Water Quality Assessment using Machine Learning: A Focus on Coliform Prediction in Water
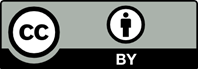
Water quality assessment is essential for safeguarding public health and protecting water resources. This study focused on predicting water quality, specifically the presence of total coliforms, using various machine-learning techniques. The present study utilises a publicly available dataset encompassing the geographical area of India consisting of various physical water quality parameters. Various regression techniques were applied to the dataset after appropriate pre-processing including feature selection and normalisation. The findings demonstrate that gradient boosting regression outperforms other methods, achieving high accuracy with mean absolute error (MAE) of 0.0349, mean squared error (MSE) of 0.0038, and root mean squared error (RMSE) of 0.0620. Conductivity and temperature emerged as the most influential factors in total coliform prediction, as revealed by feature importance analysis. These results contribute to water quality understanding, aiding water resource management for public health protection. By accurately predicting total coliform presence, proactive measures can be taken timely to mitigate and minimise health risks associated with microbial contamination.
Avigliano, E. and N.F. Schenone (2015). Human health risk assessment and environmental distribution of trace elements, glyphosate, fecal coliform and total coliform in Atlantic Rainforest Mountain rivers (South America). Microchemical Journal, 122: 149-158. Doi: https://doi.org/10.1016/j.microc.2015.05.004
Benos, L., Tagarakis, A.C., Dolias, G., Berruto, R., Kateris, D. and D. Bochtis (2021). Machine learning in agriculture: A comprehensive updated review. Sensors, 21(11): 3758. doi: 10.3390/s21113758
Bui, D.T., Khosravi, K., Tiefenbacher, J., Nguyen, H. and N. Kazakis (2020). Improving prediction of water quality indices using novel hybrid machine-learning algorithms. Science of the Total Environment, 721: 137612. doi: https://doi.org/10.1016/j.scitotenv.2020.137612
Di Nunno, F., Zhu, S., Ptak, M., Sojka, M. and F. Granata (2023). A stacked machine learning model for multi-step ahead prediction of lake surface water temperature. Science of The Total Environment, 890: 164323. doi: https://doi. org/10.1016/j.scitotenv.2023.164323
Gafri, H.F., Zuki, F.M., Aroua, M.K. and M.M. Bello (2019). Enhancing the anti-biofouling properties of polyethersulfone membrane using chitosan-powder activated carbon composite. Journal of Polymers and the Environment, 27: 2156-2166. Doi: 10.1007/s10924-019- 01505-z
Jasti, V.D.P., Kumar, G.K., Kumar, M.S., Maheshwari, V., Jayagopal, P., Pant, B., Karthick, A. and M. Muhibbullah (2022). Relevant-based feature ranking (RBFR) method for text classification based on machine learning algorithm. Journal of Nanomaterials, 2022(1): 1-12. Doi: 10.1155/2022/9238968 Kadyan, S.,
Kumar, N., Lawaniya, R., Sharma, P.K., Arora, B. and N. Tehri (2020). Rapid and miniaturized method for detection of hygiene indicators, Escherichia coli and coliforms, in dairy products. Journal of Food Safety, 40(5): 12839 doi: 10.1111/jfs.12839
Kaggle Datasets. Available at: https://www.kaggle.com/ datasets
Kang, J.K., Lee, D., Muambo, K.E., Choi, J.W. and J.E. Oh (2023). Development of an embedded molecular structurebased model for prediction of micropollutant treatability in a drinking water treatment plant by machine learning from three years monitoring data. Water Research, 239: 120037. https://doi.org/10.1016/j.watres.2023.120037
Kaur, I. and N. Kapoor (2016). Token based approach for cross project prediction of fault prone modules. In: 2016 International Conference on Computational Techniques in Information and Communication Technologies (ICCTICT), New Delhi, India, pp. 215-221, doi: 10.1109/ ICCTICT.2016.7514581.
