Machine learning applications for quality improvement in laser powder bed fusion: A state-of-the-art review
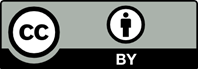
As one of the most popular additive manufacturing methods, laser powder bed fusion (L-PBF) builds 3D components with complex geometries layer by layer using alloy powders. This technique has found widespread adoption in various industrial applications, including biomedical and aerospace fields. However, L-PBF encounters challenges related to poor process repeatability and inconsistency in fabricated part quality, which hinder its broader adoption. Various quality improvement methods have been proposed to address these challenges and achieve high-quality, reliable parts. Given the abundance of parameters and the intricate phenomena that occur during the process, machine learning (ML) methods play a critical role in enhancing the quality of L-PBF, providing an optimum solution for improving the quality of manufactured parts. This review paper begins with a comprehensive and straightforward introduction to ML, focusing primarily on different learning approaches. Subsequently, the paper explores different ML methods applied to parameter optimization and in situ monitoring, both contributing to enhanced quality control. In parameter optimization, ML is employed to extract relationships between input parameters and key factors such as melt pool characteristics, porosity, and mechanical properties. Shifting the focus to in situ monitoring, the paper introduces the application of ML in analyzing various sensor data generated throughout the L-PBF process. Accomplished tasks include segmentation, regression, and classification of quality measurement. In summary, this review underscores the critical role of machine learning in addressing challenges associated with L-PBF, providing an optimal solution for quality enhancement.
- Bhavar V, Kattire P, Patil V, Khot S, Gujar K, Singh R. A review on powder bed fusion technology of metal additive manufacturing. In: Additive Manufacturing Handbook. United States, CRC Press 2017, p251-253. doi: 10.1201/9781315119106-15
- Buchanan C, Gardner L. Metal 3D Printing in construction: A review of methods, research, applications, opportunities and challenges. Eng Struct. 2019;180:332-348. doi: 10.1016/j.engstruct.2018.11.045
- Sing SL. Perspectives on additive manufacturing enabled beta-titanium alloys for biomedical applications. Int J Bioprint. 2022;8(1):478. doi: 10.18063/ijb.v8i1.478
- Frazier WE. Metal additive manufacturing: A review. J Mater Eng Perform. 2014;23:1917-1928. doi: 10.1007/s11665-014-0958-z
- Guo N, Leu MC. Additive manufacturing: Technology, applications and research needs. Front Mech Eng. 2013;8:215-243. doi: 10.1007/s11465-013-0248-8
- Gu D, Shen Y. Effects of dispersion technique and component ratio on densification and microstructure of multi-component cu-based metal powder in direct laser sintering. J Mater Process Technol. 2007;182(1-3):564-573. doi: 10.1016/j.jmatprotec.2006.09.026
- Yap CY, Chua CK, Dong ZL, et al. Review of selective laser melting: Materials and applications. Appl Physics Rev. 2015;2(4):041101. doi: 10.1063/1.4935926
- Li R, Liu J, Shi Y, Du M, Xie Z. 316L stainless steel with gradient porosity fabricated by selective laser melting. J Mater Eng Perform. 2010;19(5):666-671. doi: 10.1007/s11665-009-9535-2.
