Artificial intelligence and lymphedema: State of the art
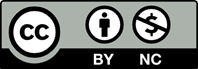
Background: Lymphedema practice is facing many challenges. Some of these challenges include eradication of tropical lymphedema, preclinical diagnosis of cancer-related lymphedema, and delivery of appropriate individualized care. The past two decades have witnessed an increasing implementation of artificial intelligence in healthcare services. The nature of the challenges facing the lymphedema practice is suitable for artificial intelligence applications.
Aim: To explore the current artificial intelligence applications in lymphedema prevention, diagnosis, and management and investigate the potential future applications.
Methods and results: Four databases were searched: PubMed, Scopus, Web of Science, and EMBASE. We used the Preferred Reporting Items for Systematic Reviews and Meta-Analysis (PRISMA) as our basis of organization. Our analysis showed that several domains of artificial intelligence, including machine learning, fuzzy models, deep learning, and robotics, were successfully implemented in lymphedema practice. Machine learning can guide the eradication campaigns of tropical lymphedema by estimating disease prevalence and mapping the risk areas. Robotic-assisted surgery for gynecological cancer was associated with a lower risk for lower limb lymphedema. Several feasible models were described for the early detection and diagnosis of lymphedema. The proposed models are more accurate, sensitive, and specific than current methods in practice. Machine learning was also used to guide and monitor patients during the rehabilitation exercises.
Conclusion: Artificial intelligence offers a variety of solutions to the most challenging problems in lymphedema practice. Further implementation into the practice can revolutionize many aspects of lymphedema prevention, diagnosis, and management.
Relevance to patients: Lymphedema is a chronic debilitating disease that is affecting millions of patients. Developing new modalities for prevention, early diagnosis, and treatment is critical to improve the outcomes. Artificial intelligence offers a variety of solutions for some of the complexities of lymphedema management. In this systematic review, we summarize and discuss the latest artificial intelligence advances in lymphedema practice.
[1] Warren AG, Brorson H, Borud LJ, Slavin SA. Lymphedema: A Comprehensive Review. Ann Plast Surg 2007;59:464-72.
[2] Wheeler ES, Chan V, Wassman R, Rimoin DL, Lesavoy MA. Familial Lymphedema Praecox: Meige’s Disease. Plast Reconstr Surg 1981;67:362-4.
[3] World Health Organization. Lymphatic Filariasis: Key Facts. Geneva: World Health Organization; 2021.
[4] Petrek JA, Senie RT, Peters M, Rosen PP. Lymphedema in a Cohort of Breast Carcinoma Survivors 20 Years After Diagnosis. Cancer 2001;92:1368-77.
[5] The Diagnosis and Treatment of Peripheral Lymphedema. Consensus Document of the International Society of Lymphology. Lymphology 2003;36:84-91.
[6] Oremus M, Dayes I, Walker K, Raina P. Systematic Review: Conservative Treatments for Secondary Lymphedema. BMC Cancer 2012;12:6.
[7] Deo RC. Machine Learning in Medicine. Circulation 2015;132:1920-30.
[8] Noorbakhsh-Sabet N, Zand R, Zhang Y, Abedi V. Artificial Intelligence Transforms the Future of Health Care. Am J Med 2019;132:795-801.
[9] Zanagnolo V, Minig L, Rollo D, Tomaselli T, Aletti G, Bocciolone L, et al. Clinical and Oncologic Outcomes of Robotic Versus Abdominal Radical Hysterectomy for Women with Cervical Cancer: Experience at a Referral Cancer Center. Int J Gynecol Cancer 2016;26:568-74.
[10] Krill LS, Bristow RE. Robotic Surgery: Gynecologic Oncology. Cancer J 2013;19:167-76.
[11] Moreira R, Magalhães A, Oliveira HP. A Kinect-based System to Assess Lymphedema Impairments in Breast Cancer Patients. In: Lecture Notes in Computer Science (Including Subseries Lecture Notes in Artificial Intelligence and Lecture Notes in Bioinformatics). Berlin, Germany: Springer; 2015. p. 228-36.
[12] Arnold BF, van der Laan MJ, Hubbard AE, Steel C, Kubofcik J, Hamlin KL, et al. Measuring Changes in Transmission of Neglected Tropical Diseases, Malaria, and Enteric Pathogens from Quantitative Antibody Levels. PLoS Negl Trop Dis 2017;11:e0005616.
[13] Chiang AT, Chen Q, Wang Y, Fu MR. Kinect-based in-home Exercise System for Lymphatic Health and Lymphedema Intervention. IEEE J Transl Eng Health Med 2018;6:4100313.
[14] Deribe K, Cano J, Njouendou AJ, Eyong ME, Beng AA, Giorgi E, et al. Predicted Distribution and Burden of Podoconiosis in Cameroon. BMJ Global Health 2018;3:730.
[15] Eneanya OA, Cano J, Dorigatti I, Anagbogu I, Okoronkwo C, Garske T, et al. Environmental Suitability for Lymphatic Filariasis in Nigeria. Parasit Vectors 2018;11:513.
