Clustering of Groundwater Wells and Spatial Variation of Groundwater Recharge in Sina Basin, India
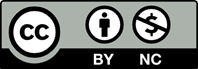
A spatial and temporal analysis of groundwater levels, topography, and precipitation is required to properly manage the groundwater resource. The present paper explains it in two parts: (1) spatial analysis of groundwater levels and selection of suitable clustering approach for selection of representative wells and (2) spatial and temporal variations of groundwater recharge calculated by three numerical models: Chaturvedi model, Amritsar model and ERAS model. Four clustering techniques including K-Means clustering algorithm, Hierarchical clustering technique, canopy and expectation maximisation (EM) were used for the clustering of groundwater levels. Among these, the canopy technique presents more reliable results compared to the other techniques for the spatial analysis of groundwater levels and the formation of representative wells in the Sina basin. For the groundwater recharge estimation, Chaturvedi model and ERAS model values were found very close. The recharge values show consistency with the precipitation data and found that 15% of precipitation contributes to annual groundwater recharge. Spatio-temporal variation of groundwater recharge correlated with precipitation is also carried out for the selected basin. The results show a drastic decline in the groundwater recharge from the year 1990 to 2008. An empirical expression is also developed for groundwater recharge estimation in terms of groundwater level. This provides regional scale information about the basin and helps to understand the groundwater exploitation scenario for instance.
Arthur, D. and S. Vassilvitskii (2007). K-means++: The advantages of careful seeding. In: 2007 ACM-SIAM symposium on discrete algorithms (SODA’07).
Bartoletti, N., Casagli, F., Marsili-Libelli, S., Nardi, A. and L. Palandri (2018). Data-driven rainfall/runoff modelling based on a neuro-fuzzy inference system. Environmental Modelling & Software, 106: 35-47.
Boughariou, E., Saidi, S., Barkaoui, A.E., Khanfir, H., Zarehloul, Y. and S. Bouri (2014). Mapping recharge potential zones and natural recharge calculation: Study case in Sfax region. Arabian Journal of Geosciences, 8: 5203-5221.
Bouwer, H. (2002). Artificial recharge of groundwater: Hydrogeology and engineering. Hydrogeology Journal,
10: 121-142.
Chaturvedi, R.S. (1973). A note on the investigation of ground water resources in western districts of Uttar Pradesh. In: Annual Report. U.P. Irrigation Research Institute, 86-122.
Gemitzi, A., Ajami, H. and H.H. Richnow (2017). Developing empirical monthly groundwater recharge equations based on modeling and remote sensing data – Modeling future groundwater recharge to predict potential climate change impacts. Journal of Hydrology, 546: 1-13.
Hall, M., Frank, E., Holmes, G.P., Fahringer, B., Reutemann, P. and I.H. Witten (2009). The WEKA Data Mining Software: An Update. SIGKDD Explorations 11.
Han, J. and M. Kamber (2006). Data Mining Concepts and Techniques. Morgan Kaufmann Publishers.
Krishnamurthy, J., Venkatesesa, Kumar, N., Jayraman, V. and M. Manivel (1996). An approach to demarcate ground water potential zones through remote sensing and a geographical information system. International Journal of Remote Sensing, 7: 1867-1884.
McCallum, A., Nigam, K. and L.H. Ungar (2000). Efficient clustering of high dimensional data sets with application to reference matching. In: Proceedings of the Sixth ACM SIGKDD International Conference on Knowledge Discovery and Data Mining ACM-SIAM Symposium on Discrete Algorithms. 169-178.
Murthy, K.S.R. (2000). Groundwater potential in a semi-arid region of Andhra Pradesh—A geographical information system approach. International Journal of Remote Sensing,21: 1867-1884.
Niraula, R., Meixner, T., Ajami, H., Rodell, M., Gochis, D. and C.L. Castro (2017). Comparing potential recharge estimates from three Land Surface Models across the western US. Journal of Hydrology, 545: 410-423.
Roshni, T., Jha, M.K., Deo, R.C. and A. Vandana (2019). Development and evaluation of hybrid artificial neural network architectures for modeling spatio-temporal groundwater fluctuations in a complex aquifer system. Water Resources Management, 33: 2381-2397.
Sahoo, S. and M.K. Jha (2016). Pattern recognition in lithology classification: Modeling using neural networks, self-organizing maps and genetic algorithms. Hydrogeology Journal, 25: 311-330.
Sehgal, S.R. (1973). Ground Water Resources of Punjab State– A Recent Study. Third Annual Research Session. Central Board of Irrigation and Power, New Delhi.
Selvam, S., Magesh, N.S., Chidambaram, S., Rajamanickam, M. and M.C. Sashikkuma (2014). A GIS-based identification of groundwater recharge potential zones using RS and IF technique: A case study in Ottapidaram taluk, Tuticorin district, Tamil Nadu. Environmental Earth Science, 73: 3785-3799.
Wable, P.S., Jha, M.K. and S. Murasingh (2017). Evaluation of groundwater resources for sustainable groundwater development in a semiarid river basin of India. Environmental Earth Science, 76: 601. DOI 10.1007/ s12665-017-6912-2
Written, I.H. and E. Frank (2005). Data Mining: Practical Machine Learning Tools and Techniques. Morgan Kaufmann Publication.