Correlation-Based Comparative Machine Learning Analysis for the Classification of Metastatic Breast Cancer Using Blood Profile
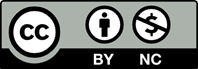
Objectives: Histopathological and mammography image-guided diagnosis is a common practice for the detection of cancer grade, which is often associated with poor survival outcomes in breast cancer patients. A deep learning (DL) based clinical decision support system was developed for histologic grading of breast cancer, which often requires invasive procedures or expensive imaging equipment. Our study aimed to establish a machine learning model based on simple blood profile data.
Methods: The dataset consists of blood profiles of 1250 breast cancer patients and 259 normal subjects. Statistical methods were used to select the relevant feature for machine learning model development. Selected features were fitted into various Machine Learning classifiers to predict breast cancer with highest accuracy.
Results: Correlation-based feature selection revealed that blood profile ratio counterparts were statistically significant (p<0.05) and were used for the classification of metastatic breast cancer patients as compared to normal subjects.
Conclusion: The ensemble stacking classifier outperformed other algorithms with an accuracy, sensitivity, specificity, and F1 score with values of 96%, 98%, 98% and 98% respectively and it can be used for non-invasive laboratory-based diagnosis for early prediction of breast cancer.
1. Aruleba K, Obaido G, Ogbuokiri B, Fadaka AO, Klein A, Adekiya TA, et al. Applications of computational methods in biomedical breast cancer imaging diagnostics: A review. J Imaging 2020;6:105.
2. Tse GM, Tan PH. Diagnosing breast lesions by fine needle aspiration cytology or core biopsy: Which is better? Breast Cancer Res Treat 2010;123:1−8.
3. Elston CW, Ellis IO. Pathological prognostic factors in breast cancer. I. The value of histological grade in breast cancer: Experience from a large study with long-term follow-up. Histopathology 1991;19:403−10.
4. Kuhn E, Gambini D, Despini L, Asnaghi D, Runza L, Ferrero S. Updates on lymphovascular invasion in breast cancer. Biomedicines 2023;11:968.
5. Granavel H, Joseph LD, Priya M, Chandrasekharan A, Dev B. Inflammatory Lessions. In: Dev B, Joseph LD, eds. Holistic approach to breast disease. Singapore: Springer; 2023. p. 187−210.
6. Sun H, Ding Q, Sahin AA. Immunohistochemistry in the diagnosis and classification of breast tumors. Arch Pathol Lab Med 2023;147:1119−32.
7. Tardivon AA, Guinebretière JM, Dromain C, Deghaye M, Caillet H, Georgin V. Histological findings in surgical specimens after core biopsy of the breast. Eur J Radiol 2002;42:40−51.
8. Harada TL, Nakashima K, Uematsu T, Sugino T, Nishimura S, Takahashi K, et al. Imaging features of breast cancer with marked hemosiderin deposition: A case report. Eur J Radiol Open 2019;6:302–6.
9. Leftin A, Ben-Chetrit N, Klemm F, Joyce JA, Koutcher JA. Iron imaging reveals tumor and metastasis macrophage hemosiderin deposits in breast cancer. PLoS One 2017;12:e0184765.
10. Gosling SB, Arnold EL, Davies SK, Cross H, Bouybayoune I, Calabrese D, et al. Microcalcification crystallography as a potential marker of DCIS recurrence. Sci Rep 2023;13:9331.
11. Tian Y, Zhao L, Gui Z, Liu S, Liu C, Yu T, et al. Clinical and pathological features analysis of invasive breast cancer with microcalcification. Cancer Med 2023;12:11351−62.
12. Sakibuzzaman M, Mahmud S, Afroze T, Fathma S, Zakia UB, Afroz S, et al. Pathology of breast cancer metastasis and a view of metastasis to the brain. Int J Neurosci 2023;133:544−54.
13. Joneja U, Palazzo J. The spectrum of mucinous lesions of the breast. Arch Pathol Lab Med 2023;147:19−29.
14. Tarin D. Understanding cancer. 1st ed. Cham, Switzerland: Springer; 2023. p. 157−73.
15. Sidey-Gibbons JAM, Sidey-Gibbons CJ. Machine learning in medicine: A practical introduction. BMC Med Res Methodol 2019;19:64.
16. Deo RC. Machine learning in medicine. Circulation. 2015;132:1920−30.
17. Jadoon EK, Khan FG, Shah S, Khan A, ElAffendi M. Deep learning-based multi-modal ensemble classification approach for human breast cancer prognosis. IEEE Access 2023;11:85760– 9.
18. Chan RC, To CKC, Cheng KCT, Yoshikazu T, Yan LLA, Tse GM. Artificial intelligence in breast cancer histopathology. Histopathology 2023;82:198−210.
19. Botlagunta M, Botlagunta MD, Myneni MB, Lakshmi D, Nayyar A, Gullapalli JS, et al. Classification and diagnostic prediction of breast cancer metastasis on clinical data using machine learning algorithms. Sci Rep 2023;13:485.
20. Landis JR, Koch GG. The measurement of observer agreement for categorical data. Biometrics 1977;33:159−74.
21. Rakha EA, Tse GM, Quinn CM. An update on the pathological classification of breast cancer. Histopathology 2023;82:5−16.