- Gao W, Zhang Y, Ramanujan D, et al. The status, challenges, and future of additive manufacturing in engineering. Comput Aided Des. 2015;69:65-89. doi: 10.1016/j.cad.2015.04.001
- Pereira T, Kennedy JV, Potgieter J. A comparison of traditional manufacturing vs additive manufacturing, the best method for the job. Proc Manuf. 2019;30:11-18. doi: 10.1016/j.promfg.2019.02.003
- Fotovvati B, Balasubramanian M, Asadi E. Modeling and optimization approaches of laser-based powder-bed fusion process for Ti-6Al-4V alloy. Coatings. 2020;10(11):1104. doi: 10.3390/coatings10111104
- Khaimovich AI, Stepanenko IS, Smelov VG. Optimization of selective laser melting by evaluation method of multiple quality characteristics. IOP Conf Ser Mater Sci Eng. 2018;302(1):012067. doi: 10.1088/1757-899X/302/1/012067
- Wang C, Tan XP, Tor SB, Lim CS. Machine learning in additive manufacturing: State-of-the-art and perspectives. Addit Manuf. 2020;36:101538. doi: 10.1016/j.addma.2020.101538
- Janík S, Szabó P, Mĺkva M, Mareček-Kolibiský M. Effective data utilization in the context of industry 4.0 technology integration. Appl Sci. 2022;12(20):10517. doi: 10.3390/app122010517
- Jordan MI, Mitchell TM. Machine learning: Trends, perspectives, and prospects. Science. 2015;349(6245):255-260. doi: 10.1126/science.aaa8410
- Huang DJ, Li H. A machine learning guided investigation of quality repeatability in metal laser powder bed fusion additive manufacturing. Mater Des. 2021;203:109606. doi: 10.1016/j.matdes.2021.109606
- Goh GD, Sing SL, Yeong WY. A review on machine learning in 3D printing: Applications, potential, and challenges. Artif Intell Rev. 2021;54:63-94. doi: 10.1007/s10462-020-09876-9
- Scime L, Beuth J. Anomaly detection and classification in a laser powder bed additive manufacturing process using a trained computer vision algorithm. Addit Manuf. 2018;19:114-126. doi: 10.1016/j.addma.2017.11.009
- Sanchez S, Rengasamy D, Hyde CJ, Figueredo GP, Rothwell B. Machine learning to determine the main factors Affecting creep rates in laser powder bed fusion. J Intell Manufact. 2021;32:2353-2373. doi: 10.1007/s10845-021-01785-0
- Srinivas M, Sucharitha G, Matta A, Chatterjee P, editors. Machine Learning Algorithms and Applications. 1st ed. United States: Wiley; 2021. doi: 10.1002/9781119769262
- Van Engelen JE, Hoos HH. A survey on semi-supervised learning. Mach Learn. 2020;109(2):373-440. doi: 10.1007/s10994-019-05855-6
- Bradford E, Schweidtmann A, Lapkin A. Efficient multiobjective optimization employing Gaussian processes, spectral sampling, and a genetic algorithm. J Glob Optim. 2018;71:407-438. doi: 10.1007/s10898-018-0609-2
- Liashchynskyi P, Liashchynskyi P. Grid search, random search, genetic algorithm: A big comparison for NAS[J]. arXiv preprint arXiv:1912.06059; 2019. doi: 10.48550/arXiv.1912.06059
- Shi T, Sun J, Li J, Qian G, Hong Y. Machine learning based very-high-cycle fatigue life prediction of AlSi10Mg alloy fabricated by selective laser melting. Int J Fatigue. 2023;171:107585. doi: 10.1016/j.ijfatigue.2023.107585
- Bergstra J, Bengio Y. Random search for hyper-parameter optimization. J Mach Learn Res. 2012;13:281-305. doi: 10.5555/2188385.2188395
- Shahriari B, Swersky K, Wang Z, et al. Taking the human out of the loop: A review of Bayesian optimization. Proc IEEE. 2016;104(1):148-175. doi: 10.1109/JPROC.2015.2494218
- Rong-Ji W, Xin-Hua L, Qing-Ding W, Lingling W. Optimizing process parameters for selective laser sintering based on neural network and genetic algorithm. Int J Adv Manuf Technol. 2009;42:1035-1042. doi: 10.1007/s00170-008-1669-0
- Zouhri W, Dantan JY, Häfner B, et al. Optical process monitoring for laser-powder bed fusion (L-PBF). CIRP J Manufact Sci Technol. 2020;31:607-617. doi: 10.1016/j.cirpj.2020.09.001
