Rational drug design from phosphatidylinositol 3-kinase-α inhibitors through molecular docking and 3D-QSAR methodologies for cancer immunotherapy
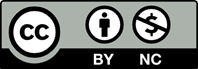
Dysregulation or aberrant activation of the phosphatidylinositol 3-kinase (PI3K) signaling pathway is commonly observed in various cancers and is associated with tumor growth, metastasis, and resistance to therapy. Targeting PI3K-α with appropriate inhibitors can disrupt this pathway, hindering cancer progression, and potentially enhancing the immune system’s ability to recognize and eliminate cancer cells. In this study, we aimed to design a novel and potent inhibitor of PI3K-α for cancer immunotherapy using rational drug design techniques, including virtual screening, molecular docking, and 3D-QSAR. We obtained the human PI3K-α protein (6PYS) complexed with (3S)-3-benzyl-3-methyl-5-[5-(2-methylpyrimidin-5-yl)pyrazolo[1,5-a]pyrimidin-3-yl]-1,3-dihydro-2H-indol-2-one (PJ5) from the RCSB Protein Data Bank. Virtual screening of ligands, integrated with predictive computational molecular docking and 3D-field-based-QSAR, was implemented using appropriate Schrödinger Maestro modules. Rational drug design was also carried out, and its clinical relevance was validated across several ADMET descriptors. Docking results suggested that a hybrid of sulfonamide and pyridine-based heterocyclic compounds, functionalized with potent moieties derived from alkaloids, exhibited adequate synergistic biological effects capable of enhancing sufficient biological activity against PI3K-α. A field-based 3D-QSAR model was built on four partial least squares factors, and five statistical metrics were employed to validate the model. The newly designed ligand from this approach, named 6’-amino-5’-(2-fluoro-1,3-oxazol-5-yl)-N-{[3-(hydroxymethyl)oxetan-3-yl]methyl}-3-methyl-[2,3’-bipyridine]-6-sulfonamide or T85, exhibited a predicted bioactivity (pIC50) of 8.25. The predicted ADMET properties of T85 fell reasonably within the range of recommended standards, especially adhering to Lipinski’s rule of five and Jorgensen’s rule of three. In conclusion, the results of this study offer significant insights into in silico drug design using a rational approach, which could expedite the discovery and development of new drug molecules.
- Toppmeyer DL, Press MF. Testing considerations for phosphatidylinositol-3-kinase catalytic subunit Alpha as an emerging biomarker in advanced breast cancer. Cancer Med. 2020;9:6463-6472. doi: 10.1002/cam4.3278
- Mansour MA, Lasheen DS, Gaber HM, Abouzid KAM. Elaborating piperazinyl-furopyrimidine based scaffolds as phosphoinositol-3-kinase enzyme alpha (PI3Kα) inhibitors to combat pancreatic cancer. RSC Adv. 2020;10(53):32103-32112. doi: 10.1039/D0RA06428A
- Castillo JJ, Furman M, Winer ES. CAL-101: A phosphatidylinositol-3-kinase p110-delta inhibitor for the treatment of lymphoid malignancies. Expert Opin Investig Drugs. 2012;21:15-22. doi: 10.1517/13543784.2012.640318
- Akinleye A, Avvaru P, Furqan M, Song Y, Liu D. Phosphatidylinositol 3-kinase (PI3K) inhibitors as cancer therapeutics. J Hematol Oncol. 2013;6:88. doi: 10.1186/1756-8722-6-88
- Burris HA. Overcoming acquired resistance to anticancer therapy: Focus on the PI3K/AKT/mTOR pathway. Cancer Chemother Pharmacol. 2013;71:829-842. doi: 10.1007/s00280-012-2043-3
