Use of Back-propagation Artificial Neural Networks for Groundwater Level Simulation
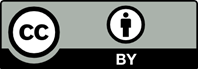
This article presents simulation of groundwater level fluctuation based on an artificial neural network modelling. The prediction used multi-layer back-propagation neural networks (BPANN). The case of study area was Jakarta, Indonesia, that has high population density and several purposes of groundwater resource usage. Input variables were using delay five-daily groundwater level fluctuation (GLF) of observation well interest to predict current GLF. The applicability of BPANN for GLF prediction was verified in three sets of input variables. The result showed that application of BPANN to simulate GLF gives satisfied prediction results.
Amari, S., Murata, N., Müller, K.R., Finke, M. and H. Yang(1997). Asymptotic statistical theory of overtraining and cross-validation. IEEE Transactions on Neural Network, 8(5): 985-996.
Aziz, A.R.A. and K.F.V. Wong (1992). Neural network approach to the determination of aquifer parameter. Ground Water, 30(2): 164-166.
Balkhair, K.S. (2002). Aquifer parameters determination for large diameter wells using neural network approach.Hydrol., 265: 118-128.
Coulibaly, P., Anctil, F., Aravena, R. and B. Bobée (2001). Artificial neural network modeling of water table depth fluctuations. Water Resources Research, 37(4): 885-896.
Daliakopoulos, L.N., Coulibaly, P. and I.K. Tsanis (2005). Groundwater level forecasting using artificial neural networks. J. Hydrol., 309: 229-240.
Govindaraju, R.S. (2000). Artificial neural networks in hydrology. I: Preliminary concepts. By the ASCE task Committee on application of artificial neural networks in hydrology. J. Hydrol. Eng. 5: 115-123.
Kamp, R.G. and H.H.G. Savenije (2006). Optimising training data for ANNs with genetic algorithms. Hydrol. Earth Syst. Sci. Discuss., 3: 285-297.
Lallahem, S., Mania, J., Hani, A. and Y. Najjar (2005). On the use of neural networks to evaluate groundwater levels in fractured media. J. of Hydrol., 307: 92-111.
Maier, H.R. and G.C. Dandy (2000). Neural networks for the prediction and forecasting of water resources variables: A review of modelling issues and applications. Environmental Modelling & Software, 15: 101-124.
McIntosh A.C. (2003). Asian water supplies: Reaching the urban poor. A guide and sourcebook on urban water supplies in Asia for governments, utilities, consultants, development agencies, and nongovernment organizations. Asian Development Bank and International Water Association, 137 p.
Nayak, P.C., Satyaji Rao, Y.R. and K.P. Sudheer (2006). Groundwater level forecasting in a shallow aquifer using artificial neural network approach. Water Resources Management, 20: 77-90.
Rajanayaka, C., Samarasinghe, S. and D. Kulasiri (2002). Solving the Inverse Problem in Stochastic Groundwater Modelling with Artificial Neural Networks. Proc. iEMSs 2002 sessions (part two), 154-159. Available at:http://www.iemss.org/iemss2002/proceedings/pdf/ volume%20due/385_rajanayaka.pdf
Ranjithan, S., Eheart, J.W. and J.H. Garrett (1993). Neural network-based screening for groundwater reclamation under uncertainty. Water Resources Research, 29(3): 563-574.
Rumelhart, D., Hinton, G.E. and R. Williams (1986). Learning representations by back-propagation errors. Nature, 323: 533-536.
Soetrisno, S. (1999). Groundwater management problem: A Jakarta and Bandung (Indonesia) comparative city case studies. Available at: http://www.geocities.com/Eureka/ Gold/1577/index.html.
Tirtomiharjo, H. (1996). Urban groundwater database. Directorate of Environmental Geology of Indonesia. Available at: http://www.scar.utoronto.ca/~gwater/ IAHCGUA/UGD/jakarta.html.