Prediction of the Penetration Rate and Number of Consumed Disc Cutters of Tunnel Boring Machines (TBMs) Using Artificial Neural Network (ANN) and Support Vector Machine (SVM)—Case Study: Beheshtabad Water Conveyance Tunnel in Iran
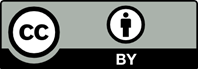
Tunnel boring machines (TBMs) are designed to excavate underground spaces and widely used in tunneling, civil and mining projects. TBM performance prediction substantially deals with the evaluation of machine’s penetration rate and the number of consumed disc cutters. There are various methods and equations to predict the TBMs performance in the literature. In this paper, we predicted the penetration rate and number of consumed disc cutters in Beheshtabad water conveyance tunneling project, one of the major water conveyance tunneling projects in Iran, using Artificial Neural Network (ANN) and Support Vector Machine (SVM) methods. Results showed that both approaches are very effective but SVM yields more precise and realistic findings than ANN.
Ahmadi, A., Zolfagharipoor, M.A. and A.A. Afzali (2018). Stability Analysis of Stakeholders’ Cooperation in InterBasin Water Transfer Projects: A Case Study. Water Resour Manage. https://doi.org/10.1007/s11269-018-2065-7
Alsahly, A., Stascheit, J. and G. Meschke (2016). Advanced finite element modeling of excavation and advancement processes in mechanized tunneling. Adv Eng Softw, 100: 198-214. https://doi.org/10.1016/j.advengsoft. 2016.07.011
Bagherpour, R. and M. Rahimdel, M. (2016). The Dangerous Condition of Ground during High Overburden Tunneling (A Case Study in Iran). Periodica Polytechnica Civil Engineering, 60(1): 11-20. https://doi.org/10.3311/ PPci.7923
Bamford, W.F. (1984). Rock test indices are being successfully correlated with tunnel boring machine performance. In: Proceedings of the 5th Australian Tunneling Conference, Melbourne, Vol. 2.
Benato, A. and P. Oreste (2015). Prediction of penetration per revolution in TBM tunneling as a function of intact rock and rock mass characteristics. Int. J. Rock Mech. Min. Sci., 74: 119-127.
Bieniawski, Z.T., Celada, B., Galera, J.M. and I. Tardáguila (2008). New applications of the excavability index for selection of TBM types and predicting their performance. ITA World Tunneling Congress, Agra, India.
Boyd, R.J. (1986). Hard rock continuous mining machine: Mobile Miner MM-120. In: D.F. Howarth et al. (Editors), Rock Excavation Engineering Seminar, Dept. Mining and Met. Eng, University of Queensland.
Büchi, E. (1984). Influence of geological parameters on the propulsion achievement of a tunnel drilling machine. Thesis, University of Berne.
Cho, J., Jeon, S., Jeong, H. and S. Chang (2013). Evaluation of cutting efficiency during TBM disc cutter excavation within a Korean granitic rock using linear-cutting-machine testing and photogrammetric measurement. Tunn. Undergr. Space Technol., 35: 37–54.
Farmer, I.W. and N.H. Glossop (1980). Mechanics of disc cutter penetration. Tunnels Tunnell. Int., 12(6): 22-25.
Farrokh, E., Rostami, J. and C. Laughton (2011). Analysis of unit supporting time and support installation time for Open TBMs. Rock Mechanics and Rock Engineering, 44(4): 431-445.
Geng, Q., Wei, Z., Meng, H. and F.J. Macias (2016). Mechanical performance of TBM cutterhead in mixed rock ground conditions. Tunn Undergr Space Technol, 57: 76-84. https://doi.org/10.1016/j.tust.2016.02.012
Giacomo, A. Maria, R. and F. Federica (2018). Geological and mechanical rock mass conditions for TBM performance prediction. The case of “La Maddalena” exploratory tunnel, Chiomonte (Italy). Tunnelling Underground Space Technol. 77: 115-12.
Graham, P.C. (1976). Rock exploration for machine manufacturers. In: Z.T. Bieniawski (Editor), Exploration for Rock Engineering. Balkema, Johannesburg.
Jakubowski, J., Stypulkowski, J.B. and F.G. Bernardeau (2017). Multivariate linear regression and CART regression analysis of TBM performance at Abu Hamour Phase-I tunnel. Arch Min Sci, 62(4): 825-841. https://doi. org/10.1515/amsc-2017-0057
Liu QS, Liu JP, Pan YC, Zhang XP, Peng XX, Gong QM, Du LJ (2017). A wear rule and cutter life prediction model of a 20-in TBM cutter for granite: A case study of a water conveyance tunnel in China. Rock Mech Rock Eng. https:// doi.org/10.1007/s00603-017-1176-4
Maji, V.B. and G.V. Theja (2017). A new performance prediction model for rock TBMs. Indian Geotech J, 47: 364-372. https://doi.org/10.1007/s40098-017-0226-x
Martin, D. (1988). TBM Tunnelling in Poor and Very Poor Rock Conditions. Tunnels and Tunnelling, 20(3): 22–27.
Mikaeil, R., Zare Naghadehi M. and S. Ghadernejad (2018). An extended multifactorial fuzzy prediction of hard rock TBM penetrability. Geotech Geol Eng, 36: 1779-1804. https://doi.org/10.1007/s10706-017-0432-4
Movinkel, T. and O. Johannessen (1986). Geological parameters for hard rock tunnel boring. Tunnels Tunnelling, 4: 45-48.
Roby, J., Sandell, T., Kocab, J. and L. Lindbergh (2008). Current state of disc cutter design and development directions. North American Tunneling 2008 Proceedings
Salimi, A., Rostami, J., Moormann, C. and J. Hassanpour (2018). Examining feasibility of developing a rock mass classification for hard rock TBM application using non-linear regression, regression tree and generic programming. Geotechn Geol Eng, 36: 1145-1159. https:// doi.org/10.1007/s10706-017-0380-z
Sapigni, M., Berti, M., Behtaz, E., Busillo, A. and G. Cardone (2002). TBM performance estimation using rock mass classification. Int. J. Rock Mech. Min. Sci., 39: 771-788.
Seo, Y., Macias, F.J., Jakobsen, P.D. et al. (2018). Influence of Subjectivity in Geological Mapping on the Net PenetrationRate Prediction for a Hard Rock TBM. Rock Mech Rock Eng, 51: 1599. https://doi.org/10.1007/s00603-018-1408-2
Tarkoy, P.J. (1973). Predicting TBM penetration rates in selected rock types. In: Proceedings, 9th Canadian Rock Mechanics Symposium, Montreal.
Thuro, K. and R.J. Plinninger (2003). Hard rock tunnel boring, cutting, drilling and blasting: Rock parameters for excavatability. In: Proceedings of the 10th ISRM International Congress on Rock Mechanics, Johannesburg, South Africa.
Yagiz, S. (2017). New equations for predicting the field penetration index of tunnel boring machines in fractured rock mass. Arab J Geosci, 10(2): 33. https://doi. org/10.1007/s12517-016-2811-1