- Popova NV, Jücker M. The role of mTOR signaling as a therapeutic target in cancer. Int J Mol Sci. 2021;22(4):1743. doi: 10.3390/ijms22041743
- Liu P, Cheng H, Roberts TM, Zhao JJ. Targeting the phosphoinositide 3-kinase pathway in cancer. Nat Rev Drug Discov. 2009;8(8):627-644. doi: 10.1038/nrd2926
- Saal LH, Johansson P, Holm K, et al. Poor prognosis in carcinoma is associated with a gene expression signature of aberrant PTEN tumor suppressor pathway activity. Proc Natl Acad Sci U S A. 2007;104(18):7564-7569. doi: 10.1073/pnas.0702507104
- Millis SZ, Ikeda S, Reddy S, Gatalica Z, Kurzrock R. Landscape of phosphatidylinositol-3-kinase pathway alterations across 19 784 diverse solid tumors. JAMA Oncol. 2016;2(12):1565-1573. doi: 10.1001/jamaoncol.2016.0891
- Vivanco I, Sawyers C. The phosphatidylinositol 3-Kinase-AKT pathway in human cancer. Nat Rev Cancer. 2002;2:489-501. doi: 10.1038/nrc839
- Markman B, Dienstmann R, Tabernero J. Targeting the PI3K/Akt/mTOR pathway--beyond rapalogs. Oncotarget. 2010;1:530-543. doi: 10.18632/oncotarget.188
- Courtney KD, Corcoran RB, Engelman JA. The PI3K pathway as drug target in human cancer. J Clin Oncol. 2010;28:1075-1083. doi: 10.1200/JCO.2009.25.3641
- Vanhaesebroeck B, Leevers SJ, Panayotou G, Waterfield MD. Phosphoinositide 3-kinases: A conserved family of signal transducers. Trends Biochem Sci. 1997;22:267-272. doi: 10.1016/S0968-0004(97)01061-X
- Reif K, Okkenhaug K, Sasaki T, Penninger JM, Vanhaesebroeck B, Cyster JG. Cutting edge: Differential roles for phosphoinositide 3-Kinases, p110gamma and p110delta, in lymphocyte chemotaxis and homing. J Immunol. 2004;173:2236-2240. doi: 10.4049/jimmunol.173.4.2236
- Williams R, Berndt A, Miller S, Hon WC, Zhang X. Form and flexibility in phosphoinositide 3-kinases. Biochem Soc Trans. 2009;37(4):615-626. doi: 10.1042/BST0370615
- Engelman JA, Luo J, Cantley LC. The evolution of phosphatidylinositol 3-kinases as regulators of growth and metabolism. Nat Rev Genet. 2006;7:606-619. doi: 10.1038/nrg1879
- Katso R, Okkenhaug K, Ahmadi K, White S, Timms J, Waterfield MD. Cellular function of phosphoinositide 3-Kinases: Implications for development, homeostasis, and cancer. Annu Rev Cell Dev Biol. 2001;17:615-675. doi: 10.1146/annurev.cellbio.17.1.615
- Raffoul JJ, Kucuk O, Sarkar FH, Hillman GG. Dietary agents in cancer chemoprevention and treatment. J Oncol. 2012;2012:749310. doi: 10.1155/2012/749310
- Kasala ER, Bodduluru LN, Barua CC, Sriram CS, Gogoi R. Benzo(a)pyrene induced lung cancer: Role of dietary phytochemicals in chemoprevention. Pharmacol Rep. 2015;67:996-1009. doi: 10.1016/j.pharep.2015.03.004
- Backer JM. The regulation and function of class III PI3Ks: Novel roles for Vps34. Biochem J. 2008;410:1-17. doi: 10.1042/BJ20071427
- Yadav RR, Guru SK, Joshi P, et al. 6-Aryl substituted 4-(4-cyanomethyl) phenylamino quinazolines as a new class of isoform-selective PI3K-alpha inhibitors. Eur J Med Chem. 2016;122:731-743. doi: 10.1016/j.ejmech.2016.07.006
- Hauptman N, Jevšinek D, Glavač D. Noncoding RNA alterations in cancer molecular pathways. In: Cancer and Noncoding RNAs. Cambridge: Academic Press; 2018. p. 247-268. doi: 10.1016/b978-0-12-811022-5.00014-0
