The Application of Artificial Intelligence in Breast Cancer
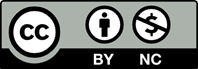
The utilization of artificial intelligence (AI) in the detection and treatment of breast cancer is attracting attention. AI technologies are crucial in shaping the future of breast surgery and enhancing healthcare services. Deep learning algorithms show promise in accurately detecting breast cancer from mammograms and clinical data, even predicting the risk of interval and advanced cancers. When combined with breast density measurements, AI imaging algorithms can predict invasive breast cancers, particularly in later stages. AI-based methods can also forecast breast cancer from ultrasound scans, improving malignancy detection. Genetic testing with AI assists in identifying individuals at high risk for breast cancer based on genetic profiles, enabling personalized screening and prevention strategies. AI tools support pathologists in analyzing tissue samples for breast cancer indications, enhancing diagnoses. The integration of AI in breast cancer detection and prediction has the potential to revolutionize oncology and improve patient care. This review offers a thorough analysis of previous academic studies on the use of AI in breast cancer.
1. Goel A, Goel AK, Kumar A. The role of artificial neural network and machine learning in utilizing spatial information. Spat Inform Res 2023;31:275–85.
2. Pinto-Coelho L. How artificial intelligence is shaping medical imaging technology: A survey of innovations and applications. Bioengineering (Basel) 2023;10:1435.
3. Farina E, Nabhen JJ, Dacoregio MI, Batalini F, Moraes FY. An overview of artificial intelligence in oncology. Future Sci OA 2022;8:FSO787.
4. Sufyan M, Shokat Z, Ashfaq UA. Artificial intelligence in cancer diagnosis and therapy: Current status and future perspective. Comput Biol Med 2023;165:107356.
5. Jiang X, Hu Z, Wang S, Zhang Y. Deep learning for medical image-based cancer diagnosis. Cancers (Basel) 2023;15:3608.
6. Alowais SA, Alghamdi SS, Alsuhebany N, Alqahtani T, Alshaya AI, Almohareb SN, et al. Revolutionizing healthcare: the role of artificial intelligence in clinical practice. BMC Med Educ 2023;23:689.
7. Johnson KB, Wei WQ, Weeraratne D, Frisse ME, Misulis K, Rhee K, et al. Precision medicine, ai, and the future of personalized health care. Clin Transl Sci 2021;14:86–93.
8. Tan XJ, Cheor WL, Lim LL, Ab Rahman KS, Bakrin IH. Artificial Intelligence (AI) in breast imaging: A scientometric umbrella review. Diagnostics (Basel) 2022;12:3111.
9. Ahn JS, Shin S, Yang SA, Park EK, Kim KH, Cho SI, et al. Artificial intelligence in breast cancer diagnosis and personalized medicine. J Breast Cancer 2023;26:405–35.
10. Liu Q, Qu M, Sun L, Wang H. Accuracy of ultrasonic artificial intelligence in diagnosing benign and alignant breast diseases: A protocol for systematic review and meta-analysis. Medicine (Baltimore) 2021;100:e28289.
11. Elsharawy KA, Gerds TA, Rakha EA, Dalton LW. Artificial intelligence grading of breast cancer: a promising method to refine prognostic classification for management precision. Histopathology 2021;79:187–99.
12. Rao A, Manley L, Smith S. Use of artificial intelligence to identify patients to be assessed in a breast clinic on 2-week wait: A retrospective cohort study. Ann Med Surg (Lond) 2023;85:5459–63.
13. Davalagi SB, Palicheralu BS, Murthy SSN, Hurlihal S. Acceptance of artificial intelligence (AI)-based creening for breast health in urban slums of central Karnataka, India - SWOC analysis. J Family Med Prim Care 2022;11:6023–8.
14. Sadoughi F, Kazemy Z, Hamedan F, Owji L, Rahmanikatigari M, Azadboni TT. Artificial intelligence methods for the diagnosis of breast cancer by image processing: A review. Breast Cancer (Dove Med Press) 2018;10:219–30.
15. Mahant SS, Varma AR. Artificial intelligence in breast ultrasound: the emerging future of modern medicine. Cureus 2022;14:e28945.
16. Taylor CR, Monga N, Johnson C, Hawley JR, Patel M. Artificial intelligence applications in breast imaging: current status and future directions. Diagnostics (Basel) 2023;13:2041.