- Wang C, Tan X, Liu E, Tor SB. Process parameter optimization and mechanical properties for additively manufactured stainless steel 316L parts by selective electron beam melting. Mater Des. 2018;147:157-166. doi: 10.1016/j.matdes.2018.02.059
- Chadwick AF, Voorhees PW. The effects of melt pool geometry and scan strategy on microstructure development during additive manufacturing. IOP Conf Ser Mater Sci Eng. 2023;1274:012010. doi: 10.1088/1757-899X/1274/1/012010
- Liu Y, Sing SL. Review on the use of machine learning techniques to optimize the processing of copper alloys in additive manufacturing. In: Asian Society for Precision Engineering and Nanotechnology (ASPEN 2022). Singapore: Research Publishing Services; 2022. p. 107-109. doi: 10.3850/978-981-18-6021-8_OR-01-0297.html
- Sabuj MR, Afshari SS, Liang X. Selective LASER melting part quality prediction and energy consumption optimization. Meas Sci Technol. 2023;34(7):075902. doi: 10.1088/1361-6501/acc5a4
- Uy M, Telford JK. Optimization by Design of Experiment Techniques. Big Sky, MT, USA, IEEE Aerospace Conference 2009, p1-10. doi: 10.1109/AERO.2009.4839625
- Goh GD, Huang X, Huang S, Thong JLJ, Seah JJ, Yeong WY. Data imputation strategies for process optimization of laser powder bed fusion of Ti6Al4V using machine learning. MSAM. 2023;2(1):50. doi: 10.36922/msam.50
- Wang C, Tan XP, Tor SB, Lim CS. Machine learning in additive manufacturing: State-of-the-art and perspectives. Addit Manuf. 2020;36:101538. doi: 10.1016/j.addma.2020.101538
- Forien J, Calta N, DePond P, Guss G, Roehling T, Matthews M. Detecting keyhole porosity and monitoring process signatures in additive manufacturing: An in situ pyrometry and ex situ X-ray radiography correlation. Addit Manuf. 2019;35. doi: 10.1016/j.addma.2020.101336
- Akbari P, Ogoke F, Kao NY, et al. MeltpoolNet: Melt pool characteristic prediction in metal additive manufacturing using machine learning. Addit Manuf. 2022;55:102817. doi: 10.1016/j.addma.2021.102817
- Lee S, Peng J, Shin D, Choi YS. Data analytics approach for melt-pool geometries in metal additive manufacturing. Sci Technol Adv Mater. 2019;20(1):972-978. doi: 10.1080/14686996.2019.1669114
- Yang Z, Lu Y, Yeung H, Krishnamurty S. From scan strategy to melt pool prediction: A Neighboring-effect modeling method. ASME J Comput Inf Sci Eng. 2020;20(5):051001. doi: 10.1115/1.4047926
- Saunders R, Rawlings A, Birnbaum A, et al. Additive manufacturing melt pool prediction and classification via multifidelity Gaussian process surrogates. Integr Mater Manuf Innov. 2022;11:497-515. doi: 10.1007/s40192-022-00204-3
- Bland S, Aboulkhair NT. Reducing porosity in additive manufacturing. Metal Powder Report. 2015;70(2):79-81. doi: 10.1016/j.mprp.2015.01.005
- Gao B, Zhao H, Peng L, Sun Z. A review of research progress in selective laser melting (SLM). Micromachines (Basel). 2023;14:57. doi: 10.3390/mi14010057
- Solberg K, Guan S, Razavi N, et al. Fatigue of additively manufactured 316L stainless steel: The influence of porosity and surface roughness. Fatigue Fract Eng Mater Struct. 2019;42:2043-52. doi: 10.1111/ffe.13004
- Tapia G, Elwany AH, Sang H. Prediction of porosity in metal-based additive manufacturing using spatial Gaussian process models. Addit. Manuf. 2016;12:282-290. doi: 10.1016/j.addma.2016.04.002
- Imani F, Gaikwad A, Montazeri M, Rao P, Yang H, Reutzel E. Process mapping and in-process monitoring of porosity in laser powder bed fusion using layerwise optical imaging. ASME J Manuf Sci Eng. 2018;140:101009. doi: 10.1115/1.4039501
