A deep-learning model using chest computed tomography images to predict epidermal growth factor receptor (EGFR) T790M mutation after first-line treatment with EGFR-tyrosine kinase inhibitor in patients with non-small cell lung cancer
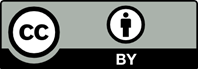
To predict the epidermal growth factor receptor (EGFR) T790M status of patients with advanced non-small cell lung cancer (NSCLC) following the first-line first-/second-generation EGFR-tyrosine kinase inhibitor (EGFR-TKI) therapy, the related clinical features and chest computed tomography (CT) images of patients with advanced NSCLC in our hospital were retrospectively collected. All patients who met the criteria were randomly divided into training and validation cohorts. Then, a clinical model with the filtered clinical characteristics and a deep-learning model (DLM) were constructed. The area under the curve (AUC), specificity, sensitivity, accuracy, and decision curve analysis were used to evaluate model performance. In total, 66 patients met the inclusion criteria of the study (training cohort, n = 40; validation cohort, n = 26). EGFR19del and the use of gefitinib were significant (P < 0.05), and then, the clinical model was established using multivariate logistic regression analysis. The AUCs of the clinical model were 0.862 (95% confidence interval [CI], 0.570 – 0.966) and 0.755 (0.566 – 0.943) in the training and validation cohorts, respectively. The AUCs of the DLM from the chest CT image analysis were 0.839 (95% CI, 0.708 – 0.970) and 0.842 (0.680 – 1.000) in the training and validation cohorts, respectively. In the validation cohort, the DLM and clinical model exhibited an accuracy of 0.7308 and 0.5000, specificity of 0.6667 and 0.2000, positive probability values of 0.6429 and 0.4545, and negative probability values of 0.8333 and 0.7500, respectively. The DLM was developed using chest CT images to predict the EGFR T790M status following the first-line first- and second-generation EGFR-TKI treatment of advanced EGFR-positive NSCLC.
- Bray F, Laversanne M, Sung H, et al. Global cancer statistics 2022: GLOBOCAN estimates of incidence and mortality worldwide for 36 cancers in 185 countries. CA Cancer J Clin. 2024;74(3):229-263. doi: 10.3322/caac.21834
- Siegel RL, Giaquinto AN, Jemal A. Cancer statistics, 2024. CA Cancer J Clin. 2024;74(1):12-49. doi: 10.3322/caac.21820
- Sung H, Ferlay J, Siegel RL, et al. Global cancer statistics 2020: GLOBOCAN estimates of incidence and mortality worldwide for 36 cancers in 185 countries. CA Cancer J Clin. 2021;71(3):209-249. doi: 10.3322/caac.21660
- Barta JA, Powell CA, Wisnivesky JP. Global epidemiology of lung cancer. Ann Glob Health. 2019;85(1):8. doi: 10.5334/aogh.2419
- Gillespie CS, Mustafa MA, Richardson GE, et al. Genomic alterations and the incidence of brain metastases in advanced and metastatic NSCLC: A systematic review and meta-analysis. J Thorac Oncol. 2023;18(12):1703-1713. doi: 10.1016/j.jtho.2023.06.017
- Chen P, Liu Y, Wen Y, Zhou C. Non-small cell lung cancer in China. Cancer Commun (Lond). 2022;42(10):937-970. doi: 10.1002/cac2.12359
- Lahiri A, Maji A, Potdar PD, et al. Lung cancer immunotherapy: Progress, pitfalls, and promises. Mol Cancer. 2023;22(1):40. doi: 10.1186/s12943-023-01740-y
- Miller M, Hanna N. Advances in systemic therapy for non-small cell lung cancer. BMJ. 2021;375:n2363. doi: 10.1136/bmj.n2363
- Wu J, Lin Z. Non-small cell lung cancer targeted therapy: Drugs and mechanisms of drug resistance. Int J Mol Sci. 2022;23(23):15056. doi: 10.3390/ijms232315056
- Kim ES, Melosky B, Park K, Yamamoto N, Yang JC. EGFR tyrosine kinase inhibitors for EGFR mutation-positive non-small-cell lung cancer: Outcomes in Asian populations. Future Oncol. 2021;17(18):2395-2408. doi: 10.2217/fon-2021-0195
- Batra U, Biswas B, Prabhash K, Krishna MV. Differential clinicopathological features, treatments and outcomes in patients with exon 19 deletion and exon 21 L858R EGFR mutation-positive adenocarcinoma non-small-cell lung cancer. BMJ Open Respir Res. 2023;10(1):e001492. doi: 10.1136/bmjresp-2022-001492