- Furet P, Guagnano V, Fairhurst RA, et al. Discovery of NVP-BYL719 a potent and selective phosphatidylinositol-3 kinase alpha inhibitor selected for clinical evaluation. Bioorg Med Chem Lett. 2013;23(13):3741-3748. doi: 10.1016/j.bmcl.2013.05.007
- Nunnery SE, Mayer IA. Management of toxicity to isoform α-specific PI3K inhibitors. Ann Oncol . 2019;30:x21-x26. doi: 10.1093/annonc/mdz440
- Utermark T, Rao T, Cheng H, et al. The p110α and p110β isoforms of PI3K play divergent roles in mammary gland development and tumorigenesis. Genes Dev. 2012;26(14):1573-1586. doi: 10.1101/gad.191973.112
- Sabbah DA, Simms NA, Brattain MG, Vennerstrom JL, Zhong H. Biological evaluation and docking studies of recently identified inhibitors of phosphoinositide-3-kinases. Bioorg Med Chem Lett. 2012;22(2):876-880. doi: 10.1016/j.bmcl.2011.12.044
- Bhaskar BV, Rammohan A, Babu TM, et al. Molecular insight into isoform specific inhibition of PI3K-α and PKC-η with dietary agents through an ensemble pharmacophore and docking studies. Sci Rep. 2021;11(1):12150. doi: 10.1038/s41598-021-90287-3
- Ali AM, Makki AA, Ibraheem W, et al. Design of novel phosphatidylinositol 3-kinase inhibitors for non-hodgkin’s lymphoma: Molecular docking, molecular dynamics, and density functional theory studies on gold nanoparticles. Molecules. 2023;28(5):2289. doi: 10.3390/molecules28052289
- Dirican E, Akkiprik M. Phosphatidylinositol 3-kinase regulatory subunit 1 and phosphatase and tensin homolog as therapeutic targets in breast cancer. Tumour Biol. 2017;39:101042831769552. doi: 10.1177/1010428317695529
- Saldanha SN, Tollefsbol TO. The role of nutraceuticals in chemoprevention and chemotherapy and their clinical outcomes. J Oncol. 2012;2012:192464. doi: 10.1155/2012/192464
- Mirzaei S, Ghodsi R, Hadizadeh F, Sahebkar A. 3D-QSAR-Based pharmacophore modeling, virtual screening, and molecular docking studies for identification of tubulin inhibitors with potential anticancer activity. Biomed Res Int. 2021;2021:6480804. doi: 10.1155/2021/6480804
- Wasukan N, Kuno M, Maniratanachote R. Molecular docking as a promising predictive model for silver nanoparticle-mediated inhibition of cytochrome P450 enzymes. J Chem Inf Model. 2019;59:5126-5134. doi: 10.1021/acs.jcim.9b00572
- Dibia KT, Igbokwe PK, Ezemagu GI, Asadu CO. Exploration of the quantitative structure-activity relationships for predicting cyclooxygenase-2 inhibition bioactivity by machine learning approaches. Results Chem. 2022;4:100272. doi: 10.1016/j.rechem.2021.100272
- Chen X, Liu M, Gilson MK. BindingDB: A web-accessible molecular recognition database. Comb Chem High Throughput Screen. 2001;4(8):719-725. doi: 10.2174/1386207013330670
- Liu T, Lin Y, Wen X, Jorissen RN, Gilson MK. BindingDB: A web-accessible database of experimentally determined protein-ligand binding affinities. Nucleic Acids Res. 2007;35:D198-D201. doi: 10.1093/nar/gkl999
- Bateman A, Martin MJ, Orchard S, et al. UniProt: The universal protein knowledgebase in 2023. Nucleic Acids Res. 2023;51(D1):D523-D531. doi: 10.1093/nar/gkac1052
- Fan C, Chen M, Wang X, Wang J, Huang B. A review on data preprocessing techniques toward efficient and reliable knowledge discovery from building operational data. Front Energy Res. 2021;9:652801. doi: 10.3389/fenrg.2021.652801
- Alasadi SA, Bhaya WS. Review of data preprocessing techniques in data mining. J Eng Appl Sci. 2017;12(16):4102-4107. doi: 10.3923/jeasci.2017.4102.4107
