
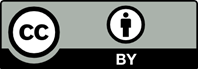
In this study, a novel hybrid algorithm success history-based adaptive differential evolution salp swarm algorithm (SHADE-SSA) is proposed to solve two different cases of IEEE 30 bus reactive power dispatch problems integrated with thermal generators, wind farms and solar photovoltaic plants. Real power loss minimization and voltage deviation minimization are considered as main objectives in the present work. The performance and robustness of the proposed hybrid SHADE-SSA algorithm are compared with the results of five different metaheuristic algorithms for the same test system and consider the same control variables and constraints. The results of the simulation of the proposed algorithm conform to the effective choice for the solution of optimal reactive power dispatch problems of power systems.
Afgan, N.H. and M.G. Carvalho (2002). Multicriteria assessment of new and renewable energy power plants. Energy, 27: 739-755.
Biswas, P.P., Suganthan, P.N. and G.A.J. Amaratunga (2017). Optimal power flow solutions incorporating stochastic wind and solar power. Energy Convers Manage., 148: 1194-1207.
Ahmad, M., Javaid, N., Niaz, I.A., Almogren, A. and A. Radwan (2021). A bio-inspired heuristic algorithm for solving optimal power flow problem in hybrid power system. IEEE Access, 9: 159809-159826.
Kumar, N., Kumar, R., Mohapatra, P. and R. Kumar (2020). Modified competitive swarm technique for solving the economic load dispatch problem. Journal of Information and Optimization Sciences, 41: 173-184.
Kadir, A. and Y. Volkan (2016). Differential search algorithm for solving multiobjective optimal power flow problem. Int J Electr Power Energy Syst, 79: 1-10.
Kumar, R., Rajan, A., Talukdar, F., Dey, N., Santhi, V. and V.E. Balas (2017). Optimization of 5.5-GHz CMOS LNA parameters using firefly algorithm. Neural Comput. Appl, 28(12): 3765-3779.
Kumar, R., Talukdar, F., Dey, N. and V. Balas (2016). Quality factor optimization of spiral inductor using firefly algorithm and its application in amplifier. InternationalJournal of Advanced Intelligence Paradigms, 11(3-4): 299-314.
Mohamed, A.A.A., Mohamed, Y.S., El-Gaafary, A.A. and A.M. Hemeida (2017). Optimal power flow using moth swarm algorithm. Electric Power Sys Res, 142: 190-206.
Mota-Palomino, R. and V.H. Quintana (1986). Sparse Reactive Power Scheduling by a Penalty Function—Linear Programming Technique. IEEE Trans, Power Syst. pp. 31-39.
Panda, A., Mishra, U., Tseng, M.L. and M. Ali (2020). Hybrid power systems with emission minimization: Multi- objective optimal operation. J. Cleaner Prod, 268: 121418.
Panda, A. and M. Tripathy (2015). Security constrained optimal power flow solution of wind-thermal generation system using modified bacteria foraging algorithm. Energy, 93: 816-827.
Tanabe, R. and A. Fukunaga (2013). Success-History Based Parameter Adaptation for Differential Evolution. In: IEEE CEC. pp. 71-78.