Climate Change Scenario in the Gujarat Region— Analyses based on LARS-WG (Long Ashton Research Station-Weather Generator) Model
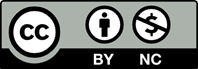
LARS-WG (Long Ashton Research Station-Weather Generator) model was used to predict future climate change scenario over the Gujarat region. For this purpose, three locations of the region namely Ahmedabad, Deesa and Vadodara for which long period daily weather data (1969 – 2013) are available were chosen.
The results indicated that LARS-WG model adequately predicted monthly rainfall means and standard deviations and they were in agreement with the observed series as reflected in the t and f-tests at 5% probability. The agreement was even better for maximum and minimum air temperatures at all the three locations of the Gujarat region. LARS-WG-predicted climate change scenario indicated an increasing trend in annual and seasonal rainfall at Ahmedabad and it would increase by 6.7% i.e. 54.3 mm (in 2020) to 18.2% i.e. 147.4 mm (in 2060) against the base period normal rainfall of 807.5 mm. Seasonal rainfall (monsoon) also has shown an increasing trend and it would increase by 5% (38.0 mm) in 2020 to 14.5% (110.8 mm) in 2060 against the average seasonal rainfall of 766.4 mm for the base period. At Deesa and Vadodara no trend has been observed in the annual and seasonal rainfall scenario. Standard weekly rainfall during the monsoon season has been found to increase at the start as well as in the middle of the season during 2014 to 2063 compared to the base period (1969-2013) at Deesa and Vadodara; however, no such changes have been observed for Ahmedabad. For all the three locations no trend has been observed in the annual average minimum and maximum temperature as well as in the summer maximum and winter minimum temperature
Abdul Haris, A., Khan, M.A., Chhabra, V., Biswas, S. and A. Pratap (2010). Evaluation of LARS-WG for generating long term data for assessment of climate change impact in Bihar. J. Agrometeorol., 12(2): 198-201.
Binh, P.D. (2012). Simulation of climate change scenarios for Phu Luong watershed in northern Vietnam. J. Environ. Sci. Eng., 1: 1263-1270.
Chia, E. and M.F. Hutchinson (1991). The beta distribution as a probability model for daily cloud duration. J. Agriculture for Meterology, 56: 195-208.
Hashmi, M.Z., Shamseldin, A.Y. and B.W. Melville (2012). Downscaling of future rainfall extreme events: A weather generator based approach. In 18th World IMACS/MODSIM Congress, Cairns, Australia.
Kumar, D., Arya, D.S., Murumkar, A.R. and M.M. Rahman (2013). Impact of climate change on rainfall in northwestern Bangladesh using multi-GCM ensembles. Int. J. Climatol., DOI:10.1002/JOC.3770.
Mahat, V. and A. Anderson (2013). Impacts of climate and catastrophic forest changes on streamflow and water balance in a mountainous headwater stream in Southern Alberta. Hydrol. Earth Syst. Sci., 17: 4941-4956.
Racsko P., Szeidl, L. and M. Semenov (1991). A serial approach to local stochastic weather models. J. Ecological Modelling, 57: 27-41.
Reddy, K.S., Kumar, M., Nagarjuna Kumar, R., Umesha, B., Vijayalakshmi and B. Venkateswarlu (2012). Long term rainfall and temperature analysis through ClimGen model in Ranga Reddy district of Andhra Pradesh. J. Agrometeorol. (Spec. Issue-I), 15: 45-50.
Robert, S.W. and S.W. John (1998). Statistics, 8th ed. John Wiley & Sons, Inc., USA,
Wilks, D.S. (1992). Adapting stochastic weather generation algorithms for climate changes studies. Clim Change, 22: 67-84.
Semenov, M.A. and E.M. Barrow (1997). Use of a stochastic weather generator in the development of climate change scenarios. Clim. Chang., 35(4): 397-414.
Semenov, M.A., Brooks, R.J., Barrow, E.M. and C.W. Richardson (1998). Comparison of the WGEN and LARS-WG stochastic weather generators for diverse climates. Clim Res., 10(2): 95-107.
Semenov, M.A. (2008). Simulation of extreme weather events by a stochastic weather generator. Clim Res., 35: 203-212.
Wilks, D.S. and R.L. Wilby (1999). The weather generation game: A review of stochastic weather models. Prog Phys Geogr., 23: 329-357.