Creating a Soil Map Using Digital Soil Mapping on the Example of the Diurtiulinsky Municipal District
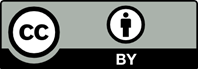
The study describes the use of machine learning methods, geostatistics, etc. in establishing soil properties depending on various classes of soil. The most commonly used data are information from spectral reflectance bands of satellite images and terrain models. Besides, there is also great potential for creating new data tiers. The study relies on a method known as SCORPAN-SSPFe, which assumes spatial error autocorrelation as a standalone function. This method is actively used in places where there is not enough information about soil data. Besides, four types of interpolation were compared using the SCORPAN method: multiple linear regression, cubistic model, cubistic model with kriging and random forest model, which use extensive but common values of soil properties associated with soil classes. The research result is obtained by applying the method to conduct large-scale soil surveys, which determines the purpose and relevance of our study
Arnold, R.W. (2006). Soil survey and soil classification. Environmental soil-landscape modeling: Geographic information technologies and pedometrics. Taylor & Francis Group, Boca Raton, FL, 37-60 pp.
Boettinger, J.L., Howell, D.W., Moore, A.C., Hartemink, A.E. and S. Kienast-Brown (Eds.). (2010). Digital soil mapping: Bridging research, environmental application, and operation. Springer Science & Business Media.
Chai, H., Rao, S., Wang, R., Liu, J., Huang, Q. and X. Mou (2015). The effect of the geomorphologic type as surrogate to the time factor on digital soil mapping. Open Journal of Soil Science, 5(06): 123.
Coca-Castro, A., Gutierrez-Díaz, J.S., Camacho, V., López, A.F., Escudero, P., Serrato, P.K. and J. González (2021). Optimized data-driven pipeline for digital mapping of quantitative and categorical properties of soils in Colombia. Revista Brasileira de Ciência do Solo, 45: e0210084.
Hewitt, A., Dominati, E., Webb, T. and T. Cuthill (2015). Soil natural capital quantification by the stock adequacy method. Geoderma, 241: 107-114.
Iaparov, I.M. (2005). Atlas of the Republic of Bashkortostan. Kitap, Ufa. Kaladhar, D.S.V.G.K., Pottumuthu, B.K., Rao, P.V.N., Vadlamudi, V., Chaitanya, A.K. and R.H. Reddy (2013). The elements of statistical learning in colon cancer datasets: Data mining, inference and prediction. Algorithms Research, 2(1): 8-17.
Kempen, B., Yigini, Y., Viatkin, K., de Jesus, J. M., de Sousa, L.M., van den Bosch, R. and R. Vargas (2019, January). The Global Soil Information System (GloSIS)–concept and design. In: Geophysical Research Abstracts (Vol. 21).
Kuhn, M., Wing, J., Weston, S., Williams, A., Keefer, C., Engelhardt, A., Cooper, T., Mayer, Z., Kenkel, B., Benesty, M., Lescarbeau, R., Ziem, A., Scrucca, L., Tang, Y. and C. Candan (2016). Caret: Classification and regression training. R Core Team. Available from: http://www.Rproject.org/.
Malone, B.P., Odgers, N.P., Stockmann, U., Minasny, B. and A. McBratney (2018). Digital mapping of soil classes and continuous soil properties. In: Pedometrics (pp. 373-413). Springer, Cham.
Minasny, B., McBratney, A.B., Malone, B.P. and I. Wheeler (2013). Digital mapping of soil carbon. Advances in Agronomy, 118: 1-47
Steinbuch, L., Orton, T. and D. Brus (2019). Model-based geostatistics from a Bayesian perspective: Investigating area-to-point kriging with small data sets. Mathematical Geosciences, 52: 1-27.
Odgers, N.P., McBratney, A.B. and B. Minasny (2015). Digital soil property mapping and uncertainty estimation using soil class probability rasters. Geoderma, 237: 190- 198
Webster, R. and M.A. Oliver (2013). Geostatistics for Environmental Scientists, second edition. John Wiley & Sons, Chichester.
Webster, R. and M.A. Oliver (2016). Modeling spatial variation of soil as random functions. In: Environmental soil–landscape modeling: Geographic information technologies and pedometrics. CRC Press, Boca Raton, FL, 241-289 pp.
Zhang, G.L., Feng, L.I.U. and X.D. Song (2017). Recent progress and future prospect of digital soil mapping: A review. Journal of Integrative Agriculture, 16(12): 2871- 2885.