- Chen J, Yang H, Teo ASM, et al. Genomic landscape of lung adenocarcinoma in East Asians. Nat Genet. 2020;52(2): 177-186. doi: 10.1038/s41588-019-0569-6
- Riely GJ, Wood DE, Ettinger DS, et al. Non-small cell lung cancer, version 4.2024, NCCN clinical practice guidelines in oncology. J Natl Compr Canc Netw. 2024;22(4):249-274. doi: 10.6004/jnccn.2204.0023
- Hendriks LE, Kerr KM, Menis J, et al. Oncogene-addicted metastatic non-small-cell lung cancer: ESMO Clinical Practice Guideline for diagnosis, treatment and follow-up. Ann Oncol. 2023;34(4):339-357. doi: 10.1016/j.annonc.2022.12.009
- Hosomi Y, Morita S, Sugawara S, et al. Gefitinib alone versus gefitinib plus chemotherapy for non-small-cell lung cancer with mutated epidermal growth factor receptor: NEJ009 study. J Clin Oncol. 2020;38(2):115-123. doi: 10.1200/jco.19.01488
- Jiang Z, Zhang J, Sun H, et al. Icotinib alone or with bevacizumab as first-line therapy in Chinese patients with advanced nonsquamous non-small cell lung cancer and activating EGFR mutations: A retrospective study. Thorac Cancer. 2021;12(17):2369-2374. doi: 10.1111/1759-7714.14079
- Goldberg SB, Redman MW, Lilenbaum R, et al. Randomized trial of afatinib plus cetuximab versus afatinib alone for first-line treatment of EGFR-mutant non-small-cell lung cancer: Final results from SWOG S1403. J Clin Oncol. 2020;38(34):4076-4085. doi: 10.1200/jco.20.01149
- Wu YL, Cheng Y, Zhou X, et al. Dacomitinib versus gefitinib as first-line treatment for patients with EGFR-mutation-positive non-small-cell lung cancer (ARCHER 1050): A randomised, open-label, phase 3 trial. Lancet Oncol. 2017;18(11):1454-1466. doi: 10.1016/s1470-2045(17)30608-3
- Kanaji N, Yokohira M, Nakano-Narusawa Y, et al. Hepatocyte growth factor produced in lung fibroblasts enhances non-small cell lung cancer cell survival and tumor progression. Respir Res. 2017;18:118. doi: 10.1186/s12931-017-0604-z
- Dong RF, Zhu ML, Liu MM, et al. EGFR mutation mediates resistance to EGFR tyrosine kinase inhibitors in NSCLC: From molecular mechanisms to clinical research. Pharmacol Res. 2021;167:105583. doi: 10.1016/j.phrs.2021.105583
- He J, Huang Z, Han L, Gong Y, Xie C. Mechanisms and management of 3rd-generation EGFR-TKI resistance in advanced non-small cell lung cancer (review). Int J Oncol. 2021;59(5):90. doi: 10.3892/ijo.2021.5270
- Wu SG, Shih JY. Management of acquired resistance to EGFR TKI-targeted therapy in advanced non-small cell lung cancer. Mol Cancer. 2018;17(1):38. doi: 10.1186/s12943-018-0777-1
- Borgeaud M, Parikh K, Banna GL, et al. Unveiling the landscape of uncommon EGFR mutations in NSCLC-a systematic review. J Thorac Oncol. 2024;19(7):973-983. doi: 10.1016/j.jtho.2024.03.016
- Patil BR, Bhadane KV, Ahmad I, et al. Exploring the structural activity relationship of the Osimertinib: A covalent inhibitor of double mutant EGFRL858]R/T790]M tyrosine kinase for the treatment of Non-small Cell Lung Cancer (NSCLC). Bioorg Med Chem. 2024;109:117796. doi: 10.1016/j.bmc.2024.117796
- Nagano T, Tachihara M, Nishimura Y. Mechanism of resistance to epidermal growth factor receptor-tyrosine kinase inhibitors and a potential treatment strategy. Cells. 2018;7(11):212. doi: 10.3390/cells7110212
- Johnson M, Garassino MC, Mok T.Mitsudomi T. Treatment strategies and outcomes for patients with EGFR-mutant non-small cell lung cancer resistant to EGFR tyrosine kinase inhibitors: Focus on novel therapies. Lung Cancer. 2022;170:41-51. doi: 10.1016/j.lungcan.2022.05.011
- Christopoulos P, Kirchner M, Roeper J, et al. Risk stratification of EGFR+ lung cancer diagnosed with panel-based next-generation sequencing. Lung Cancer. 2020;148:105-112. doi: 10.1016/j.lungcan.2020.08.007
- Canale M, Petracci E, Delmonte A, et al. Impact of TP53 mutations on outcome in EGFR-mutated patients treated with first-line tyrosine kinase inhibitors. Clin Cancer Res. 2017;23(9):2195-2202. doi: 10.1158/1078-0432.CCR-16-0966