17. Witowski J, Heacock L, Reig B, Kang SK, Lewin A, Pysarenko K, et al. Improving breast cancer diagnostics with deep learning for MRI. Sci Transl Med 2022;14:eabo4802.
18. Abdolahi M, Salehi M, Shokatian I, Reiazi R. Artificial intelligence in automatic classification of invasive uctal carcinoma breast cancer in digital pathology images. Med J Islam Repub Iran 2020;34:140.
19. Aidossov N, Zarikas V, Zhao Y, Mashekova A, Ng EYK, Mukhmetov O, et al. An integrated intelligent system for breast cancer detection at early stages using IR Images and machine learning methods with explainability. SN Comput Sci 2023;4:184.
20. Hamyoon H, Yee Chan W, Mohammadi A, Yusuf Kuzan T, MirzaAghazadeh-Attari M, Leong WL, et al. Artificial intelligence, BIRADS evaluation and morphometry: A novel combination to diagnose breast cancer using ultrasonography, results from multi-center cohorts. Eur J Radiol 2022;157:110591.
21. Seth I, Bulloch G, Joseph K, Hunter-Smith DJ, Rozen WM. Use of artificial intelligence in the advancement of breast surgery and implications for breast reconstruction: A narrative review. J Clin Med 2023;12:5143.
22. Nawrat Z. Introduction to AI-driven surgical robots. Artific Intell Surg 2023;3:90–7.
23. Sheetz KH, Claflin J, Dimick JB. Trends in the adoption of robotic surgery for common surgical procedures. JAMA Netw Open 2020;3:e1918911.
24. Denecke K, Baudoin CR. A review of artificial intelligence and robotics in transformed health ecosystems. Front Med (Lausanne) 2022;9:795957.
25. Batko K, Ślęzak A. The use of big data analytics in healthcare. J Big Data 2022;9:3.
26. Kaul V, Enslin S, Gross SA. History of artificial intelligence in medicine. Gastrointest Endosc 2020;92:807–12.
27. Ali O, Abdelbaki W, Shrestha A, Elbasi E, Alryalat MAA, Dwivedi YK. A systematic literature review of artificial intelligence in the healthcare sector: Benefits, challenges, methodologies, and functionalities. J Innov Knowl 2023;8:100333.
28. Xu N, Yang D, Arikawa K, Bai C. Application of artificial intelligence in modern medicine. Clinical eHealth 2023;6:130–7.
29. Hamet P, Tremblay J. Artificial intelligence in medicine. Metabolism 2017;69S:S36–40.
30. Deo N, Anjankar A. Artificial intelligence with robotics in healthcare: A narrative review of its viability in India. Cureus 2023;15:e39416.
31. Davenport T, Kalakota R. The potential for artificial intelligence in healthcare. Future Healthc J 2019;6:94–8.
32. Weerarathna IN, Kamble AR, Luharia A. Artificial intelligence applications for biomedical cancer research: A review. Cureus 2023;15:e48307.
33. Kann BH, Hosny A, Aerts HJWL. Artificial intelligence for clinical oncology. Cancer Cell 2021;39:916–27.
34. Bhalla S, Laganà A. Artificial intelligence for precision oncology. Adv Exp Med Biol 2022;1361:249–68.
35. Sebastian AM, Peter D. Artificial intelligence in cancer research: trends, challenges and future directions. Life (Basel) 2022;12:1991.
36. Amiri Z, Heidari A, Navimipour NJ, Esmaeilpour M, Yazdani Y. The deep learning applications in IoT-based bio- and medical informatics: a systematic literature review. Neural Comput Appl 2024;36:5757–97.
37. Li M, Jiang Y, Zhang Y, Zhu H. Medical image analysis using deep learning algorithms. Front Public Health 2023;11:1273253.
38. Karalis VD. The integration of artificial intelligence into clinical practice. Appl Biosci 2024;3:14–44.
39. Adler-Milstein J, Aggarwal N, Ahmed M, Castner J, Evans BJ, Gonzalez AA, et al. Meeting the moment: addressing barriers and facilitating clinical adoption of artificial intelligence in medical diagnosis. NAM Perspect 2022;2022:10.31478/202209c.
40. Abdel-Qader I, Abu-Amara F. A computer-aided diagnosis system for breast cancer using independent component analysis and fuzzy classifier. Model Simul Eng 2008;2008:238305.
