Forecasting of PM10 Using Autoregressive Models and Exponential Smoothing Technique
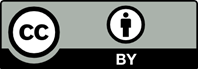
Particulate matter with 10 μm or less in diameter (PM10) have adverse effects on environment and human health. To reduce PM10 emissions in India, it is essential to have models that accurately estimate and predict PM10 concentrations for reporting and monitoring purposes. In this paper Exponential Smoothing Technique and Autoregressive (AR) models are developed to forecast 1-month ahead value of PM10 for Allahabad city which is novelty of this study. AR (1) and AR (5) models are developed using Burge and Yule Walker methods. The mean absolute percentage error (MAPE) for Burge method in AR (1) and AR (5) are 14.23% and 10.20%. The MAPE for Yule Walker method in AR (1) and AR (5) are 32.72% and 31.13%. The MAPE in Exponential Smoothing Technique is 5.81% which shows it forecasts better than AR model based on Burge and Yule Walker methods. It is found that Burge Method in AR (5) has less MAPE than Yule Walker Method. Therefore Exponential Smoothing Technique can be used to forecast PM10 for cities in India, showing it is beneficial for giving prior information for human health.
Alwee, R., Shams Uddin, H. and R. Sallehuddin (2013). Hybrid Support Vector Regression and Autoregressive Integrated Moving Average Models Improved by Particle Swarm Optimization for Property Crime Rates Forecasting with Economic Indicators. The Scientific World Journal, Article ID 951475, 1-11.
Chaudhuri, S., Das, D., Middey, A. and S. Goswami (2011). Forecasting the concentration of atmospheric pollutants: Skill assessment of Autoregressive and Radial Basis Function Network Models. International Journal of Environmental Protection, 1(5): 41-47.
Filho, A. and M. Fernandes (2013). Time series forecasting of pollutant concentration levels using Particle Swarm Optimization and Artificial Neural Networks. Quim. Nova, 36(6): 783-789.
http://www.uppcb.com/ambient_quality.htm
https://en.wikipedia.org/wiki/Autoregressive_model
Kaushik, I. and R. Melwan (2007). Time series analysis of ambient air quality at ITO intersection in Delhi (India). Journal of Environmental Research and Development, 2(2): 1-5.
Kumari, S., Jain, V.K. and Isha (2013). Autoregression model for the prediction of ambient air pollutant concentration in Delhi. International Journal of Environmental Science: Development and Monitoring, 4(2): ISSN 2231-1289.
Lewis, C.D. (1982). International and business forecasting methods. Butter-worths, London.
Liu, J. and L. Li (2015). Application Study of Comprehensive Forecasting Model Based on Entropy Weighting Method on Trend of PM2.5 Concentration in Guangzhou, China. International Journal of Environmental Research and Public Health, 12: 7085-7099.
Robles-Diaz, A.L., Bravo, O.C.J., Fu, S.J., Reed, D.G., Chow, C.J., Watson, G.J. and A.J. Herrera-Moncada (2008). A hybrid ARIMA and Artificial Neural Networks model to forecast the particulate matter in urban areas. Atmospheric Environment, 42: 8331-8340.
Salini, A. and P. Perez (2015). A Study of the Dynamic Behaviour of Fine Particulate Matter in Santiago, Chile. Journal of Aerosol and Air Quality Research, 15: 154-165.
Stoimenova, P. (2016). Stochastic Modeling of Problematic Air Pollution with Particulate Matter in the City of Pernik, Bulgaria. Ecologia Balkanica, 8(2): 33-44.
Syafei, D., Fujiwara, A. and J. Zhang (2015). Prediction Model of Air Pollutant Levels Using Linear Model with Component Analysis. International Journal of Environmental Science and Development, 6(7): 519-525.
Whalley, J. and S. ZandI (2016). Particulate matter sampling techniques and data modelling methods. Air quality measurement and modelling