22. van Dooijeweert C, van Diest PJ, Willems SM, Kuijpers CCHJ, van der Wall E, Overbeek LIH, et al. Significant inter- and intralaboratory variation in grading of invasive breast cancer: A nationwide study of 33,043 patients in the Netherlands. Int J Cancer 2020;146:769−80.
23. Ginter PS, Idress R, D'Alfonso TM, Fineberg S, Jaffer S, Sattar AK, et al. Histologic grading of breast carcinoma: A multi-institution study of interobserver variation using virtual microscopy. Mod Pathol 2021;34:701−9.
24. Quinn C, Maguire A, Rakha E. Pitfalls in breast pathology. Histopathology 2023;82:140−61.
25. Zebari DA, Ibrahim DA, Zeebaree DQ, Haron H, Salih MS, Damasevicius R, et al. Systematic review of computing approaches for breast cancer detection based computer aided diagnosis using mammogram images. Appl Artif Intell 2021;35:2157– 203.
26. Öztürk Ş, Akdemir B. Application of feature extraction and classification methods for histopathological image using GLCM, LBP, LBGLCM, GLRLM and SFTA. Procedia Comput Sci 2018;132:40–6.
27. Becker AS, Marcon M, Ghafoor S, Wurnig MC, Frauenfelder T, Boss A. Deep learning in mammography: Diagnostic accuracy of a multipurpose image analysis software in the detection of breast cancer. Invest Radiol 2017;52:434−40.
28. Darweesh MS, Adel M, Anwar A, Farag O, Kotb A, Adel M. Early breast cancer diagnostics based on hierarchical machine learning classification for mammography images. Cogent Eng 2021;8:1968324.
29. Wetstein SC, de Jong VMT, Stathonikos N, Opdam M, Dackus GMHE, Pluim JPW, et al. Deep learning-based breast cancer grading and survival analysis on whole-slide histopathology images. Sci Rep 2022;12:15102.
30. Shin SJ, Roh J, Kim M, Jung MJ, Koh YW, Park CS, et al. Prognostic significance of absolute lymphocyte count/absolute monocyte count ratio at diagnosis in patients with multiple myeloma. Korean J Pathol 2013;47:526−33.
31. Johnson PJ, Dhanaraj S, Berhane S, Bonnett L, Ma YT. The prognostic and diagnostic significance of the neutrophil-tolymphocyte ratio in hepatocellular carcinoma: A prospective controlled study. Br J Cancer 2021;125:714−6.
32. Pereira-Veiga T, Bravo S, Gómez-Tato A, Yáñez-Gómez C, Abuín C, Varela V, et al. Red blood cells protein profile is modified in breast cancer patients. Mol Cell Proteomics 2022;21:100435.
33. Wang Y, Wang H, Yin W, Lin Y, Zhou L, Sheng X, et al. Novel lymphocyte to red blood cell ratio (LRR), neutrophil to red blood cell ratio (NRR), monocyte to red blood cell ratio (MRR) as predictive and prognostic biomarkers for locally advanced breast cancer. Gland Surg 2019;8:627−35.
34. Kasimir-Bauer S, Karaaslan E, Hars O, Hoffmann O, Kimmig R. In early breast cancer, the ratios of neutrophils, platelets and monocytes to lymphocytes significantly correlate with the presence of subsets of circulating tumor cells but not with disseminated tumor cells. Cancers (Basel) 2022;14:3299.
35. Burçak KC, Baykan ÖK, Uğuz H. A new deep convolutional neural network model for classifying breast cancer histopathological images and the hyperparameter optimisation of the proposed model. J Supercomput 2021;77:973–89.
36. Vakharia V, Shah M, Suthar V, Patel VK, Solanki A. Hybrid perovskites thin films morphology identification by adapting multiscale-SinGAN architecture, heat transfer search optimized feature selection and machine learning algorithms. Phys Scr 2023;98:025203.
37. Vakharia V, Shah M, Nair P, Borade H, Sahlot P, Wankhede V. Estimation of lithium-ion battery discharge capacity by integrating optimized explainable-AI and stacked LSTM model. Batter 2023;9:125.
38. Vakharia V, Gupta VK, Kankar PK. A comparison of feature ranking techniques for fault diagnosis of ball bearing, Soft Comput 2016;20:1601–19.
39. Falessi D, Huang J, Narayana L, Thai JF, Turhan B. On the need of preserving order of data when validating within-project defect classifiers. Empir Softw Eng 2020;25:4805–30.
40. Syed-Abdul S, Firdani RP, Chung HJ, Uddin M, Hur M, Hyeon J, et al. Artificial intelligence based models for screening of hematologic malignancies using cell population data. Sci Rep 2020;10:4583.
41. Jalali A, Miresse D, Fahey MR, Ni Mhaonaigh N, McGuire A, Bourke E, et al. Peripheral blood cell ratios as prognostic indicators in a neoadjuvant chemotherapy-treated breast cancer cohort. Curr Oncol 2022;29:7512−23.
42. Luo L, Tan Y, Zhao S, Yang M, Che Y, Li K, et al. The potential of high-order features of routine blood test in predicting the prognosis of non-small cell lung cancer. BMC Cancer 2023;23:496.
43. Zhang YD, Satapathy SC, Guttery DS, Górriz JM, Wang SH. Improved breast cancer classification through combining graph convolutional network and convolutional neural network. Inf Process Manag 2021;58:102439.