- Kanwar G, Kumar A, Mahajan A. Open source software tools for computer aided drug design. Int J Res Pharm Sci. 2018;9(1):86-95. doi: 10.26452/ijrps.v9i1.1191
- Lindahl ER. Molecular dynamics simulations. Methods Mol Biol. 2008;443:3-23. doi: 10.1007/978-1-59745-177-2_1
- McGreig JE, Uri H, Antczak M, Sternberg MJE, Michaelis M, Wass MN. 3DLigandSite: Structure-based prediction of protein-ligand binding sites. Nucleic Acids Res. 2022;50(W1):W13-W20. doi: 10.1093/nar/gkac250
- Mukhopadhyay A, Borkakoti N, Pravda L. Finding enzyme cofactors in Protein Data Bank. Bioinformatics. 2019;35(18):3510-3511. doi: 10.1093/bioinformatics/btz115
- Torrance JW, MacArthur MW, Thornton JM. Evolution of binding sites for zinc and calcium ions playing structural roles. Proteins. 2008;71(2):813-830. doi: 10.1002/prot.21741
- Zhao J, Cao Y, Zhang L. Exploring the computational methods for protein-ligand binding site prediction. Comput Struct Biotechnol J. 2020;18:417-426. doi: 10.1016/j.csbj.2020.02.008
- Roche DB, Brackenridge DA, McGuffin LJ. Proteins and their interacting partners: An introduction to protein-ligand binding site prediction methods. Int J Mol Sci. 2015;16:29829-29842. doi: 10.3390/ijms161226202
- Kudo G, Hirao T, Yoshino R, Shigeta Y, Hirokawa T. Pocket to concavity: A tool for the refinement of protein-ligand binding site shape from Alpha spheres. Bioinformatics. 2023;39(4):btad212. doi: 10.1093/bioinformatics/btad212
- Heifetz A, Katchalski-Katzir E, Eisenstein M. Electrostatics in protein-protein docking. Protein Sci. 2009;11(3):571-587. doi: 10.1110/ps.26002
- Palma PN, Krippahl L, Wampler JE, Moura JJ. Bigger: A new (soft) docking algorithm for predicting protein interactions. Proteins. 2000;39(4):372-384. doi: 10.1002/(SICI)1097-0134(20000601)39:4<372:AID-PROT100>3.0.CO;2-Q
- Adiyaman R, McGuffin LJ. Methods for the refinement of protein structure 3D models. Int J Mol Sci. 2019;20:2301. doi: 10.3390/ijms20092301
- Madhavi G, Adzhigirey M, Day T, Annabhimoju R, Sherman W. Protein and ligand preparation: Parameters, protocols, and influence on virtual screening enrichments. J Comput Aided Mol Des. 2013;27(3):221-234. doi: 10.1007/s10822-013-9644-8
- Lerm JA, Meyners C, Christmann A, et al. Binding pocket stabilization by high-throughput screening of yeast display libraries. Front Mol Biosci. 2022;9:1023131. doi: 10.3389/fmolb.2022.1023131
- Müller I. Guidelines for the successful generation of protein-ligand complex crystals. Acta Crystallogr D Struct Biol. 2017;73:79-92. doi: 10.1107/S2059798316020271
- Guterres H, Park SJ, Jiang W, Im W. Ligand-binding-site refinement to generate reliable holo protein structure conformations from apo structures. J Chem Inf Model. 2020;61(1):535-546. doi: 10.1021/acs.jcim.0c01354
- Shin WH, Kumazawa K, Imai K, Hirokawa T, Kihara D. Current challenges and opportunities in designing protein-protein interaction targeted drugs. Adv Appl Bioinform Chem. 2020;13:11-25. doi: 10.2147/AABC.S235542
- Halgren TA. Identifying and characterizing binding sites and assessing druggability. J Chem Inf Model. 2009;49(2):377-389. doi: 10.1021/ci800324m
- De Wolf FA, Brett GM. Ligand-binding proteins: Their potential for application in systems for controlled delivery and uptake of ligands. Pharmacol Rev. 2000;52(2):207-236.