- Hanzl H, Zetek M, Baka T, Kroupa T. The influence of processing parameters on the mechanical properties of SLM parts. Proc Eng. 2015;100:1405-1413. doi: 10.1016/j.proeng.2015.01.456
- Maitra V, Shi J. Predictability assessment of as-built hardness of Ti-6Al-4V alloy fabricated via laser powder bed fusion. Manufact Lett. 2023;35:785-796. doi: 10.1016/j.mfglet.2022.12.001
- Ravichander BB, Rahimzadeh A, Farhang B, Moghaddam NS, Amerinatanzi A, Mehrpouya M. A prediction model for additive manufacturing of inconel 718 superalloy. Appl Sci. 2021;11:8010. doi: 10.3390/app11188010
- Zhang T, Zhou X, Zhang P, et al. Hardness prediction of laser powder bed fusion product based on melt pool radiation intensity. Materials (Basel). 2022;15(13):4674. doi: 10.3390/ma15134674
- Fang Q, Tan Z, Li H, et al. In-situ capture of melt pool signature in selective laser melting using U-Net-based convolutional neural network. J Manuf Process. 2021;68:347-355. doi: 10.1016/j.jmapro.2021.05.052
- Taherkhani K, Eischer C, Toyserkani E. An unsupervised machine learning algorithm for in-situ defect-detection in laser powder-bed fusion. J Manuf Process. 2022;81:476-489. doi: 10.1016/j.jmapro.2022.06.074
- Huang DJ, Li H. A machine learning guided investigation of quality repeatability in metal laser powder bed fusion additive manufacturing. Mater Des. 2021;203:109606. doi: 10.1016/j.matdes.2021.109606
- Feng S, Chen Z, Bircher B, Ji Z, Nyborg L, Bigot S. Predicting laser powder bed fusion defects through in-process monitoring data and machine learning. Mater Des. 2022;222:111115. doi: 10.1016/j.matdes.2022.111115
- Paulson NH, Gould B, Wolff SJ, Stan M, Greco AC. Correlations between thermal history and keyhole porosity in laser powder bed fusion. Addit Manuf. 2020;34:101213. doi: 10.1016/j.addma.2020.101213
- Yuan B, Giera B, Guss G, Matthews I, Mcmains S. Semi- Supervised Convolutional Neural Networks for In-situ Video Monitoring of Selective Laser Melting. In: 2019 IEEE Winter Conference on Applications of Computer Vision (WACV). United States, IEEE 2019, p744-753. doi: 10.1109/WACV.2019.00084
- Li J, Cao L, Xu J, Wang S, Zhou Q. In situ porosity intelligent classification of selective laser melting based on coaxial monitoring and image processing. Measurement. 2022;187:110232. doi: 10.1016/j.measurement.2021.110232
- Li J, Zhou Q, Huang X, Li M, Cao L. In situ quality inspection with layer-wise visual images based on deep transfer learning during selective laser melting. J Intell Manuf. 2023;34(2):853-867. doi: 10.1007/s10845-021-01829-5
- Wang H, Li B, Xuan FZ. Acoustic emission for in situ process monitoring of selective laser melting additive manufacturing based on machine learning and improved variational modal decomposition. Int J Adv Manuf Technol. 2022;122(5-6):2277-2292. doi: 10.1007/s00170-022-10032-6
- Ansari MA, Crampton A, Garrard R, Cai B, Attallah M. A convolutional neural network (CNN) classification to identify the presence of pores in powder bed fusion images. Int J Adv Manuf Technol. 2022;120(7-8):5133-5150. doi: 10.1007/s00170-022-08995-7
- Drissi-Daoudi R, Pandiyan V, Logé R, Shevchik S, Masinelli G, Ghasemi-Tabasi H, et al. Differentiation of materials and laser powder bed fusion processing regimes from airborne acoustic emission combined with machine learning. Virtual Phys Prototyp. 2022;17(2):181-204. doi: 10.1080/17452759.2022.2028380
- Zhang MJ, Chen GY, Zhou Y, Li SC, Deng H. Observation of spatter formation mechanisms in high-power fiber laser welding of thick plate. Appl Surface Sci. 2013;280:868-875. doi: 10.1016/j.apsusc.2013.05.081
- Schweier M, Heins JF, Haubold MW, Zaeh MF. Spatter formation in laser welding with beam oscillation. Physics Proc. 2013;41:20-30. doi: 10.1016/j.phpro.2013.03.047
