Predictability of Severe Thunderstorms with Fractal Dimension Approach
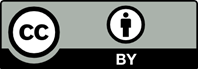
Thunderstorm is meso-scale weather with space scale varying from a few kilometres to a couple of 100 kilometres and time scale varying from less than an hour to several hours. Severe thunderstorms, however, produce strong surface wind squalls, lightning, heavy rain showers, occasional hail, down-bursts and tornadoes leading to loss of life and property on the ground and aviation hazard aloft. Prediction of severe thunderstorm is a challenging task for the atmospheric scientists around the globe. The rationale of the present study is, thus, to view the relative significance of two important convective energies, convective available potential energy (CAPE) and convective inhibition energy (CIN) in the genesis of severe thunderstorms during the pre-monsoon season (April ñ May)over Kolkata (22°32‘N, 88°20’E). The concept of fractal dimension is applied in this study to observe the degree of self- similarity between CAPE and CIN for the prevalence of severe thunderstorms during the pre-monsoon season. Fractal dimension of CAPE and CIN is measured with the help of the level of free convection (LFC). The fractal dimension is considered in this study as the measure of randomness. The result reveals that CIN has more self- similarity than CAPE for the genesis of severe thunderstorms over Kolkata.
Abraham, A., Philip, N.S. and B. Joseph (2001). Will we have a wet summer? Long term rain forecasting using Soft Computing models. In: Kerchoffs, E.J.H. and Snorek, M. (eds), Modelling and Simulation. Society for Computer Simulation International. Prague, Czech Republic, pp. 1044-1048.
Barabasi, A.L. and H.E. Stanley (1995). Fractal Concepts in Surface Growth. Cambridge University Press, Cambridge.
Chaudhuri, S. (2005). Genetic Algorithm to recognize Apt Energy for the genesis of Severe Thunderstorms. Vatabaran, 29(2): 1-8.
Chaudhuri, S. (2006). Predictability of Chaos inherent in the occurrence of Severe Thunderstorms. Advances in Complex Systems, 9(1&2):1-9.
Chaudhuri, S. (2006). Artificial Neural Network Model to forecast maximum wind speed associated with severe thunderstorms. Vatabaran, AFAC Journal of Meteorology,30(1): 14-19.
Chaudhuri, S. and S. Aich Bhowmik (2006). CAPE ñ A link between Thermodynamics and Microphysics for the occurrence of Severe Thunderstorms. Mausam ñ Q. J. Meteorology, Hydrology & Geophysics, 57(2): 249-254.
Chaudhuri, S. (2008a). Preferred Type of Cloud in the Gen of Severe Thunderstorms ñ A Soft Computing Approach. Atmospheric Research, 88: 149-156.
Chaudhuri,S.(2008b).Identification ofthe level ofdowndrafformation during severe thunderstorms: A frequencydomain analysis. Meteorology & Atmospheric Physics,102(1-2):123-129.
Chaudhuri,S. and A. Middey (2009). The applicability ofBipartite Graph Model for Thunderstorm Forecast overKolkata. Advances in Meteorology, doi:10.1155/2009270530
Coulibaly,P., Bob'ee, B. and F.Anctil (2001). Improvingextreme hydrologic events forecasting using a new criterionfor ANN selection. Hydrol Process, 15(8):1533-1536.
Desai, B.N. and Y.P. Rao (1954). On the cold pools and theirrole in the development of Nor’westers over West Bengaand East Pakistan. Ind.J. Meteorol. Geophys, 5: 243-248
El-Shafie,A., Reda Taha, M. and A. Noureldin (2007).Aneuro-fuzzy model for inflow forecasting of the Nile river atAswan high dam. Water Resour Manage, 21:533-556.
Elshorbagy,A.and S.P. Simonovic (2000). Performanceevaluation ofartificial neural networks for runoffprediction.JournalofHydrologic Engineering, ASCE, 5(4):424-427
Fernando, D.A.and A.W. Jayawardena (1998). Runoffforecasting using RBF networks with OLS algorithmJournalofHydrologic Engineering, ASCE, 3(3): 203-209
Handerson, H.W. and R. Wells (1988). Obtaining attractordimensions from meteorological time series. Advances inGeophysics, 30:205.
