Modeling the Effect of Magnesia Nanoparticles on CO Hydrogenation to Light Olefins in a Continuous Flow Reactor Using Fine Gaussian Support Vector Machine
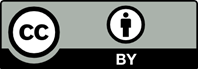
The effect of utilising energy derived from fossil sources on the environment has aroused research interest in alternative and sustainable energy sources. Synthesis gas, a mixture of carbon monoxide (CO) and hydrogen can be used as starting materials in hydrogenation reactions to produce chemical intermediates that can be used in various processes. This study investigates the robustness of applying a fine Gaussian support vector machine algorithm for predicting light olefins from catalytic CO hydrogenation using magnesia nanoparticlesbased catalysts. The datasets obtained from the CO hydrogenation reaction consist of input parameters such as magnesia nanoparticles contents, reaction temperature, and reactor pressure, and the output parameters which include CO conversions and the selectivity of light olefins (CH4, C2H6, C2H4 C3H8, C4H8, and C3H6). The dataset was trained and employed for the prediction of the light olefins using a support vector machine with an inbuilt Fine Gaussian Kernel function. The performance of the support vector machine was evaluated using the coefficient of determination (R2 ), root mean squared error (RMSE), mean square error (MSE), and the mean absolute error (MAE). The support vector machine showed significant potential in the prediction of CO conversion, CH4 selectivity, C2H6 selectivity, and C2H4 selectivity as indicated by R2 of 0.770, 0.800, 0.730, and 0.930, respectively. While less predictive performance was obtained for the prediction of C3H8 selectivity, C4H8 selectivity and C3H6 selectivity as indicated by R2 of 0.630, 0.610, and 0.320, respectively.
Atashi, H., Shiva, M., Farshchi Tabrizi, F. and A.A. Mirzaei (2013). Study of syngas conversion to light olefins by response surface methodology. Journal of Chemistry, 2013. https://doi.org/10.1155/2013/945735
Azapagic, A., Stamford, L., Youds, L. and C. BarteczkoHibbert (2016). Towards sustainable production and consumption: A novel DEcision-Support Framework IntegRating Economic, Environmental and Social Sustainability (DESIRES). Computers & Chemical Engineering, 91: 93-103. https://doi.org/https://doi. org/10.1016/j.compchemeng.2016.03.017
Bai, Y., Sun, Z., Zeng, B., Long, J., Li, L., de Oliveira, J.V. and C. Li (2019). A comparison of dimension reduction techniques for support vector machine modeling of multiparameter manufacturing quality prediction. Journal of Intelligent Manufacturing, 30(5): 2245-2256. https://doi. org/10.1007/s10845-017-1388-1
Corma, A., Corresa, E., Mathieu, Y., Sauvanaud, L., AlBogami, S., Al-Ghrami, M. S. and A. Bourane (2017). Crude oil to chemicals: Light olefins from crude oil. Catalysis Science and Technology, 7(1): 12-46. https:// doi.org/10.1039/c6cy01886f
Fan, J., Wu, L., Zhang, F., Cai, H., Wang, X., Lu, X. and Y. Xiang (2018). Evaluating the effect of air pollution on global and diffuse solar radiation prediction using support vector machine modeling based on sunshine duration and air temperature. Renewable and Sustainable Energy Reviews, 94: 732-747. https://doi.org/https://doi. org/10.1016/j.rser.2018.06.029
Ji, X., Shang, X., Dahlgren, R.A. and M. Zhang (2017). Prediction of dissolved oxygen concentration in hypoxic river systems using support vector machine: a case study of Wen-Rui Tang River, China. Environmental Science and Pollution Research, 24(19): 16062-16076. https://doi. org/10.1007/s11356-017-9243-7
Leong, W.C., Bahadori, A., Zhang, J. and Z. Ahmad (2021). Prediction of water quality index (WQI) using support vector machine (SVM) and least square-support vector machine (LS-SVM). International Journal of River BasinManagement, 19(2): 149-156. https://doi.org/10.1080/157 15124.2019.1628030
Mohammadi, N., Samimi, A., Antalovits, F., Niedzwiecki, L. and R. Mesbah (2021). Optimization of operating conditions for CO hydrogenation to hydrocarbon via response surface method. Chemical Methodologies, 5: 178-189. https://doi.org/10.22034/chemm.2021.122477
Pedersen, E.Ø., Svenum, I.-H. and E.A. Blekkan (2018). Mn promoted Co catalysts for Fischer-Tropsch production of light olefins – An experimental and theoretical study. Journal of Catalysis, 361: 23-32. https://doi.org/https:// doi.org/10.1016/j.jcat.2018.02.011
Rădulescu, M., Rădulescu, S. and C.Z. Rădulescu (2009). Sustainable production technologies which take into account environmental constraints. European Journal of Operational Research, 193(3): 730-740. https://doi.org/ https://doi.org/10.1016/j.ejor.2007.05.057
Shi, B., Zhang, Z., Liu, Y., Su, J., Liu, X., Li, X., Wang, J., Zhu, M., Yang, Z., Xu, J. and Y.-F. Han (2020). Promotional effect of Mn-doping on the structure and performance of spinel ferrite microspheres for CO hydrogenation. Journal of Catalysis, 381: 150-162. https:// doi.org/https://doi.org/10.1016/j.jcat.2019.10.034
Wang, Y., Zhao, Y., Liu, J., Li, Z., Waterhouse, G.I.N., Shi, R., Wen, X. and T. Zhang (2020). Manganese oxide modifiednickel catalysts for photothermal CO hydrogenation to light olefins. Advanced Energy Materials, 10(5): 1-9. https://doi.org/10.1002/aenm.201902860
Yang, Z., Zhang, Z., Liu, Y., Ding, X., Zhang, J., Xu, J. and Y. Han (2021). Tuning direct CO hydrogenation reaction over Fe-Mn bimetallic catalysts toward light olefins: Effects of Mn promotion. Applied Catalysis B: Environmental, 285(November 2020): 119815. https://doi.org/10.1016/j. apcatb.2020.119815
Yuan, X., Tan, Q., Lei, X., Yuan, Y. and X. Wu (2017). Wind power prediction using hybrid autoregressive fractionally integrated moving average and least square support vector machine. Energy, 129: 122-137. https://doi.org/10.1016/j. energy.2017.04.094
Zendehboudi, A., Baseer, M.A. and R. Saidur (2018). Application of support vector machine models for forecasting solar and wind energy resources: A review. Journal of Cleaner Production, 199: 272-285. https://doi. org/https://doi.org/10.1016/j.jclepro.2018.07.164
Zhu, Y., Pan, X., Jiao, F., Li, J., Yang, J., Ding, M., Han, Y., Liu, Z. and X. Bao (2017). Role of manganese oxide in syngas conversion to light olefins. ACS Catalysis, 7(4): 2800-2804. https://doi.org/10.1021/acscatal.7b00221