41. Arun Kumar S, Sasikala S. Review on deep learning-based CAD systems for breast cancer diagnosis. Technol Cancer Res Treat 2023;22:15330338231177977.
42. Al-Antari MA, Al-Masni MA, Choi MT, Han SM, Kim TS. A fully integrated computer-aided diagnosis system for digital X-ray mammograms via deep learning detection, segmentation, and classification. Int J Med Inform 2018;117:44–54.
43. Zuluaga-Gomez J, Masry Z Al, Benaggoune K, Meraghni S, Zerhouni N. A CNN-based methodology for breast cancer diagnosis using thermal images. Available at: https://arxiv.org/abs/1910.13757. Accessed Aug 16, 2024.
44. Oyelade ON, Ezugwu AE. A deep learning model using data augmentation for detection of architectural distortion in whole and patches of images. Biomed Sig Proc Control 2021;65:102366.
45. Kadhim YA, Khan MU, Mishra A. Deep learning-based Computer-Aided Diagnosis (CAD): applications for medical image datasets. Sensors (Basel) 2022;22:8999.
46. Lokaj B, Pugliese MT, Kinkel K, Lovis C, Schmid J. Barriers and facilitators of artificial intelligence conception and implementation for breast imaging diagnosis in clinical practice: A scoping review. Eur Radiol 2024;34:2096–109.
47. Chan HP, Samala RK, Hadjiiski LM. CAD and AI for breast cancer-recent development and challenges. Br J Radiol 2020;93:20190580.
48. Kelly CJ, Karthikesalingam A, Suleyman M, Corrado G, King D. Key challenges for delivering clinical impact with artificial intelligence. BMC Med 2019;17:195.
49. Syed AH, Khan T. Evolution of research trends in artificial intelligence for breast cancer diagnosis and prognosis over the past two decades: A bibliometric analysis. Front Oncol 2022;12:854927.
50. Shah SM, Khan RA, Arif S, Sajid U. Artificial intelligence for breast cancer analysis: Trends & directions. Comput Biol Med 2022;142:105221.
51. Wang SH, Chen G, Zhong X, Lin T, Shen Y, Fan X, et al. Global development of artificial intelligence in cancer field: a bibliometric analysis range from 1983 to 2022. Available at: https://www.frontiersin.org/journals/oncology/articles/10.3389/ fonc.2023.1215729/full. Accessed Aug 16, 2024.
52. Kazakova VA, Shivilov E V., Anichkina KA, Tyulyakova SA, Pasternak A V. On the Introduction of Artificial Intelligence into the Daily Practice of Breast Pathology Diagnosis: has Everything been Taken into Account? Radiol Pract 2023;3:36–49.
53. Shen Y, Shamout FE, Oliver JR, Witowski J, Kannan K, Park J, et al. Artificial intelligence system reduces false-positive findings in the interpretation of breast ultrasound exams. Nature Commun 2021;12:5645.
54. Arce S, Vijay A, Yim E, Spiguel LR, Hanna M. Evaluation of an artificial intelligence system for detection of invasive lobular carcinoma on digital mammography. Cureus 2023;15:e38770.
55. Lu W, Lashen AG, Wahab N, Miligy IM, Jahanifar M, Toss M, et al. AI-based intra-tumor heterogeneity score of Ki67 expression as a prognostic marker for early-stage ER+/HER2- breast cancer. J Pathol Clin Res 2024;10:e346.
56. Jung M, Song SG, Cho SI, Shin S, Lee T, Jung W, et al. Augmented interpretation of HER2, ER, and PR in breast cancer by artificial intelligence analyzer: enhancing interobserver agreement through a reader study of 201 cases. Breast Cancer Res 2024;26:31.
57. Jones MA, Islam W, Faiz R, Chen X, Zheng B. Applying artificial intelligence technology to assist with breast cancer diagnosis and prognosis prediction. Front Oncol 2022;12:980793.
58. Wu S, Yue M, Zhang J, Li X, Li Z, Zhang H, et al. The role of artificial intelligence in accurate interpretation of HER2 immunohistochemical scores 0 and 1+ in breast cancer. Mod Pathol 2023;36:100054.
59. Geras KJ, Mann RM, Moy L. Artificial intelligence for mammography and digital breast tomosynthesis: current concepts and future perspectives. Radiology 2019;293:246–59.
60. Rodriguez-Ruiz A, Lång K, Gubern-Merida A, Teuwen J, Broeders M, Gennaro G, et al. Can we reduce the workload of mammographic screening by automatic identification of normal exams with artificial intelligence? A feasibility study. Eur Radiol 2019;29:4825–32.