Hsieh, W.W. and T. Tang (1998).Applying Neural NetworkModels to Prediction and Data Analysis in Meteorologyand Oceanography. Bulletin ofthe American Meteorologica!Society,79:1855-1869.
Jacovides, C.P. and T. Yonetani (1990). An evaluation ofStability indices for Thunderstorm prediction in GreaterCyprus.American Meteorological Society, 559-569.
Jin, L., Yao, C. and Y.X.Huang (2008).A Nonlinear ArtificialIntelligence Ensemble Prediction Model for TyphoonIntensity. Monthly Weather Review, 136: 4541-4554.
Kandalgaokar,S.S., Tinmaker, M..R., Kulkarni, M.K. andAsha Nath (2002). Thunderstorm activity and sea surfacetemperature over the Island stations and along the east andwest coast ofIndia. Mausam,53:245.
Kuligowski, R.1. and A.P. Barros (1998). Localized Preci.pitation Forecasts from a Numerical Weather PredictionModel Using Artificial Neural Networks. Weather andForecasting, 13: 1194-1204.
Manohar, GK., Kandalgaokar,S.S. and M.l.R. Tinmaker(1999). Thunderstorm activity over India and Indian southwest monsoon.J. Geophys. Res.. 104:4169.
Mandelbrot, B.B.(1982). The Fractal Geometry ofthe Nature.Freeman, San Francisco.Marzban, C. and G. Stumpf (1998). A Neural Network forDamaging Wind Prediction. Weather and Forecasting, 13:151-163.
Marzban, C. and A. Witt (2001).A Bayesian neural networkfor severe hail size prediction. Weather and Forecasting16:600-610.
McCann, D.W.(1992).ANeural Network Short-Term Forecastof Significant Thunderstorms. Weather and Forecasting7:525-534.
Perez, P. and J. Reyes (2001). Prediction of particulate airpollution using neural techniques. Neural Computing andApplication,10:165-171.
Rajurkar, M.P., Kothyari, U.C. and U.C. Chaube (2004).Modeling of the daily rainfall-runoff relationship withartificial neural network.Journal ofHydrology,285(1-4):96-113.
Seker, H., Odetayo, M.O., Petrovic, D. and R.N.G. Naguib(2003).A fuzzylogic based method for prognostic decisionmaking in breast and prostate cancers. lEEE Trans InfTechnol Biomed, 7(2):114-122.
Sharifi, M.B. and K.P. Geograkakos(1990). Evidence ofdeterministic chaos in the pulse of storm rainfall. J. oAtmospheric Sciences, 47:888.
Sivakumar, B., Liong, S.Y. and C.Y. Liaw (1998). Evidence of chaotic behavior in Singapore rainfall. Journal of American Water Resources Association, 34: 301.
Shao, J. (1997). Improving Nowcasts of Road Surface Temperature by a Backpropagation Neural Network. Wea. Forecasting, 13: 164-171.
Tokar, A.S. and M. Markus (2000). Precipitationñrunoff modeling using artificial neural networks and conceptual models. Journal of Hydrologic Engineering, 5(2): 156-161.
Wang, Y., Yu, T.Y., Yeary, M., Shapiro, A., Nemati, S., Foster, M.,Andra, D.L. (Jr.) and Jain, M. (2008). Tornado Detection Using a NeuroñFuzzy System to Integrate Shear and Spectral Signatures. Journal of Atmospheric and Oceanic Technology, 25(7): 1136-1148.
William (1995). Handbook of Atmospheric Electrodynamics. CRC Press, London.
Yu, P.S. and C.J. Chen (2000). Application of gray and fuzzy methods for rainfall forecasting. J Hydrol Eng, 4(5): 339- 345.
Zealand, C.M., Burn, D.H. and S.P. Simonovic (1999). Short term stream flow forecasting using artificial neural networks. Journal of Hydrology, Amsterdam, 214: 32-48.