Assessing Limits of Classification Accuracy Attainable through Maximum Likelihood Method in Remote Sensing
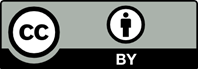
The signal reaching the satellite is mix-up of contributions from varied features on the ground surface besides atmospheric errors. Getting back the information on ground features from satellite-based data is an illdefined inverse problem and is solved by applying statistics-based maximum likelihood (ML) type constraints. Here, the limits of classification accuracy attainable, using (in full) the professionally prepared GIS vector overlay for giving comprehensive training sets (approach a), and thereafter fortifying this further even by starting with a priori probability worked out using relative fractional distribution under each class (approach b), and improvement of accuracy in iterative mode were studied. Both GIS vector overlay-based approaches a and b gave nearly similar results for the area under different thematic classes and nearly matched with area(s) under input GIS overlay for dense, mixed and teak forest, grassland and water, and did not match for settlement, podu/blank, bamboo and semi-evergreen forests. In non-GIS based ML classification, near matching with GIS vector overlay was achieved in initial classification for dense forest, semi-evergreen forest, settlement and water. Overall accuracy and kappa coefficients were favouring GIS vector overlay-based technique giving overall accuracy in 83.5-87.5% range (and not 100%) while for non-GIS overlay approaches it was about 73%.
Bruzzone, L. and S.B. Serpico (2000). A technique for feature selection in multiclass problem. Intl. J. Rem Sens ., 21(3): 549-563.
Casals-Carrasco, P., Kubo, S. and B. Babu Madhvan (2000). Application of spectral mixture analysis for terrain evaluation studies. Intl. J. Rem. Sens., 21(16): 3039-3055.
Chuvieco, E. and R.G. Congalton (1988). Using cluster analysis to improve the selection of training statistics in classifying remotely sensed data. Photogramm. Engg. Rem. Sens., 54: 1275-1281.
Congalton, R.G. (1991). A review of assessing the accuracy of classifications of remotely sensed data. Rem. Sens. Environ ., 37: 35-46.
Duda, T. and M. Canty (2002). Unsupervised classification of satellite imagery: Choosing a good algorithm. Intl. J. Rem.Sens. , 23(11): 2193-2212.
Erol, H. (2000). A practical method for constructing the mixture model for a spectral class. Intl. J. Rem. Sens ., 21(4): 823-830.
Janssen, L., Jaarsma, M. and E. van der Linden (1990). Integrating Topographic data with remote sensing for land cover classification. Photogramm. Engg. Rem. Sens ., 56: 1503-1506.