Fuzzy and Neuro-Fuzzy Modelling for Prediction of Effluent COD for a Real Scale UASB Reactor Treating Distillery Wastewater
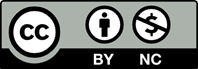
The high rate anaerobic processes are used for treatment of industrial wastewater. These processes are subjected to many disturbances due to variation in quantity and quality of wastewater. Neuro-fuzzy (ANFIS) and fuzzy models were developed for prediction of effluent COD for an UASB reactor treating distillery wastewater. Different combinations of input parameters were assessed to obtain the ANFIS model. A fuzzy rule based model was developed with same combination of input parameters as ANFIS model. Expert’s knowledge, plant operator’s opinion and trend of the data were considered during model development. Parameters required for daily monitoring and operation of the plant were used for model development. Statistical parameters like the correlation coefficient (R) and root mean square error (RMSE) were used to assess the performance of these models. The value of 0.8922 and 1334.69 for R and RMSE were observed with the ANFIS model whereas 0.8197 and 4208.34 were obtained with the fuzzy model respectively. These values indicate good agreement between the predicted and observed values of effluent COD and satisfactory performance of the models. The modelling approach discussed in this paper will be useful for assessment of performance of the real scale UASB reactor and to control the operation of the plant.
Belanche, L.A., Vades, J.J., Comas, J., Roda, I.R. and M. Poch (1999). Towards a model of input-output behavior of wastewater treatment plants using soft computing techniques. Environmental Modelling & Software, 14: 409-419.
Cakmakci, M. (2007). Adaptive neuro-fuzzy modeling of anaerobic digestion of primary sedimentation sludge. Springer. Bioprocess Biosyst Engineering, 30: 349-357.
Carrasco, E.F., Rodriguez, J., Punal, A., Roca, E. and J.M. Lema (2004). Diagnosis of acidification states in an anaerobic wastewater treatment plant using a fuzzy-based expert system. Control Engineering Practice, 12: 59-64.
Estaben, M., Polit, M. and J.P. Steyer (1997). Fuzzy control for an anaerobic digester. Control Eng. Practice, 5(98): 1303-1310.
Garcia, C., Molina, F., Roca, E. and J.M. Lema (2007). Fuzzy-based Control of an Anaerobic Reactor Treating Wastewaters containing Ethanol and Carbohydrates. Industrial Engineering Chemical Research, 46: 6707-
6715.
Genovesi, A., Armand, J. and J.P. Steyer (2000). Integrated Fault Detection and Isolation: Application to a Winery’s Wastewater Treatment Plant. Applied Intelligence, 13: 59-76.
Huang, M., Wan, J., Ma, Y., Li, W., Sun, X. and Y. Wan (2010). A fast predicting neural fuzzy model for on-line estimation of nutrients dynamics in anoxic/oxic processes. Bioresource Technology, 101( 6): 1642-1651.
Jang, J.S.R. (1993). ANFIS: Adaptive-Network based Fuzzy Inference System. IEEE 9. Transactions on System, Man and Cybernetics, 23(3).
Jang, J.S.R. and C.T. Sun (1995). Neuro-Fuzzy Modeling and Control. Proceedings of IEEE, March 1995.
Lopez, I. and L. Borzacconi (2009). Modeling a full scale UASB reactor using a COD global balance approach and state observers. Chemical Engineering Journal, 146(1): 1-5.
Manesis, S.A., Sapidis, D.J. and R.E. King (1997). Intelligent control of wastewater treatment plants. Artificial Intelligence in Engineering, 12: 275-281.
Perendeci, A., Arsan, S., Tanyolac, A. and S.S. Celebi (2007). Evaluation of Input Variables in Adaptive–Network-Based Fuzzy Inference System Modeling for an Anaerobic Wastewater Treatment Plant under unsteady state. ASCE, Journal of Environmental Engineering, 133(7): 765-771.
Perendeci, A., Arsan, S., Celebi, S.S. and A. Tanyolac (2008). Prediction of an anaerobic treatment plant under unsteady state through ANFIS modeling with on-line input variables. Chemical Engineering Journal, 145(1): 78-85.
Polit, M., Genovesi, A. and B. Claudet (2001). Fuzzy logic observers for a biological wastewater treatment process. Applied Numerical Mathematics, 39: 173-180.
Polit, M., Estaben, M. and P. Labat (2002). A fuzzy model for an anaerobic digester, comparison with experimental results. Pergamon, Artificial Intelligence, 15: 385-390.
Punal, A., Palazzotto, L., Bouvier, J.C., Conte, T. and I.P. Steyer (2003). Automatic control of volatile fatty acids in anaerobic digestion using a fuzzy logic based approach. Water Science and Technology, 48(6): 103-110.
Sadiq, R., Al-Zahrani, M.A., Shaikh, A.K., Husain, T. and S. Farooq (2004). Performance evaluation of slow sand filters using fuzzy rule-based modeling. Environmental Modeling & Software, 19: 507-515.
Sarayu Mohana, Acharya, B.K. and D. Madamwar (2009). Distillery Spent wash: Treatment technologies and potential applications. Journal of Hazardous Materials,163(1): 12-25.
Tewari, P.K., Batra, V.S. and M. Balkrishnan (2007). Water management initiatives in sugarcane molasses based distilleries in India. Resources, Conservation & Recycling,52: 351-367.
Tay, J.H. and X. Zhang (1999). Neural Fuzzy Modeling of anaerobic biological wastewater treatment system. Journal of Environmental Engineering, 125(12): 1149-1159.
Tay, J.H. and X. Zhang (2000). Stability of high rate anaerobic systems. II: Fuzzy Stability Index. Journal of Environmental Engineering, 126(8): 726-731.
Tay, J.H. and X. Zhang (2000). A fast predicting Neural Fuzzy model for high rate anaerobic wastewater treatment systems. Water Research, 34(11): 2849-2860.
Traore, A., Grieu, S., Thiery, F., Polit, M. and J. Colprim (2006). Control of sludge height in a secondary settler using fuzzy algorithms. Computers and Chemical Engineering, 30: 1235-1242.