Radiomics: Phases of osteoclastic metastasis status in breast cancer identified by morphologic markers
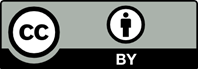
In the practical work of radiologists or oncologists, particularly in individualized treatment, a rapid and accurate diagnosis, timely assessments of drug effects, and direction of disease progression are essential. Radiomics and neural networks offer significant help in analyzing data from diagnostic imaging studies. This study examines quantitative biomarkers derived from magnetic resonance imaging, tentatively categorized as mathematical morphological markers, and explores their relationship with osteoclast tumor regression in breast cancer. This study aims to determine the consistency of imaging biomarkers in the stabilization, healing, and progression of breast cancer bone metastases.
- Cardoso F, Paluch-Shimon S, Schumacher-Wulf E, et al. 6th and 7th International consensus guidelines for the management of advanced breast cancer (ABC guidelines 6 and 7). Breast. 2024;76:103756. doi: 10.1016/j.breast.2024.103756
- Regentova OS, Solodky VA, Bozhenko VK, et al. Radiomic data analysis in neuro-oncology. Bull Russ Sci Cent Roentgenol. 2024;24:69-77.
- Jing G, Chen Y, Ma X, et al. Predicting mismatch-repair status in rectal cancer using multiparametric radiomics mri-based models: A preliminary study. Biomed Res Int. 2022;2022:6623574. doi: 10.1155/2022/6623574
- Hu Z, Zhuang Q, Xiao Y, et al. MIL normalization-- prerequisites for accurate MRI radiomics analysis. Comput Biol Med. 2021;133:104403. doi: 10.1016/j.compbiomed.2021.104403
- Tang WT, Su CQ, Lin J, Xia ZW, Lu SS, Hong XN. T2-FLAIR mismatch sign and machine learning-based multiparametric MRI radiomics in predicting IDH mutant 1p/19q non-co-deleted diffuse lower-grade gliomas. Clin Radiol. 2024;79(5):e750-e758. doi: 10.1016/j.crad.2024.01.021
- Sergeev NI, Kotlyarov PM, Teplyakov VV, Solodkiy VA. Peculiarities of application of diagnostic visualization methods in evaluation of treatment results of bone metastases. Russ Electron J Radiat Diagn. 2021;11:84-93. doi: 10.21569/2222-7415-2021-11-4-84-93
- Koob S, Kehrer M, Strauss A, Janzen V, Wirtz VC, Schmolders J. Knochenmetastasen-pathophysiologie, diagnostik und therapie (Teil 1) [Bone metastases-pathophysiology, diagnostic testing and therapy (part 1)]. Z Orthop Unfall. 2017;155(6):716-726. doi: 10.1055/s-0043-116799
- Ghanem N, Uhl M, Brink I, et al. Diagnostic value of MRI in comparison to scintigraphy, PET, MS-CT and PET/ CT for the detection of metastases of bone. Eur J Radiol. 2005;55(1):41-55. doi: 10.1016/j.ejrad.2005.01.016
- Source Forge DCM4CHE. DICOM Implementation in JAVA Files. Available from: https://sourceforge.net/projects/ dcm4che/files/dcm4che3 [Last accessed on 2024 Nov 11].
- Yue W, Hui W, Biaobiao Z, et al. Using radial basis function networks for function approximation and classification. Int Sch Res Not ISRN Appl Math. 2012;324194:34. doi: 10.5402/2012/324194
- Steinhauer V, Sergeev NI. Radiomics in breast cancer: In-depth machine analysis of MR images of metastatic spine lesion. Sovrem Tehnol Med. 2022;14(2):16-24. doi: 10.17691/stm2022.14.2.02
- Steinhauer V, Hartung G. Radiomics and quantitative MDA criteria in breast cancer with bone metastases by MRI: Examples of calculation algorithms and their practical use. Sovrem Tehnol Med. 2024;16(3):5-10. doi: 10.17691/stm2024.16.3.01
- Danilenko VI, Simmetriya IR. Metodologiya I Morfogenez [Symmetry and Cancer. Methodology and Morphogenesis]. Saarbrücken, Germany: Lap Lambert Academic Publishing; 2017. p. 85.
- Hanahan D, Weinberg RA. Hallmarks of cancer: The next generation. Cell. 2011;144(5):646-674. doi: 10.1016/j.cell.2011.02.013
- Wietecha MS, Lauenstein D, Cangkrama M, et al. Phase-specific signatures of wound fibroblasts and matrix patterns define cancer-associated fibroblast subtypes. Matrix Biol. 2023;119:19-56. doi: 10.1016/j.matbio.2023.03.003
- Maccarthy-Morrogh L, Martin P. The hallmarks of cancer are also the halmarks of wound healing. Sci Signal. 2020;13(648):eaay8690. doi: org/10.1126/scisignal.aay8690
- Koroban NV. Monitoring the effectiveness of osteoporosis therapy in HIV infection. HIV Infect Immunosuppression. 2017;9(2):91-94. doi: 10.22328/2077-9828-2017-9-2-91-94
- Karateev DE, Luchikhina EL. Current treatment for spondyloarthritis: focus on netakimab. A review. Terapevticheskii Arkhiv (Ter. Arkh.). 2022;18(8):8-15. doi: 10.26442/00403660.2024.05.202794
- Steinhauer V, Sergeev NI. Radiomics and density motphology of osteoblastic metastases of prostate cancer according to MSCT data during therapy. Interdiscip Sci Pract J. 2024;18(2):62-70.
- Neuroph. Java Neural Network Framework. Version 2.98. Available from: https://neuroph.sourceforge.net [Last accessed on 2024 Nov 11].