Role of Artificial Intelligence in Modernisation of Fire Risk Management
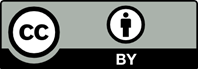
Fire in the form of wildfire, indoor fire, and bombardment, regardless of their natural or manmade origin, impacts substantially the economic as well as environmental hazards such as Air Pollution. This research aims to identify the role of artificial intelligence (AI) in modernising fire risk management. Using interpretive structural modeling (ISM) techniques, we can understand the interdependencies and hierarchical relationships within this context. AI enables the analysis of vast amounts of data from various sources, including historical fire incidents, weather patterns, building structures, and human behaviour, to assess and predict fire risks more accurately. ISM is a computational technique that uses a qualitative and interpretive approach to address intricate issues by mapping the relationships between variables and converting them into a multilevel structural model. Interpretive Structural Modeling (ISM) is a mathematical and qualitative tool used to identify key variables and create a hierarchical model that illustrates their interrelationships. Seven variables have been identified based on literature and expert input. Variables have been classified based on their influence and reliance.
Al-Taai, S.H.H. and W.A.M. al-Dulaimi (2022). Air pollution: A study of its concept, causes, sources and effects. Asian Journal of Water, Environment and Pollution, 19: 17-22.
Bazargan, S. et al. (2022). Analysis of the performance of cutting tools of tunnel boring machine (TBM) in silty-sand soils using artificial neural network (ANN) – Case Study: Tabriz Metro Line 2 Project. Asian Journal of Water, Environment and Pollution, 19: 71-78.
Bullock, J.B. (2019). Artificial intelligence, discretion, and bureaucracy. The American Review of Public Administration, 49: 751-761.
Feng, S., Zhenning Li, Z. and X. Sun (2016). Analysis of bus fires using interpretative structural modelling. Journal of Public Transportation, 19: 1-18.
Guan, C. et al. (2022). Hierarchical structure model of safety risk factors in new coastal towns: a systematic analysis using the DEMATEL-ISM-SNA method. International Journal of Environmental Research and Public Health, 19: 10496.
Hodges, J.L., Lattimer, B.Y. and V.L. Champlin (2022). The Role of Artificial Intelligence in Firefighting. In: Handbook of Cognitive and Autonomous Systems for Fire Resilient Infrastructures. Cham: Springer International Publishing, pp. 177-203.
Kim, Y.-K. et al. (2018). Disaster theory. In: Disaster risk management in the Republic of Korea, pp: 23-76.
Knyziak, P., Kowalski, R. and J.R. Krentowski (2019). Fire damage of RC slab structure of a shopping center. Engineering Failure Analysis, 97: 53-60.
Maraveas, Chrysanthos et al. (2021). Applications of artificial intelligence in fire safety of agricultural structures. Applied Sciences, 11: 7716.
Park, J.H. et al. (2019). Dependable fire detection system with multifunctional artificial intelligence framework. Sensors, 19: 2025.
Qian, Y. and H. Wang (2023). Vulnerability assessment for port logistics system based on DEMATEL-ISM-BWM. Systems, 11: 567.
Renganath, K. and M. Suresh (2016). Analyzing the drivers for safety practices using interpretive structural modeling: A case of Indian manufacturing firms. In: 2016 IEEE international conference on computational intelligence and computing research (ICCIC). IEEE.
Siraj, Md Tanvir, et al. (2023). Analysis of the fire risks and mitigation approaches in the apparel manufacturing industry: Implications toward operational safety and sustainability. Heliyon, 9: 9.
Sun, W., Bocchini, P. and B.D. Davison (2020). Applications of artificial intelligence for disaster management. Natural Hazards, 103: 2631-2689.
Wang, B. and Y. Wang (2021). Big data in safety management: An overview. Safety Science, 143: 105414.
Wu, Xiqiang et al. (2022). A real-time forecast of tunnel fire based on numerical database and artificial intelligence. Building Simulation, 15: 511-524.
Xu, W., Chan, S.C. and W.Y. Leong (2023). Effectiveness study of artificial intelligent facility system in maintaining building fire safety (case study: typical public building cases of fire-fighting facilities management in China). Discrete Dynamics in Nature and Society, 2023: 2592322.
Zhang, Shuo et al. (2022). Design of intelligent fire-fighting robot based on multi-sensor fusion and experimental study on fire scene patrol. Robotics and Autonomous Systems, 154: 104122.