61. Salim M, Wåhlin E, Dembrower K, Azavedo E, Foukakis T, Liu Y, et al. External evaluation of 3 commercial artificial intelligence algorithms for independent assessment of screening mammograms. JAMA Oncol 2020;6:1581–8.
62. Yoon JH, Kim EK. Deep learning-based artificial intelligence for mammography. Korean J Radiol 2021;22:1225–39.
63. Raya-Povedano JL, Romero-Martín S, Elías-Cabot E, GubernMérida A, Rodríguez-Ruiz A, Álvarez-Benito M. AI-based strategies to reduce workload in breast cancer screening with mammography and tomosynthesis: A retrospective evaluation. Radiology. 2021;300:57–65.
64. Wang Y, Acs B, Robertson S, Liu B, Solorzano L, Wählby C, et al. Improved breast cancer histological grading using deep learning. Ann Oncol 2022;33:89–98.
65. Boissin C, Wang Y, Sharma A, Weitz P, Karlsson E, Robertson S, et al. Deep learning-based risk stratification of preoperative breast biopsies using digital whole slide images. Breast Cancer Res 2024;26:90.
66. Du J, Yang YC, An ZJ, Zhang MH, Fu XH, Huang ZF, et al. Advances in spatial transcriptomics and related data analysis strategies. J Transl Med 2023;21:330.
67. Khan A, Ali L, Wei DQ. Editorial: Breast cancer resistance, biomarkers and therapeutics development in the era of artificial intelligence. Front Mol Biosci 2022;9:1034990.
68. Alharbi WS, Rashid M. A review of deep learning applications in human genomics using next-generation sequencing data. Hum Genomics 2022;16:26.
69. Jongbloed EM, Jansen MPHM, de Weerd V, Helmijr JA, Beaufort CM, Reinders MJT, et al. Machine learning-based somatic variant calling in cell-free DNA of metastatic breast cancer patients using large NGS panels. Sci Rep 2023;13:10424.
70. Jafari A. Machine-learning methods in detecting breast cancer and related therapeutic issues: A review. Comput Methods Biomech Biomed Eng Imaging Visual 2024;12:1–11.
71. Reyna MA, Haan D, Paczkowska M, Verbeke LPC, Vazquez M, Kahraman A, et al; PCAWG Consortium. Pathway and network analysis of more than 2500 whole cancer genomes. Nat Commun 2020;11:729.
72. Zou FW, Tang YF, Liu CY, Ma JA, Hu CH. Concordance study between ibm watson for oncology and real clinical practice for cervical cancer patients in China: A retrospective analysis. Front Genetic 2020;11:512564.
73. Liu C, Liu X, Wu F, Xie M, Feng Y, Hu C. Using artificial intelligence (watson for oncology) for treatment recommendations amongst chinese patients with lung cancer: Feasibility study. J Med Internet Res 2018;20:e11087.
74. Schapin N, Majewski M, Varela-Rial A, Arroniz C, Fabritiis G De. Machine learning small molecule properties in drug discovery. Artific Intell Chem 2023;1:100020.
75. Gao Y, Chen S, Tong J, Fu X. Topology-enhanced molecular graph representation for anti-breast cancer drug selection. BMC Bioinformatics 2022;23:382.
76. Paul D, Sanap G, Shenoy S, Kalyane D, Kalia K, Tekade RK. Artificial intelligence in drug discovery and development. Drug Discov Today 2021;26:80–93.
77. Wang M, Wang Z, Sun H, Wang J, Shen C, Weng G, et al. Deep learning approaches for de novo drug design: An overview. Curr Opin Struct Biol 2022;72:135–44.
78. Gu Y, Li J, Kang H, Zhang B, Zheng S. Employing molecular conformations for ligand-based virtual screening with equivariant graph neural network and deep multiple instance learning. Molecules 2023;28:5982.
79. Bian Y, Kwon JJ, Liu C, Margiotta E, Shekhar M, Gould AE. Target-driven machine learning-enabled virtual screening (TAME-VS) platform for early-stage hit identification. Front Mol Biosci 2023;10:1163536.
80. Singh S, Gupta H, Sharma P, Sahi S. Advances in Artificial Intelligence (AI)-assisted approaches in drug screening. Artific Intell Chem 2024;2:100039.