Renewable Energy Sources for Clean Environment: Opinion Mining
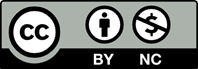
Use of conventional energy sources causes enormous amount of pollution leading to the problem of global warming. Most of the countries in Asia are facing the problem of pollution leading to lots of health problems. To curb these problems, renewable energy sources play a very important role. Sentiment analysis is used to analyze opinions of the user for decision making with the help of natural language processing techniques. Opinions are the sentiment or emotions about a subject that is expressed by a user. To study about the emotions of people about alternate energy sources we have carried out comparative sentiment analysis on various renewable energy sources using Twitter data. In our paper we have considered five sources namely: Solar energy, Bioenergy, Wind power, Hydro power and Geothermal energy. Data has been collected from Twitter which is approximately 20,000 tweets for each energy source amounting approximately to 100,000 data which have been considered for analysis. Eight sentiments are calculated for each renewable energy source. It has been found that the people’s opinion about renewable energy sources—mainly solar and wind energy—fetches most tweets and people are more positive towards renewable energy sources for better environment.
Crannell, W.C., Clark, E., Jones, C., James, T.A. and J. Moore (2016). A pattern-matched Twitter analysis of US cancer-patient sentiments. Journal of Surgical Research, 206(2): 536-542.
Injadat, M., Salo, F. and A.B. Nassif (2016). Data mining techniques in social media: A survey. Neurocomputing, 214: 654-670.
Kunz, N.C., Kastelle, T. and C.J. Moran (2017). Social network analysis reveals that communication gaps mayprevent effective water management in the mining sector. Journal of Cleaner Production, 148: 915-922.
Manek, A.S., Shenoy, P.D., Mohan, M.C. and K.R. Venugopal (2017). Aspect term extraction for sentiment analysis in large movie reviews using Gini Index feature selection method and SVM classifier. World wide web, 20(2): 135-154.
Medhat, W., Hassan, A. and H. Korashy (2014). Sentiment analysis algorithms and applications: A survey. Ain Shams Engineering Journal, 5(4): 1093-1113.
Óskarsdóttir, M., Bravo, C., Verbeke, W., Sarraute, C., Baesens, B. and J. Vanthienen (2016). A comparative study of social network classifiers for predicting churn in the telecommunication industry. IEEE/ACM International Conference on Advances in Social Networks Analysis and Mining (ASONAM), 2016: 1151-1158.
Pang, B. and L. Lee (2008). Opinion mining and sentiment analysis. Foundations and Trends® in Information Retrieval, 2(1–2): 1-135.
Pill, S., Harvey, S. and B. Hyndman (2017). Novel research approaches to gauge global teacher familiarity with gamebased teaching in physical education: An exploratory# Twitter analysis. Asia-Pacific Journal of Health, Sport and Physical Education, 8(2): 161-178.
Renewable Energy Statistics – Accessed from http:// ec.europa.eu/eurostat/statistics-explained/index.php/ Renewable_energy_statistics
Taboada, M., Brooke, J., Tofiloski, M., Voll, K. and M. Stede (2011). Lexicon-based methods for sentiment analysis. Computational Linguistics, 37(2): 267-307.
Tang, J., Chang, Y., Aggarwal, C. and H. Liu (2016). A survey of signed network mining in social media. ACM Computing Surveys (CSUR), 49(3): 42.
Tayal, D.K. and S.K. Yadav (2017). Sentiment analysis on social campaign “Swachh Bharat Abhiyan” using unigram method. AI & Society, 32(4): 633-645.