Contribution Based on Neurons Networks for the Prediction of Greenhouse Gas Emissions in a Handling Port
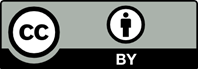
Greenhouse gases emitted by ships and port handling equipment contribute enormously to the climate change, which is why our reflection in this paper focusses on its quantification. This study is performed to predict the amount of greenhouse gases during the unloading and loading operations of ships at the quayside in a seaport. After developing a model whose resolution allowed us to obtain a solid database, we performed a simulation using the Levenberg-Marquart algorithm using artificial neural networks. The results allowed us to determine the performance of our machine-learning model.
Ademe (2022). Méthode pour la réalisation des bilans d’émissions de gaz à effet de serre Agence de la Transition Ecologique) Version 5, pp. 85-102.
Alexandre L., Luccioni, A., Schmidt, V. and T. Dandres (2019). Quantifying the carbon emissions of machine learning, arXiv preprint 1910.09700, pp. 2-4.
Alfredo, C., Culurciello, E. and A. Paszke (2017). An analysis of deep neural network models for practical applications, arXiv preprint 1605.07678, pp. 2-6.
Chaoyun, Z., Patras, P. and H. Haddadi (2019). Deep learning in mobile and wireless networking: A survey. IEEE Communications Surveys and Tutorials, 21(3): 2224-2287.
Gouvenal, E. and R. Lacoste (2014). The reduction of ship-based emissions: Aggregated impact on costs and emissions for North Europe-East Asia liner services. International Journal of Shipping and Transport Logistics, 6(2): 213-233.
Guillaume, B. (2023). Analyse et modélisation de l’impact environnemental du système d’information, Université de La Rochelle, pp. 47-70.
Ji-Bum C. and E.-S. Kim (2018). Public perception of energy transition in Korea: Nuclear power, climate change, and party preference. Energy Policy, 116: 137-144.
Marjorie D., et al. (2014). The reduction of ship-based emissions: Aggregated impact on costs and emissions for North Europe-East Asia liner services. International Journal of Shipping and Transport Logistics, 6(2): 213-233.
MELCCFP: Ministère de l’environnement, de la lutte contre les changements climatiques, de la faune et des parcs (2022). Guide de quantification des émissions de gaz à effet de serre, 2022, pp. 101-120.
Pascal, B. (2023). La technologie permettra d’éviter le réchauffement climatique, Cairn Info, Science humaine et sociale, pp. 51-53.
Raghnavendra, S., Lasse, F., Wolff A. and B. Kanding (2020). Carbontracker: Tracking and predicting the carbon footprint of training deep learning models. arXiv 03051, pp. 1-4.
Samah, T. (2023). Réduction des émissions de gaz à effet de serre des réseaux sans fil - Amélioration des performances des approches d’intelligence artificielle, Université du Québec en Outaouais, pp. 38-52.
Sara, B., Raffanel, D. and B. Beauzamy (2013). Vitesse des véhicules et émissions de CO2. Société de Calcul Mathématique SA Août 2013: 2-10.
Setyawati, D. (2020). Analysis of perceptions towards the rooftop photovoltaic solar system policy in Indonesia. Energy Policy, 144: 111569.
Stamoulis, D., Ermao Cai, E., Da-Cheng, J. and D. Marculescu (2018). Hyperpower: Power-and memory-constrained hyper-parameter optimization for neural networks in 2018 Design, Automation and Test in Europe Conference and Exhibition. IEEE, pp. 19-24.
Strubell, E., Ananya Ganesh, A. and A. Mccallum (2019). Energy and policy considerations for deep learning in NLP, arXiv preprint 1906.02243, pp. 1-5.
Ubeda, S., Arcelus, F.J. and J. Faulin (2011). Green logistics at Eroski: A case study. International Journal of Production Economics, 131: 44-51.
Wuisan, L. (2012). Greening international shipping through private governance: A case study of the Clean Shipping Project. Marine Policy, 36(1): 165-173.