Multi Kernel Support Vector Machine for Particulate Matter Estimation
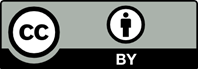
The presence of particulate matter, in the atmospheric environment, affects the health of living creatures as well as the ecosystem. Estimation of particulate matter has become one of the most challenging study for researchers. There are numerous computer techniques for the estimation of these particles. In this study, a multi kernel support vector machine (M-SVM) approach is introduced for the categorisation of particulate matter captured as digital images. Images from the archive of many outdoor scene (AMOS) have been taken for implementation purpose. The model is trained to predict the level of particulate matter captured as a digital image. An experimental model with M-SVM classification predicts the particulate matter captured as image among three levels, i.e., whether an image has a normal level, critical level or highly critical level. Simulated results were found to analyse the particulate matter with 98% of accuracy, which ensures efficient recognition of our experimental method
Aiolli, F. and A. Sperduti (2005). Multiclass classification with multi-prototype support vector machines. Journal of Machine Learning Research. 6: 817-850.
Al-Yaseen, W.L., Zulaiha A.O. and M.Z.A. Nazri (2017). Multi-level hybrid support vector machine and extreme learning machine based on modified K-Means for intrusion detection system. Expert Systems with Applications, 67: 296-303.
Azid, A., et al. (2018). Assessing indoor air quality using chemometric models. Polish Journal of Environmental Studies, 27(6): 2443-2450.
Batuwita, R. and V. Palade (2013). Class Imbalance Learning Methods for Support Vector Machines. In: Imbalanced Learning: Foundations, Algorithms and Applications. Chapter 5, IEEE, pp. 83-99.
Bo, Q., et al. (2018). Particle Pollution Estimation from Images Using Convolutional Neural Network and Weather Features. In: Proceedings - International Conference on Image Processing, ICIP, pp. 3433-3437.
Chen, X., Yaru L. and D. Li (2016). An efficient method for air quality evaluation via ANN-based image recognition. Presented at the 2nd International Conference on Artificial Intelligence and Industrial Engineering (AIIE2016). Advances in Intelligent System Research, 133: 253-256.
Dong, C., Loy, C.C., He, K. and X. Tang (2016). Image super-resolution using deep convolutional networks. IEEE Transactions on Pattern Analysis and Machine Intelligence, 38(2): 295-307.
Farahmand, A., Abdolhossein S. and J. Shanbehzadeh (2013). Document Image Noises and Removal Methods. Proceedings of the International Multi-Conference of Engineers and Computer Scientists 2013 (IMECS 2013), Hong Kong, Vol. I.
Gaur, D., Mehrotra, D. and K. Singh (2020). Particulate Matter Estimation Based on Haze Degree Factor. In: Pant, M., Sharma, T., Verma, O., Singla, R. and A. Sikander (eds). Soft Computing: Theories and Applications. Advances in Intelligent Systems and Computing, 1053: 769-779. Springer, Singapore. https://doi.org/10.1007/978- 981-15-0751-9_70
Gu, K., Qiao, J. and X. Li (2019). Highly efficient picture-based prediction of PM2.5 concentration. IEEE Transactions on Industrial Electronics, 6(4): 3176-3184.
He, K., Sun, J. and X. Tang (2013). Guided image filtering. IEEE Transactions on Pattern Analysis and Machine Intelligence, 35(6): 1397-1409.Hsu, Chih-Wei, Chih-Chung Chang and Chih-Jen Lin (2003). A practical guide to support vector classification. pp. 1396- 1400. http://www.datascienceassn.org
Kim, S.H., et al. (2019). Vision-based deep Q-learning network models to predict particulate matter concentration levels using temporal digital image data. Journal of Sensors, 2019: 9673047.
Liu, C., Tsow, F., Zou, Y. and N. Tao (2016). Particle pollution estimation based on image analysis. PLoS ONE, 11(2): e0145955.
O’Sullivan, J.D., Stylianou, A., Abrams, A. and R. Pless (2015). Democratizing the Visualization of 500 Million Webcam Images. In: Proceedings - Applied Imagery Pattern Recognition Workshop, pp. 1-5.
Peiris, R.H., Budman, H., Moresoli, C. and R.L. Legge (2010). Understanding fouling behaviour of ultrafiltration membrane processes and natural water using principal component analysis of fluorescence excitation-emission matrices. Journal of Membrane Science, 357(1-2): 62-72.
Pharr, M. and G. Humphreys (2004). Sampling and Reconstruction. In: Physically Based Rendering: From Theory to Implementation. Chapter 7. Morgan Kauffmann Publishers.
Rebentrost, P., Masoud, M. and S. Lloyd (2014). Quantum support vector machine for big data classification. Physical Review Letters, 113: 130503.
Rijal, N., et al. (2018). Ensemble of Deep Neural Networks for Estimating Particulate Matter from Images. In: 2018 3rd IEEE International Conference on Image, Vision and Computing, ICIVC 2018. pp. 733-738.
Shad, R., Mesgari, M.S., Abkar, A. and A. Shad (2009). Predicting air pollution using fuzzy genetic linear membership kriging in GIS. Computers, Environment and Urban Systems, 33(6): 472-481.
Smith, K., et al. (2015). CIDRE: An illumination-correction method for optical microscopy. Nature Methods, 12(5): 404-406.
Thurston, G.D., Ito, K. and R. Lall (2011). A source apportionment of U.S. fine particulate matter air pollution. Atmospheric Environment, 45(24): 3924-3946.
Troscianko, J. and M. Stevens (2015). Image calibration and analysis toolbox -A free software suite for objectively measuring reflectance, colour and pattern. Methods in Ecology and Evolution, 6(11): 1320-1331.
Wang, Z. and X. Xue (2013). Multi-Class Support Vector Machine. In: Support Vector Machines Applications, pp. 23-48.
Weizhen, H., et al. (2014). Using support vector regression to predict PM 10 and PM 2.5. In IOP Conference Series: Earth and Environmental Science, 17: 012268.
Zhao, Y. and Y. Abu (2013). Fine particulate matter concentration level prediction by using tree-based ensemble classification algorithms. International Journal of Advanced Computer Science and Applications, 4(5): 21-27.
Zaman, N.A.F.K., Kanniah, K.D. and D.G. Kaskaoutis (2017). Estimating particulate matter using satellite based aerosol optical depth and meteorological variables in Malaysia. Atmospheric Research, 193: 142-162.