CSO Technique for Solving the Economic Dispatch Problem Considering the Environmental Constraints
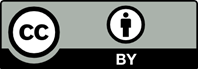
In this paper, the competitive swarm optimization (CSO) algorithm is applied for handling the economical load dispatch problem. The CSO algorithm is fundamentally encouraged by the particle swarm optimization (PSO) algorithm, but it does not memorize the personal best and global best to update the swarms. Rather in CSO algorithm, a pairwise competitive scenario was presented, where the loser particle is updated from the winner particle and the winner particles are directly accepted to the next population. The algorithm has been performed to find the generations of different units in a plant to reduce the entire fuel price and to maintain the total demand as well as the losses. The experimental study and investigations have revealed better performance for the CSO algorithm than the PSO and numerous state-of-art meta-heuristic algorithms in solving the economical power dispatch problem
Adamu, C.I., Nganje, T.N. and A. Edet (2015). Heavy metal contamination and health risk assessment associated with abandoned barite mines in Cross River State, Southeastern Nigeria. Environmental Nanotechnology, Monitoring & Management, 3: 10-21.
Bakirtzis, A., Petridis, V. and S. Kazarlis (1994). Genetic algorithm solution to the economic dispatch problem. IEE Proceedings Generation, Transmission and Distribution, IET, 141(4): 377.
Chen, P.H. and H.-C. Chang (1995). Large-scale economic dispatch by genetic algorithm. IEEE Transaction on Power Systems, 10(4): 1919-1926
Cheng, R. and Y. Jin (2014). A competitive swarm optimizer for large scale optimization. IEEE Transactions on Cybernetics, 99: 191-204.
Cheng, R., Sun, C. and Y. Jin (2013). A multi-swarm evolutionary framework based on a feedback mechanism. In: Proceedings of IEEE
Congress on Evolutionary Computation, IEEE. Chiang, C.L. (2005). Improved genetic algorithm for power economic dispatch of units with valve-point effects and multiple fuels. IEEE Transaction on Power Systems, 20(4): 1690-1699.
Chowdhury, B.H. and S. Rahman (1990). A review of recent advances in economic dispatch. IEEE Trans Power System, 5(4): 1248-1259.
Coelho, L.S. and V.C. Mariani (2006). Combing of chaotic differential evolution and quadratic programming for economic dispatch optimization with valve-point effect. IEEE Transactions on Power Systems, 21(2): 989-996.
Danaraj, R.M.S. and F. Gajendran (2005). Quadratic Programming Solution to Emission and Economic Dispatch Problems. Journal of the Institution of Engineers, 86: 129-132.
Dhar, R.N. and P.K. Mukherjee (1973). Reduced-gradient method for economic dispatch. Proceedings of the Institution of Electrical Engineers, 120(5): 608.
Elsayed, S.M., Sarker, R.A. and D.L. Essam (2013). An improved self-adaptive differential evolution algorithm for optimization problems industrial informatics. IEEE Trans. Ind. Inf., 9(1): 89-99.
Gaing, Z.L. (2003). Particle swarm optimization to solving the economic dispatch considering the generator constraints. IEEE Transanction on Power System, 18(3): 1187-1195.
Karthik, M.V. and A.S. Reddy (2014). Particle swarm optimization to solve economic dispatch considering generator constraints. The International Journal of Engineering and Science, 3(10): 94-100.
Granville, S. (1994). Optimal reactive dispatch through interior point methods. IEEE Transaction on Power System, 9(1): 136-146.
Liang, J., Qin, A., Suganthan, P.N. and S. Baskar, S. (2006). Comprehensive learning particle swarm optimizer for global optimization of multimodal functions. IEEE Transactions on Evolutionary Computation, 10(3): 281- 295.
Liang, Z.X. and J.D. Glover (1992). A zoom feature for a dynamic programming solution to economic dispatch including transmission losses. IEEE Transaction on Power System, 7(2): 544-550.
Mishra, M.P. (2012). Solution to Economic Load Dispatch Using PSO. National Institute of Technology, Rourkela.
Mohapatra, P., Das, K.N. and S. Roy (2017). A modified competitive swarm optimizer for large scale optimization problems. Applied Soft Computing, 59: 340-362
. Mohapatra, P., Das, K.N. and S. Roy (2019). Inherited competitive swarm optimizer for large scale optimizationproblems. Advances in Intelligent Systems and Computing, 741: 85-95.
Park, Y.M., Won, J.R. and J.B. Park (1998). A new approach to economic load dispatch based on improved evolutionary programming. Eng. Intell. Syst. Elect. Eng. Commun., 6(2): 103-110.
Shoultsa, R.R., Chakravartya, R.K. and R. Lowtherb (1996). Quasi-static economic dispatch using dynamic programming with an improved zoom feature. Electric Power Systems Research, 39(3): 215-222.
Sinha, N., Chakrabarti, R. and P.K. Chattopadhyay (2003). Evolutionary programming techniques for economic load dispatch. IEEE Transaction on Evolutionary Computation, 7: 83-94
Vanitha, M. and K. Thanushkodi (2011). Solution to economic dispatch problem by differential evolution algorithm considering linear equality and inequality constrains. International J. Res. Rev. Elec. Comp. Eng., 1(1): 21-27.
Yang, X.S., Hosseini, S.S.S. and A.H. Gandomi (2012). Firefly algorithm for solving non-convex economic dispatch problems with valve loading effect. Applied Soft Computing, 12: 1180-1186.
Yao, F. (2012). Quantum-inspired particle swarm optimization for power system operations considering wind power uncertainty and carbon tax in Australia. IEEE Transactions on Industrial Informatics, 8(4): 880-888.
Zhao, J.H. (2012). Optimal dispatch of electric vehicles and wind power using enhanced particle swarm optimization. IEEE Transaction on Power Systems, 8(4): 889-899