- Luo S, Ma X, Xu J, Li M, Cao L. Deep learning based monitoring of spatter behavior by the acoustic signal in selective laser melting. Sensors (Basel). 2021;21(21):7179. doi: 10.3390/s21217179
- Wang L, Chen X, Henkel D, Jin R. Pyramid ensemble convolutional neural network for virtual computed tomography image prediction in a selective laser melting process. J Manuf Sci Eng. 2021;143(12):121003. doi: 10.1115/1.4051077
- Yadav P, Rigo O, Arvieu C, Le Guen E, Lacoste E. In situ monitoring systems of the SLM process: On the need to develop machine learning models for data processing. Crystals. 2020;10(6):524. doi: 10.3390/cryst10060524
- Terry BS, Baucher B, Chaudhary A, Chakraborty S. Active monitoring of selective laser melting process by training an artificial neural net classifier on layer-by-layer surface laser profilometry data. In review. Int J Adv Manufact Technol. 2021. doi: 10.21203/rs.3.rs-893873/v1
- Snow Z, Diehl B, Reutzel EW, Nassar A. Toward in-situ flaw detection in laser powder bed fusion additive manufacturing through layerwise imagery and machine learning. J Manuf Syst. 2021;59:12-26. doi: 10.1016/j.jmsy.2021.01.008
- Pandiyan V, Drissi-Daoudi R, Shevchik S, et al. Semi-supervised monitoring of laser powder bed fusion process based on acoustic emissions. Virtual Phys Prototyp. 2021;16(4):481-497. doi: 10.1080/17452759.2021.1966166
- Okaro IA, Jayasinghe S, Sutcliffe C, Black K, Paoletti P, Green PL. Automatic fault detection for laser powder-bed fusion using semi-supervised machine learning. Addit Manuf. 2019;27:42-53. doi: 10.1016/j.addma.2019.01.006
- Mohammadi MG, Elbestawi M. Real time monitoring in L-PBF using a machine learning approach. Proc Manuf. 2020;51:725-731. doi: 10.1016/j.promfg.2020.10.102
- Li J, Cao L, Zhou Q, Liu H, Zhang X. Imbalanced quality monitoring of selective laser melting using acoustic and photodiode signals. J Manuf Process. 2023;105:14-26. doi: 10.1016/j.jmapro.2023.09.037
- Wasmer K, Kenel C, Saeidi F, Leinenbach C, Shevchik SA. In Situ and Real-Time Monitoring of Powder-Bed AM by Combining Acoustic Emission and Machine Learning. In: Lasers in Manufacturing Conference, 2017. doi: 10.1016/J.ADDMA.2017.11.012
- Wasmer K, Le-Quang T, Meylan B, Shevchik SA. In situ quality monitoring in AM using acoustic emission: A reinforcement learning approach. J Mater Eng Perform. 2019;28(2):666-672. doi: 10.1007/s11665-018-3690-2
- Colosimo BM, Huang Q, Dasgupta T, Tsung F. Opportunities and challenges of quality engineering for additive manufacturing. J Qual Technol. 2018;50(3):233-252. doi: 10.1080/00224065.2018.1487726
- Li BH, Hou BC, Yu WT, Lu XB, Yang CW. Applications of artificial intelligence in intelligent manufacturing: A review. Front Inf Technol Electron Eng. 2017;18(1):86-96. doi: 10.1631/FITEE.1601885
- Sing SL, Kuo CN, Shih CT, Ho CC, Chua CK. Perspectives of using machine learning in laser powder bed fusion for metal additive manufacturing. Virtual Phys Prototyp. 2021;16(3):372-386. doi: 10.1080/17452759.2021.1944229
- Cai Y, Xiong J, Chen H, Zhang G. A review of in-situ monitoring and process control system in metal-based laser additive manufacturing. J Manuf Syst. 2023;70:309-326. doi: 10.1016/j.jmsy.2023.07.018
- Cao Z, Liu Q, Liu Q, Yu X, Kruzic JJ, Li X. A machine learning method to quantitatively predict alpha phase morphology in additively manufactured Ti-6Al-4V. NPJ Comput Mater. 2023;9(1):1-15. doi: 10.1038/s41524-023-01152-y
- Nandhakumar R, Venkatesan K. A process parameters review on selective laser melting-based additive manufacturing of single and multi-material: Microstructure, physical properties, tribological, and surface roughness. Mater Today Commun. 2023;35:105538. doi: 10.1016/j.mtcomm.2023.105538