Modeling and Forecasting for the number of cases of the COVID-19 pandemic with the Curve Estimation Models, the Box-Jenkins and Exponential Smoothing Methods
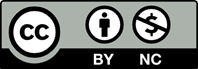
Objectives: This study aims to provide statistical information summarizing the general structure about the effects and process of infection in all countries of the world in the light of the data obtained and to model the daily change of infection criteria.
Methods: The number of COVID 19 epidemic cases of Turkey and the selected G8 countries, Germany, United Kingdom, France, Italy, Russia, Canada, Japan between 1/22/2020 and 3/22/2020 has been estimated and forecasted in this study by using some curve estimation models, Box-Jenkins (ARIMA) and Brown/Holt linear exponential smoothing methods.
Results: Japan (Holt Model), Germany (ARIMA (1,4.0)) and France (ARIMA (0,1,3)) provide statistically significant but clinically unqualified results in this data set. UK (Holt Model), Canada (Holt Model), Italy (Holt Model), Turkey (ARIMA (1,4,0)) and Russia?? the results are more reliable. This is specified for the particular model used in this case Turkey.
Conclusion: Certainly, more accurate evaluations can be made with more data in future studies. Nevertheless, since this study provides information about the levels at which the number of cases may extend in case that the current situation is not intervened, it can guide countries to take the necessary measures and to intervene it earlier.
1.WHO. 2020 [World Health Organization ]. Available from: https://www.who.int/emergencies/diseases/novel-coronavirus-2019/events-as-they-happen.
2. Farebrother R. Further results on the mean square error of ridge regression. Journal of the Royal Statistical Society Series B (Methodological). 1976;38(3):248-50.
3. Rao CR, Toutenburg H. Linear models. Linear models: Springer; 1995. p. 3-18.
4. Robinson PM. Root-N-consistent semiparametric regression. Econometrica: Journal of the Econometric Society. 1988:931- 54.
5. Tekindal MA, Yazici AC, Yavuz Y. The modelling of time-series and the evaluation of forecasts for the future: the case of the number of persons per physician in turkey between 1928 and 2010. Biomedical Research. 2016;27(3).
6. Fischer B. Decomposition of time series: comparing different methods in theory and practice: Eurostat; 1995.
7. Gujarati DN, Porter DC. Basic econometrics (ed.). New York: McGraw-HiII. 2003.
8. Yenice S, Tekindal MA. Forecasting the stock indexes of fragile five countries through Box-Jenkins methods. International Journal of Business and Social Science. 2015;6(8):180-91.
9. Dickey DA, Fuller WA. Likelihood ratio statistics for autoregressive time series with a unit root. Econometrica: journal of the Econometric Society. 1981:1057-72.
10. Box GE, Jenkins GM, Reinsel GC, Ljung GM. Time series analysis: forecasting and control: John Wiley & Sons; 1976.
11. Wickramarachchi A, Herath H, Jayasinghe-Mudalige U, Edirisinghe J, Udugama J, Lokuge L, et al. An Analysis of price behavior of major poultry products in Sri Lanka. Journal of Agricultural Sciences–Sri Lanka. 2017;12(2).
12. Brockwell PJ, Davis RA. Introduction to time series and forecasting: springer; 2016.
13. Brown RG. Exponential smoothing for predicting demand. cambridge, mass., arthur d. little. Inc; 1956.
14. Holt CC. Forecasting trends and seasonals by exponentially weighted averages. carnegie institute of technology. Pittsburgh ONR memorandum; 1957.
15. Winters PR. Forecasting sales by exponentially weighted moving averages. Management science. 1960;6(3):324-42.