[16] Fu MR, Wang Y, Li C, Qiu Z, Axelrod D, Guth AA, et al. Machine Learning for Detection of Lymphedema among Breast Cancer Survivors. Mhealth 2018;4:17.
[17] Eneanya OA, Fronterre C, Anagbogu I, Okoronkwo C, Garske T, Cano J, et al. Mapping the Baseline Prevalence of Lymphatic Filariasis Across Nigeria. Parasit Vectors 2019;12:440.
[18] Kistenev YV, Borisov AV, Nikolaev VV, Vrazhnov DA, Sandykova EA, Kurochkina OS. Application of Multiphoton Imaging and Machine Learning to Lymphedema Tissue Analysis. Biomed Optics Exp 2019;10:3353-68.
[19] Mayfield HJ, Sturrock H, Arnold BF, Andrade-Pacheco R, Kearns T, Graves P, et al. Supporting Elimination of Lymphatic Filariasis in Samoa by Predicting Locations of Residual Infection using Machine Learning and Geostatistics. Sci Rep 2020;10:20570.
[20] Chausiaux O, Williams G, Nieznański M, Bagdu A, Downer P, Keyser M, et al. Evaluation of the Accuracy of a Video and Ai Solution to Measure Lower Leg and Foot Volume. Med Dev Evid Res 2021;14:105-18.
[21] Kwarteng EV, Andam-Akorful SA, Kwarteng A, Asare DB, Quaye-Ballard JA, Osei FB, Duker AA. Spatial Variation in Lymphatic Filariasis Risk Factors of Hotspot Zones in Ghana. BMC Public Health 2021;21:230.
[22] Notash AY, Bayat P, Haghighat S, Notash AY. Evolutionary Ensemble Feature Selection Learning for Image-based Assessment of Lymphedema Arm Volume. Concurrency and Computation: Practice and Experience. United States: John Wiley & Sons Ltd.; 2021.
[23] Agarwal R, Rajanbabu A, Goel G, Unnikrishnan UG. A Comparison of the Clinical Outcomes in Uterine Cancer Surgery After the Introduction of Robotic-assisted Surgery. J Obstet Gynaecol India 2019;69:284-91.
[24] Vicentini P, Del Perez MC, Araujo E. Fuzzy Lymphedema Assessment Based on Clinical and functional Criteria: IEEE International Conference on Fuzzy Systems, United States: IEEE; 2011. p. 2708-13.
[25] Lourens GB, Ferrell DK. Lymphatic Filariasis. Nurs Clin North Am 2019;54:181-92.
[26] Grada AA, Phillips TJ. Lymphedema: Pathophysiology and Clinical Manifestations. J Am Acad Dermatol 2017;77:1009-20.
[27] Centers for Disease Control and Prevention. Lymphatic Filariasis: Epidemiology and Risk Factors. United States: Centers for Disease Control and Prevention; 2021.
[28] Huang X, Deng X, Kou J, Liu X, Wang H, Cheng P, et al. Elimination of Lymphatic Filariasis in Shandong Province, China, 1957-2015. Vector Borne Zoonotic Dis 2020;20:875-81.
[29] Davis EL, Reimer LJ, Pellis L, Hollingsworth TD. Evaluating the Evidence for Lymphatic Filariasis Elimination. Trends Parasitol 2019;35:860-9.
[30] Polikar R. Ensemble Based Systems in Decision Making. IEEE Circ Syst Mag 2006;6:21-44.
[31] Korevaar DA, Visser BJ. Podoconiosis, a Neglected Tropical Disease. Neth J Med 2012;70:210-4.
[32] Price EW, Bailey D. Environmental Factors in the Etiology of Endemic Elephantiasis of the Lower Legs in Tropical Africa. Trop Geogr Med 1984;36:1-5.
[33] Deribe K, Cano J, Trueba ML, Newport MJ, Davey G. Global Epidemiology of Podoconiosis: A Systematic Review. PLoS Negl Trop Dis 2018;12:e0006324.
[34] Lawenda BD, Mondry TE, Johnstone PA. Lymphedema: A Primer on the Identification and Management of a Chronic Condition in Oncologic Treatment. CA Cancer J Clin 2009;59:8-24.
[35] Ruocco V, Schwartz RA, Ruocco E. Lymphedema: An Immunologically Vulnerable Site for Development of Neoplasms. J Am Acad Dermatol 2002;47:124-7.
[36] Shah C, Arthur DW, Wazer D, Khan A, Ridner S, Vicini F. The Impact of Early Detection and Intervention of Breast Cancer-related Lymphedema: A Systematic Review. Cancer Med 2016;5:1154-62.
[37] McLaughlin SA, Bagaria S, Gibson T, Arnold M, Diehl N, Crook J, et al. Trends in Risk Reduction Practices for the Prevention of Lymphedema in the First 12 Months After Breast Cancer Surgery. J Am Coll Surg 2013;216:380-9.
[38] Ekeland AG, Bowes A, Flottorp S. Effectiveness of Telemedicine: A Systematic Review of Reviews. Int J Med Inform 2010;79:736-71.