Pollution Control and Monitoring System with ML Based Analysis
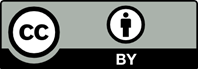
With climate change showing real and measurable effects on the day-to-day lives of millions around the world, it is high time to take necessary actions to curb pollution and greenhouse gases. Planning for a sustainable future has become a necessity. But to solve a problem of this scale and nature one needs to first understand it fully. Climate Change is not a localised problem, it is an effect of a global scale cause. Pollution and uncontrolled usage of unrenewable resources are two major factors in this global causation. To understand the extent of our effect on climate, an easy to use and distribute system for measurement will be crucial. In the reported work, the authors have designed and implemented an end-to-end Pollution Detection System with Machine Learning (ML) analysis of the data captured. It consists of two major parts, (a) Client-side Pollution Box which will be compact and cheap, fully integrated with sensors able to capture measurement points for air, water, light and noise pollution and (b) Server-side Cloud Data processing using Machine Learning techniques help to find patterns and correlation using data from the mesh. Mesh would be created by distributing these cheap pollution boxes over a locality or area
Castelli, M., Clemente, F.M., Popovic, A., Silva, S. and L. Vanneschi (2020). A machine learning approach to predict air quality in California. Complexity, 2020: 8049504.
Cheng, Y., He, K.B., Du, Z.Y., Zheng, M., Duan, F.K. and Ma, Y.L., 2015. Humidity plays an important role in the PM2.5 pollution in Beijing. Environmental Pollution, 197: 68-75.
Hegewald, J., Schubert, M., Freiberg, A., Romero Starke, K., Augustin, F., Riedel-Heller, S.G., Zeeb, H. and A. Seidler (2020). Traffic noise and mental health: A systematic review and meta-analysis. International Journal of Environmental Research and Public Health, 17: 6175.
Jechow, A., Kollath, Z., Lerner, A., Hanel, A., Shashar, N., Holker, F. and C. Kyba (2017). Measuring light pollution with fisheye lens imagery from a moving boat, a proof of concept. International Journal of Sustainable Lighting, 36(1): 15-25.
Jorgensen, D.G. (1996). The ratio method of estimating water resistivity and tds from resistivity logs. Groundwater, 34: 519-522.
Manisalidis, I., Stavropoulou, E., Stavropoulos, A. and E. Bezirtzoglou (2020). Environmental and health impacts of air pollution: A review. Frontiers in Public Health, 8: 14. doi:10.3389/fpubh.2020.00014
Min, J.Y. and K.B. Min (2018). Outdoor light at night and the prevalence of depressive symptoms and suicidal behaviors: A cross-sectional study in a nationally representative sample of Korean adults. Journal of Affective Disorders, 227: 199-205.
Ren, C. and S. Tong (2006). Temperature modifies the health effects of particulate matter in Brisbane, Australia. International Journal of Biometeorology, 51(2): 87-96.
Richards, M., Ghanem, M., Osmond, M., Guo, Y. and J. Hassard (2006). Grid-based analysis of air pollution data. Ecological Modelling, 194(1-3): 274-286.
Xu, Z.J. (2021). Time series pattern recognition with air quality sensor data. URL: https://towardsdatascience.com/ time-series-pattern-recognition-with-air-quality-sensordata-4b94710bb290bd81