Optimal Power Flow Through Hybrid Power System Using Metaheuristic Hybrid Algorithm
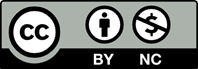
The growing population and modernisation in life styles of people increase the demand of electrical power. This has led to pressure on conventional thermal power plants to increase the production of electrical energy by using more and more fossil fuels like coal, petrol, diesel and natural gases, which enhance the emission of greenhouse gases causing environmental pollution. Hence, renewable sources of energy attract the attention of researchers as these can reduce the cost of production, and carbon emissions and has high efficiency. In this study, an IEEE 30- bus hybrid power test system consisting of thermal generators, wind generators and solar photo voltaic have been considered to achieve economically, environmentally as well as physically stable systems. The adopted hybrid power system follows a highly non-linear and complex nature, hence a novel hybrid algorithm named SHADE-SSA is framed to find optimal solutions economically and environmentally with stable voltage deviation and low power loss. The performance of the SHADE-SSA algorithm is compared with the SHADE-SF algorithm and SSA, to confirm the superiority in solving complex, non-linear hybrid power system problems
Abido, M. (2002). Optimal power flow using particle swarm optimization. Int. J. Electr. Power Energy Syst., 24: 563- 571.
Bhattacharya, A. and P.K. Chattopadhyay (2010). Application of biogeography-based optimization for solving multiobjective economic emission load dispatch problems. Electr. Power Compon. Syst., 38: 340-365.
Biswas, P.P., Suganthan, P.N. and G.A.J. Amaratunga (2017). Optimal power flow solutions incorporating stochastic wind and solar power. Energy Convers. Manage., 148: 1194-1207.
Dashtdar, M. and M. Najafi (2019). Calculating the locational marginal price and solving optimal power flow problem based on congestion management using GA-GSF algorithm. Electr. Eng., 102: 1549-1566.
Duman, S., Li, J. and L. Wu (2019). Optimal power flow with stochastic wind power and FACTS devices: A modified hybrid PSOGSA with chaotic maps approach. Neural Comput. & Applic., 32: 8463-8492.
Khunkitti, S., Siritaratiwat, A., Premrudeepreechacharn, S., Chatthaworn, R. and N.R. Watson (2018). A hybrid DAPSO optimization algorithm for multiobjective optimal power flow problems. Energies, 11: 2270
Kumar, R., Rajan, A., Talukdar, F.A., Dey, N., Santhi, V. and V.E. Balas (2017). Optimization of 5.5-GHz CMOS LNA parameters using firefly algorithm. Neural Comput. Appl, 28(12): 3765-3779.
Kumar, R., Talukdar, F., Dey, N. and V. Balas (2016). Quality factor optimization of spiral inductor using firefly algorithm and its application in amplifier. International Journal of Advanced Intelligence Paradigms, 11: 299- 314.
Kumar, N., Kumar, R., Mohapatra, P. and R. Kumar (2020). Modified competitive swarm technique for solving the economic load dispatch problem. Journal of Information and Optimization Sciences, 41: 173-184.
Mirjalili, S., Gandomi, A.H., Mirjalili, S.Z., Saremi, S., Faris, H. and S.M. Mirjalili (2017). Salp swarm algorithm: A bio-inspired optimizer for engineering design problems. Advances in Engg. Software, 114: 163-191.
Muhammad, R., Hanif, A., Hussain, S.J., Memon, M.I., Ali, M.U and A. Zafar (2021). An optimization-based strategy for solving optimal power flow problems in a power system integrated with stochastic solar and wind power energy. Applied Sciences, 11: 6883.
Panda A., Mishra U., Tseng M.L. and M. Ali (2020). Hybrid power systems with emission minimization: Multiobjective optimal operation. J. Cleaner Prod., 268: 121418 Reddy, S.S. (2018). Optimal power flow using hybrid differential evolution and harmony search algorithm. Int. J. Mach. Learn. Cybern., 10: 1077-1091.
Tanabe, R. and A. Fukunaga (2013). Success-history based parameter adaptation for differential evolution. IEEE CEC, pp. 71-78.