Comparison of Different Artificial Neural Networks Techniques and Autoregressive Models for Forecasting of PM10
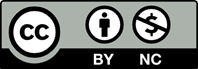
Atmospheric particulate matter (PM10) is one of the pollutant affecting human health significantly and has become a global issue. Data collected during three years in an urban area of Allahabad, Uttar Pradesh, India, are analysed and compared for 1-month ahead forecasting of PM10 using four models: Levenberg algorithm (LM) based artificial neural network (ANN), radial basis function neural network (RBFNN), generalized regression neural network (GRNN) and autoregressive (AR) model. Measured PM10 concentration are used as input to forecast the monthly averaged concentration of PM10 for one month ahead. The mean absolute percentage error (MAPE) for AR models varies from 10.20% to 32.78% whereas MAPE for ANN, RBFNN and GRNN are found 4.75%, 13.40% and 11.43% respectively, showing ANN model with LM algorithm forecast PM10 at one month ahead better than RBFNN, GRNN and AR models. In addition GRNN forecast is better than RBFNN with good accuracy. The average values of PM10 for Bharat Yantra Nigam Allahabad and Ardali Bazar Varanasi are found to be 182.16 and 262 respectively, showing Varanasi has high value of PM10. This study is useful for researcher working in forecasting of PM10.
Alkasassbeh, M., Mouhammad, Sheta, F.A., Faris, H. and H. Turabieh (2013). Prediction of PM10 and TSP Air Pollution Parameters Using Artificial Neural Network Autoregressive, External Input Models—A Case Study in Salt, Jordan. Journal of Scientific Research, 14(7): 999-1007.
Amodio, M., Andriani, E., de Gennaro, G., DemarinisLoiotile, A., Di Gilio, A. and M.C. Piacentino (2012). An integrated approach to identify the origin of PM10 exceedances. Environ. Sci. Pollut. Res., 19: 3132-3141.
Baawain, S.M. and A.S.A. Serihi (2014). Systematic Approach for the Prediction of Ground-Level Air Pollution (around an Industrial Port) Using an Artificial Neural Network. Aerosol and Air Quality Research, 14: 124-134.
Barbes, L., Neagu, C., Melnic, C., Ilie, C. and M. Velicu M (2009). The Use of Artificial Neural Network (ANN) for
Prediction of Some Airborne Pollutants Concentration in Urban Areas. Rev. Chim. (Bucureti), 60(3).
Biancofiore, F., Busilaccio, M., Verdecchia, M., Tomasseti, B., Aruffo, E., Bianco, S., Tommaso, D.S., Colangeli, C.,
Rosatelli, G. and D.P. Carlo (2017).. Recursive neural network model for analysis and forecast of PM10 and
PM2.5. Atmospheric Pollution Research, 1(8): 1042-1309.
Carnevale, C., Pisoni, E. and M. Volta, M. (2010). A nonlinear analysis to detect the origin of PM10 concentrations in Northern Italy. Sci. Total. Environ., 409: 182-191.
Chow, S.K.H., Lee, E.W.M. and D.H.W. Li (2012). Shortterm prediction of photovoltaic energy generation by
intelligent approach. Energy and Buildings, 55: 660-667.
Elminir, K.H. and A.H. Galil (2006). Estimation of air pollutant concentration from meteorological parameters
using Artificial Neural Network. Journal of Electrical Eng, 57(2): 105-110.
Frederick, M. (1996). Neuroshell 2 Manual. Ward Systems Group Inc.
Ghazi, S. and T.M. Khadir (2009). Recurrent Neural Network for multi-steps ahead prediction of PM10 concentration. J.
Automation and System Engineering.Grivas, G. and A. Chaloulakou (2006). Artificial neural network
models for prediction of PM10 hourly concentrations, in the Greater Area of Athens, Greece. Atmos. Environ., 40:1216-1229.
http://www.cpcb.gov.in/CAAQM/frmUserAvgReportCriteria.aspx.
http://www.uppcb.com/air_quality_nov.html.
Hooybergs, J., Mensink, C., Dumont, G., Fierens, F. and O. Brasseur (2005). A neural network forecast for daily
average PM10 concentrations in Belgium. Atmospheric Environment, 39: 3279-3289.
Kukkonen, J., Partanena, L., Karppinena, A., Ruuskanenb, J., Junninenb, H., Kolehmainenb, M., Niskab, H., Dorling, S., Chattertonc, T., Foxalld, R. and G. Cawleyd (2003). Extensive evaluation of neural network models for the prediction of NO2 and PM10 concentrations, compared with a deterministic modelling system and measurements in central Helsinki. Atmospheric Environment, 37: 4539-4550.
Lewis, C.D. (1982). International and Business Forecasting Methods. London: Butterworths.
Ostro, B., Chestnut, L., Vichit-Vadakan, N. and A. Laixuthai(1999). The impact of particulate matter on daily mortality in Bangkok, Thailand. J. Air Waste Manag. Assoc., 49: 100-107.
Ozel, G. and S. Chakmakyapan (2015). A new approach to the prediction of PM10 concentrations in Central Anatolia Region, Turkey. Atmospheric Pollution Research, 6: 735- 741.
Perez, P. and J. Reyes (2006). An integrated neural network model for PM10 forecasting. Atmospheric Environment,40: 2845-2851.
Rodriguez, S., Querol, X., Alastuey, A., Kallos, G. and O. Kakaliagou (2001). Saharan dust contribution to PM10 and TSP levels in Southern and Eastern Spain. Atmos. Environ., 35: 2433-2447.
Skyrzypski, J. and J.E. Szakiel (2008). Neural Network Prediction Models as a Tool for Air Quality Management in Cities. Journal of Environment Protection Engineering,34: 130-137.
Sonaje, N.P., Mane, S.J. and A.S. Kote (2013). Modelling of Respirable Suspended Particulate Matter Concentration Using Artificial Neural Networks in an Urban Area.International Journal of Engineering Science and Technology, 3(4): ISSN. 2250-3498.
Turner, M.C., Krewski, D., Pope, C.A., Chen, Y., Gapstur, S.M. and M.J. Thun (2011). Longterm ambient fine particulate matter air pollution and lung cancer in a large cohort of never-smokers. Am. J. Respir. Crit. Care Med.,184: 1374-1381.
www.inf.ufrgs.br/~engel/Common/CMP121/RBF_MATLAB. pdf.
Yadav, A.K., Malik, H. and S.S. Chandel (2014). Selection of most relevant input parameters using WEKA for artificial neural network based solar radiation prediction models. Renewable and Sustainable Energy Reviews, 31: 509-519.
Yadav, V. and Satyendra Nath (2017). Forecasting of PM10 Using Autoregressive Models and Exponential Smoothing Technique. Asian Journal of Water, Environment and Pollution, 14: 109-113.