- Sriramulu DK, Lee SG. Combinatorial effect of ligand and ligand-binding site hydrophobicities on binding affinity. J Chem Inf Model. 2020;60(3):1678-1684. doi: 10.1021/acs.jcim.9b01143
- Shin YS, Lee JY, Noh S, et al. Discovery of cyclic sulfonamide derivatives as potent inhibitors of SARS-CoV-2. Bioorg Med Chem Lett. 2021;31:127667. doi: 10.1016/j.bmcl.2020.127667
- Wan Y, Fang G, Chen H, Deng X, Tang Z. Sulfonamide derivatives as potential anti-cancer agents and their SARs elucidation. Eur J Med Chem. 2021;226:113837. doi: 10.1016/j.ejmech.2021.113837
- Hamed FM, Hassan BA, Abdulridha MM. The antitumor activity of sulfonamides derivatives: Review. Int J Pharm Res. 2020;12:2512. doi: 10.31838/ijpr/2020.SP1.390
- Ghorab MM, Alsaid MS, El-Gaby MSA, Safwat NA, Elaasser MM, Soliman AM. Biological evaluation of some new N-(2,6- dimethoxypyrimidinyl) thioureido benzenesulfonamide derivatives as potential antimicrobial and anticancer agents. Eur J Med Chem. 2016;124:299-310. doi: 10.1016/j.ejmech.2016.08.060
- Bano S, Javed K, Ahmad S, Rathish IG, Singh S, Alam MS. Synthesis and biological evaluation of some new 2-pyrazolines bearing benzene sulfonamide moiety as potential anti-inflammatory and anti-cancer agents. Eur J Med Chem. 2011;46(12):5763-5768. doi: 10.1016/j.ejmech.2011.08.015
- Alaoui S, Dufies M, Driowya M, et al. Synthesis and anti-cancer activities of new sulfonamides 4-substituted-triazolyl nucleosides. Bioorg Med Chem Lett. 2017;27(9):1989-1992. doi: 10.1016/j.bmcl.2017.03.018
- Stokes SS, Albert R, Buurman ET, et al. Inhibitors of the acetyltransferase domain of N-acetylglucosamine-1- phosphate-uridylyltransferase/glucosamine-1-phosphate-acetyltransferase (GlmU). Part 2: Optimization of physical properties leading to antibacterial aryl sulfonamides. Bioorg Med Chem Lett. 2012;22(23):7019-7023. doi: 10.1016/j.bmcl.2012.10.003
- Konda S, Raparthi S, Bhaskar K, et al. Synthesis and antimicrobial activity of novel benzoxazine sulfonamide derivatives. Bioorg Med Chem Lett. 2015;25(7):1643-1646. doi: 10.1016/j.bmcl.2015.01.026
- Durgun M, Turkmen H, Zengin G, Zengin H, Koyunsever M, Koyuncu I. Synthesis, characterization, in vitro cytotoxicity and antimicrobial investigation and evaluation of physicochemical properties of novel 4-(2-methylacetamide)benzenesulfonamide derivatives. Bioorg Chem. 2017;70:163-172. doi: 10.1016/j.bioorg.2016.12.007
- Lal J, Gupta SK, Thavaselvam D, Agarwal DD. Biological activity, design, synthesis and structure activity relationship of some novel derivatives of curcumin containing sulfonamides. Eur J Med Chem. 2013;64:579-588. doi: 10.1016/j.ejmech.2013.03.012
- Ning X, Guo Y, Ma X, et al. Design, synthesis and pharmacological evaluation of (E)-3,4-dihydroxy styryl sulfonamides derivatives as multifunctional neuroprotective agents against oxidative and inflammatory injury. Bioorg Med Chem. 2013;21(17):5589-5597. doi: 10.1016/j.bmc.2013.05.043
- Abbas A, Murtaza S, Tahir MN, et al. Synthesis, antioxidant, enzyme inhibition and DNA binding studies of novel N-benzylated derivatives of sulfonamide. J Mol Struct. 2016;1117:269-275. doi: 10.1016/j.molstruc.2016.03.066