Kaur, I., Narula, G.S. and V. Jain (2017). Differential analysis of token metric and object oriented metrics for fault prediction. International Journal of Information Technology, 9: 93-100. doi: 10.1007/s41870-017-0004-0
Liu, G., Tian, S., Xu, G., Zhang, C. and M. Cai (2023). Combination of effective color information and machine learning for rapid prediction of soil water content. Journal of Rock Mechanics and Geotechnical Engineering, 15(9): 2441-2457. doi: https://doi.org/10.1016/j. jrmge.2022.12.029
Liu, M. and J. Lu (2014). Support vector machine―an alternative to artificial neuron network for water quality forecasting in an agricultural nonpoint source polluted river? Environmental Science and Pollution Research, 21: 11036-11053. doi: 10.1007/s11356-014-3046-x
Madhav, S., Ahamad, A., Singh, A.K., Kushawaha, J., Chauhan, J.S., Sharma, S. and P. Singh (2020). Water Pollutants: Sources and Impact on the Environment and Human Health. In: Pooja, D., Kumar, P., Singh, P. and S. Patil (eds). Sensors in Water Pollutants Monitoring: Role of Material. Advanced Functional Materials and Sensors. Springer, Singapore. https://doi.org/10.1007/978-981-15- 0671-0_4
Misaghi, F., Delgosha, F., Razzaghmanesh, M. and B. Myers (2017). Introducing a water quality index for assessing water for irrigation purposes: A case study of the Ghezel Ozan River. Science of the Total Environment, 589: 107- 116. https://doi.org/10.1016/j.scitotenv.2017.02.226
Mittelmann, A.S., Ron, E.Z. and J. Rishpon (2002). Amperometric quantification of total coliforms and specific detection of Escherichia coli. Analytical Chemistry, 74(4): 903-907. doi: 10.1021/ac0156215
Pesce, S.F. and D.A. Wunderlin (2000). Use of water quality indices to verify the impact of Córdoba City (Argentina) on Suquıa River. Water Research, 34(11): 2915-2926. https://doi.org/10.1016/S0043-1354(00)00036-1
Shekoohiyan, S., Hadadian, M., Heidari, M. and H. Hosseinzadeh-Bandbafha (2023). Life cycle assessment of Tehran municipal solid waste during the COVID-19 pandemic and environmental impacts prediction using machine learning. Case Studies in Chemical and Environmental Engineering, 7: 100331. https://doi. org/10.1016/j.cscee.2023.100331
Singh, P. and D.P. Singh (2023). Comparative Analysis of Machine Learning Classifiers for Heart Disease Prediction in Cloud Environment. In: 2023 10th International Conference on Computing for Sustainable Global Development (INDIACom), New Delhi, India, pp. 552- 556.
Tomić, A.Š., Antanasijević, D., Ristić, M., Perić-Grujić, A. and V. Pocajt (2018). A linear and non-linear polynomial neural network modeling of dissolved oxygen content in surface water: Inter-and extrapolation performance with inputs’ significance analysis. Science of the Total Environment, 610-618: 1038-1046. https://doi. org/10.1016/j.scitotenv.2017.08.192
Tripathi, M. and S.K. Singal (2019). Use of principal component analysis for parameter selection for development of a novel water quality index: A case study of river Ganga India. Ecological Indicators, 96(1): 430-436. https://doi. org/10.1016/j.ecolind.2018.09.025
Wu, Z., Lai, X. and K. Li (2021). Water quality assessment of rivers in Lake Chaohu Basin (China) using water quality index. Ecological Indicators, 121: 107021. doi: https:// doi.org/10.1016/j.ecolind.2020.107021
Wu, Z., Wang, X., Chen, Y., Cai, Y. and J. Deng (2018). Assessing river water quality using water quality index in Lake Taihu Basin, China. Science of the Total Environment, 612: 914-922. https://doi.org/10.1016/j. scitotenv.2017.08.293
Zidan, K., Sbahi, S., Hejjaj, A., Ouazzani, N., Assabbane, A. and L. Mandi (2022). Removal of bacterial indicators in on-site two-stage multi-soil-layering plant under arid climate (Morocco): Prediction of total coliform content using K-nearest neighbor algorithm, Environmental Science and Pollution Research, 29: 75716-75729. Doi: 10.1007/s11356-022-21194-x