- Christopoulos P, Budczies J, Kirchner M, et al. Defining molecular risk in ALK+ NSCLC. Oncotarget. 2019;10(33):3093-3103. doi: 10.18632/oncotarget.26886
- Offin M, Rizvi H, Tenet M, et al. Tumor mutation burden and efficacy of EGFR-tyrosine kinase inhibitors in patients with EGFR-mutant lung cancers. Clin Cancer Res. 2019;25(3):1063-1069. doi: 10.1158/1078-0432.CCR-18-1102
- Menzel M, Kirchner M, Kluck K, et al. Genomic heterogeneity at baseline is associated with T790M resistance mutations in EGFR-mutated lung cancer treated with the first-/second-generation tyrosine kinase inhibitors. J Pathol Clin Res. 2024;10(2):e354. doi: 10.1002/cjp2.354
- Min Kim H, Ko T, Young Choi I, Myong JP. Asbestosis diagnosis algorithm combining the lung segmentation method and deep learning model in computed tomography image. Int J Med Inform. 2021;158:104667. doi: 10.1016/j.ijmedinf.2021.104667
- Wang S, Liu Z, Chen X, et al. Unsupervised deep learning features for lung cancer overall survival analysis. Annu Int Conf IEEE Eng Med Biol Soc. 2018;2018:2583-2586. doi: 10.1109/embc.2018.8512833
- Chen X, Feng B, Chen Y, et al. A CT-based deep learning model for subsolid pulmonary nodules to distinguish minimally invasive adenocarcinoma and invasive adenocarcinoma. Eur J Radiol. 2021;145:110041. doi: 10.1016/j.ejrad.2021.110041
- Chen X, Feng B, Xu K, et al. Development and validation of a deep learning radiomics nomogram for preoperatively differentiating thymic epithelial tumor histologic subtypes. Eur Radiol. 2023;33(10):6804-6816. doi: 10.1007/s00330-023-09690-1
- Feng B, Shi J, Huang L, et al. Robustly federated learning model for identifying high-risk patients with postoperative gastric cancer recurrence. Nat Commun. 2024;15(1):742. doi: 10.1038/s41467-024-44946-4
- Liu PM, Feng B, Shi JF, et al. A deep-learning model using enhanced chest CT images to predict PD-L1 expression in non-small-cell lung cancer patients. Clin Radiol. 2023;78(10):e689-e697. doi: 10.1016/j.crad.2023.05.010
- Mok TS, Wu YL, Ahn MJ, et al. Osimertinib or platinum-pemetrexed in EGFR T790M-positive lung cancer. N Engl J Med. 2017;376(7):629-640. doi: 10.1056/NEJMoa1612674
- Blair HA. Befotertinib: First approval. Drugs. 2023;83(15):1433-1437. doi: 10.1007/s40265-023-01946-w
- Cheng WC, Hsia TC, Tu CY, Chen HJ. The impact of acquired EGFR T790M mutation and EGFR circulating cell-free DNA on survival in patients with lung adenocarcinoma following EGFR-TKI therapy. Onco Targets Ther. 2020;13:13425-13435. doi: 10.2147/ott.S279540
- Lin YT, Chen JS, Liao WY, et al. Clinical outcomes and secondary epidermal growth factor receptor (EGFR) T790M mutation among first-line gefitinib, erlotinib and afatinib-treated non-small cell lung cancer patients with activating EGFR mutations. Int J Cancer. 2019;144(11):2887-2896. doi: 10.1002/ijc.32025
- Nam Y, Kim HC, Kim YC, et al. Clinical impact of rebiopsy among patients with epidermal growth factor receptor-mutant lung adenocarcinoma in a real-world clinical setting. Thorac Cancer. 2021;12(6):890-898. doi: 10.1111/1759-7714.13857
- Wang Z, Chen R, Wang S, et al. Quantification and dynamic monitoring of EGFR T790M in plasma cell-free DNA by digital PCR for prognosis of EGFR-TKI treatment in advanced NSCLC. PLoS One. 2014;9(11):e110780. doi: 10.1371/journal.pone.0110780
- Lu S, Dong X, Jian H, et al. AENEAS: A randomized phase III trial of aumolertinib versus gefitinib as first-line therapy for locally advanced or metastaticnon-small-cell lung cancer with EGFR exon 19 deletion or L858R mutations. J Clin Oncol. 2022;40(27):3162-3171. doi: 10.1200/jco.21.02641
- Ke EE, Zhou Q, Zhang QY, et al. A higher proportion of the EGFR T790M mutation may contribute to the better survival of patients with exon 19 deletions compared with those with L858R. J Thorac Oncol. 2017;12(9):1368-1375. doi: 10.1016/j.jtho.2017.05.018
- Dickerson H, Diab A, Al Musaimi O. Epidermal growth factor receptor tyrosine kinase inhibitors in cancer: Current use and future prospects. Int J Mol Sci. 2024;25(18):10008. doi: 10.3390/ijms251810008