- Chandna N, Kumar S, Kaushik P, et al. Synthesis of novel celecoxib analogues by bioisosteric replacement of sulfonamide as potent anti-inflammatory agents and cyclooxygenase inhibitors. Bioorg Med Chem. 2013;21(15):4581-4590. doi: 10.1016/j.bmc.2013.05.029
- Lu XY, Wang ZC, Ren SZ, Shen FQ, Man RJ, Zhu HL. Coumarin sulfonamides derivatives as potent and selective COX-2 inhibitors with efficacy in suppressing cancer proliferation and metastasis. Bioorg Med Chem Lett. 2016;26(15):3491-3498. doi: 10.1016/j.bmcl.2016.06.037
- Naim MJ, Alam O, Alam MJ, et al. Design, synthesis and molecular docking of thiazolidinedione based benzene sulphonamide derivatives containing pyrazole core as potential anti-diabetic agents. Bioorg Chem. 2018;76:98-112. doi: 10.1016/j.bioorg.2017.11.010
- Mechelke M, Habeck M. Robust probabilistic superposition and comparison of protein structures. BMC Bioinformatics. 2010;11:363. doi: 10.1186/1471-2105-11-363
- Darling HS. Do you have a standard way of interpreting the standard deviation? A narrative review. Cancer Res Stat Treat. 2022;5(4):728-733. doi: 10.4103/crst.crst_284_22
- Lee DK, In J, Lee S. Standard deviation and standard error of the mean. Korean J Anesthesiol. 2015;68(3):220-223. doi: 10.4097/kjae.2015.68.3.220
- Zhang D. A coefficient of determination for generalized linear models. Am Stat. 2017;71(4):310-316. doi: 10.1080/00031305.2016.1256839
- Prinz F, Schlange T, Asadullah K. Believe it or not: How much can we rely on published data on potential drug targets? Nat Rev Drug Discov. 2011;10:712. doi: 10.1038/nrd3439-c1
- Jawarkar RD, Bakal RL, Khatale PN, et al. QSAR, pharmacophore modeling and molecular docking studies to identify structural alerts for some nitrogen heterocycles as dual inhibitor of telomerase reverse transcriptase and human telomeric G-quadruplex DNA. Futur J Pharm Sci. 2021;7(1):231. doi: 10.1186/s43094-021-00380-7
- Tropsha A, Cho SJ. Perspectives in Drug Discovery and Design. Vol. 12. United States: Springer; 1998. p. 57-69. doi: 10.1023/a:1017017601586
- Consonni V, Ballabio D, Todeschini R. Comments on the definition of the Q2 Parameter for QSAR Validation. J Chem Inf Model. 2009;49(7):1669-1678. doi: 10.1021/ci900115y
- Nguyen XS, Mouaddib AI, Nguyen TP. Hierarchical gaussian descriptor based on local pooling for action recognition. Mach Vis Appl. 2019;30(2):321-343. doi: 10.1007/s00138-018-0989-9
- Raschka S, Wolf AJ, Bemister-Buffington J, Kuhn LA. Protein-ligand interfaces are polarized: Discovery of a strong trend for intermolecular hydrogen bonds to favor donors on the protein side with implications for predicting and designing ligand complexes. J Comput Aided Mol Des. 2018;32(4):511-528. doi: 10.1007/s10822-018-0105-2
- Chen D, Oezguen N, Urvil P, Ferguson C, Dann SM, Savidge TC. Regulation of protein-ligand binding affinity by hydrogen bond pairing. Sci Adv. 2016;2(3):e1501240. doi: 10.1126/sciadv.1501240
- Bulusu G, Desiraju GR. Strong and weak hydrogen bonds in protein-ligand recognition. J Indian Inst Sci. 2020;100:31-41. doi: 10.1007/s41745-019-00141-9
- Jurczyk J, Woo J, Kim SF, Dherange BD, Sarpong R, Levin MD. Single-atom logic for heterocycle editing. Nat Synth. 2022;1:352-364. doi: 10.1038/s44160-022-00052-1
- Barnes-Seeman D, Beck J, Springer C. Fluorinated compounds in medicinal chemistry: Recent applications, synthetic advances and matched-pair analyses. Curr Top Med Chem. 2014;14(7):855-864. doi: 10.2174/1568026614666140202204242
- Chandra G, Singh DV, Mahato GK, Patel S. Fluorine-a small magic bullet atom in the drug development: Perspective to FDA approved and COVID-19 recommended drugs. Chem Zvesti. 2023;77, 4085-4106. doi: 10.1007/s11696-023-02804-5
- U.S. Food and Drugs Administrations: Novel Drug Approvals for 2021; 2021. Available from: https://www.fda.gov/ drugs/new-drugs-fda-cders-new-molecular-entities-and-new-therapeutic-biological-products/novel-drug-approvals-2021 [Last accessed on 2023 Sep 11].
- Pal S, Chandra G, Patel S, Singh S. Fluorinated nucleosides: Synthesis, modulation in conformation and therapeutic application. Chem Rec. 2022;22:e202100335. doi: 10.1002/tcr.202100335
- Shet H, Sahu R, Sanghvi YS, Kapdi AR. Strategies for the synthesis of fluorinated nucleosides, nucleotides and oligonucleotides. Chem Rec. 2022;22:e202200066. doi: 10.1002/tcr.202200066
- Grygorenko OO, Melnykov KP, Holovach S, Demchuk O. Fluorinated cycloalkyl building blocks for drug discovery. ChemMedChem. 2022;17:e202200365. doi: 10.1002/cmdc.202200365
- Jena S, Dutta J, Tulsiyan KD, Sahu AK, Choudhury SS, Biswal HS. Noncovalent interactions in proteins and nucleic acids: Beyond hydrogen bonding and π-stacking. Chem Soc Rev. 2022;51:4261-4286. doi: 10.1039/d2cs00133k
- Hirota S, Lin YW. Design of artificial metalloproteins/ metalloenzymes by tuning noncovalent interactions. J Biol Inorg Chem. 2018;23:7-25. doi: 10.1007/s00775-017-1506-8
- Yunta MJR. It is important to compute intramolecular hydrogen bonding in drug design? Am J Model Optim. 2017;5(1):24-57. doi: 10.12691/ajmo-5-1-3
- Gilli G, Gilli P. The nature of the hydrogen bond: outline of a comprehensive hydrogen bond theory. In: International Union of Crystallography Monographs on Crystallography. Oxford: Oxford Academic; 2009. doi: 10.1093/acprof:oso/9780199558964.001.0001
- Deng JH, Luo J, Mao YL, et al. Π-π stacking interactions: Non-negligible forces for stabilizing porous supramolecular frameworks. Sci Adv. 2020;6(2):eaax9976. doi: 10.1126/sciadv.aax9976
- Chen T, Li M, Liu J. Π-π stacking interaction: A nondestructive and facile means in material engineering for bioapplications. Cryst Growth Des. 2018;18(5):2765-2783. doi: 10.1021/acs.cgd.7b01503
- Martinez CR, Iverson BL. Rethinking the term “pi-stacking”. Chem Sci. 2012;3:2191-2201. doi: 10.1039/c2sc20045g
- Press S. QikProp 4.4 User Manual. New York, NY: LLC, Schrödinger Press; 2015.
- Zhu Y, Alqahtani S, Hu X. Aromatic rings as molecular determinants for the molecular recognition of protein kinase inhibitors. Molecules. 2021;26(6):1776. doi: 10.3390/molecules26061776