Malignant versus normal breast tissue: Optical differentiation exploiting hyperspectral imaging system
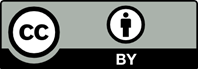
Breast malignancy is a critical problem that severely affects women’s health globally with a high-frequency rate, necessitating fast, effective, and early diagnostic methods. The present study aims to measure the breast tissue’s optical properties by capturing the spectral signatures from malignant and normal breast tissue for therapeutic and diagnostic applications. The optical imaging system incorporates a hyperspectral (HS) camera to capture the spectral signatures for both the malignant and normal breast tissues within 400 ~ 1000 nm. The system was subdivided into two exploratory (reflection/transmission) to measure the tissue’s diffuse reflectance (Rd) and light transmission (Tr), respectively. The study involved 30 breast tissue (normal/tumor) samples from 30 females in the age range of 46 ~ 72 years, who were optically inspected in the visible and near-infrared (VIS-NIR) spectra. Then, the inverse adding doubling (IAD) method for breast tissue characterization and descriptive analysis (T-test) was exploited to verify the significant difference between the various types of breast tissues and select the optimum wavelength. Finally, comparing the study outcome with the histopathological examination to evaluate the system’s effectiveness by calculation (sensitivity, specificity, and accuracy). The average outcome values demonstrated that the optimal spectral bands distinguishing between the normal and the tumor tissues regarding the reflectance approach were 600 ~ 680 nm and 750 ~ 960 nm at the VIS and NIR spectrum, respectively. Then, for the transmission technique, the optimal spectral bands were 560 ~ 590 nm and 760 ~ 810 nm at the VIS and NIR spectra, respectively. Later, the T-test and the IAD verified that the highest Rd values for discrimination were 600 ~ 640 nm and 800 ~ 840 nm at the VIS and NIR spectra, respectively. On the other side, the highest Tr values were 600 ~ 640 nm and 760 ~ 800 nm at the VIS and NIR spectra, respectively. The investigation’s average reading accuracy, sensitivity, and specificity were 85%, 81.88%, and 88.8%, respectively. The experimental trials revealed that the system could identify the optimal wavelength for therapeutic and diagnostic applications through the light interaction behavior of the breast tissue’s optical properties.
Ferlay J, Colombet M, Soerjomataram I, et al., 2021, Cancer statistics for the year 2020: An overview. Int J Cancer, 149(4): 778–789. https://doi.org/10.1002/ijc.33588
Sung H, Ferlay J, Siegel RL, et al., 2021, Global cancer statistics 2020: GLOBOCAN estimates of incidence and mortality worldwide for 36 cancers in 185 countries. CA Cancer J Clin, 71(3): 209–249. https://doi.org/10.3322/caac.21660
World Health Organization, 2021, World Health Statistics 2021. Geneva: World Health Organization.
Fitzmaurice C, Dicker D, Pain A, et al., 2015, The global burden of cancer 2013. JAMA Oncol, 1(4): 505–527. https://doi.org/10.1001/jamaoncol.2015.0735
Ferlay J, Soerjomataram I, Dikshit R, et al., 2015, Cancer incidence and mortality worldwide: Sources, methods and major patterns in GLOBOCAN 2012. Int J Cancer, 136(5): E359–E386. https://doi/org/10.1002/ijc.29210
Mody GN, Nduaguba A, Ntirenganya F, et al., 2013, Characteristics and presentation of patients with breast cancer in Rwanda. Am J Surg, 205(4): 409–413. https://doi.org/10.1016/j.amjsurg.2013.01.002
Faust O, Acharya UR, Meiburger KM, et al., 2018, Comparative assessment of texture features for the identification of cancer in ultrasound images: A review. Biocybern Biomed Eng, 38(2): 275–296.
Etehadtavakol M, Ng EY, 2013, Breast thermography as a potential non-contact method in the early detection of cancer: A review. J Mech Med Biol, 13(2): 1330001.
Nofiele JT, Czarnota GJ, Cheng HL, 2014, Noninvasive manganese-enhanced magnetic resonance imaging for early detection of breast cancer metastatic potential. Mol Imaging, 13(1): 1–10. https://doi.org/10.2310/7290.2013.00071
Gøtzsche PC, Jørgensen KJ, 2013, Screening for breast cancer with mammography. Cochrane Database Syst Rev, 2013(6): CD001877. https://doi.org/10.1002/14651858.CD001877.pub5
Brem RF, Lenihan MJ, Lieberman J, et al., 2015, Screening breast ultrasound: Past, present, and future. Am J Roentgenol, 204(2): 234–240. https://doi.org/10.2214/AJR.13.12072
Hambly NM, Liberman L, Dershaw DD, et al., 2011, Background parenchymal enhancement on baseline screening breast MRI: Impact on biopsy rate and short-interval follow-up. Am J Roentgenol, 196(1): 218–224. https://doi.org/10.2214/AJR.10.4550
Mehnati P, Tirtash MJ, 2015, Comparative efficacy of four imaging instruments for breast cancer screening. Asian Pac J Cancer Prev, 16(15): 6177–6186. https://doi.org/10.7314/apjcp.2015.16.15.6177
Gutierrez RM, Cerquera EA, Mañana G, 2012, MPGD for breast cancer prevention: A high resolution and low dose radiation medical imaging. J Instrum, 7(7): C07007. https://doi.org/10.1088/1748-0221/7/07/C07007
Wang L, 2017, Early diagnosis of breast cancer. Sensors (Basel), 17(7): 1572. https://doi.org/10.3390/s17071572
Breastcancer.org (2006). Your Guide to the Breast Cancer Pathology Report. Available from: https://www.breastcancer. org [Last accessed on 2022 Nov 15].
Sahu A, 2012, Hyperspectral Imaging to Discern Malignant and Benign Canine Mammary Tumors a Thesis Proposal Submitted to the Temple University Graduate Board By Amrita Sahu.
Ouyang Y, Tsui P, Wu S, et al., 2019, Classification of benign and malignant breast tumors using H-scan ultrasound imaging. Diagnostics (Basel), 9(4): 182. https://doi.org/10.3390/diagnostics9040182
Merrill AL, Coopey SB, Tang R, et al., 2016, Implications of new lumpectomy margin guidelines for breast-conserving surgery: Changes in reexcision rates and predicted rates of residual tumor. Ann Surg Oncol, 23(3): 729–734. https://doi.org/10.1245/s10434-015-4916-2
Vos EL, Jager A, Verhoef C, et al., 2015, Overall survival in patients with a re-excision following breast conserving surgery compared to those without in a large population-based cohort. Eur J Cancer, 51(3): 282–291. https://doi.org/10.1016/j.ejca.2014.12.003
Kho E, Dashtbozorg B, de Boer LL, et al., 2019, Broadband hyperspectral imaging for breast tumor detection using spectral and spatial information. Biomed Opt Express, 10(9): 4496. https://doi.org/10.1364/boe.10.004496
Boyce BF, 2017, An update on the validation of whole slide imaging systems following FDA approval of a system for a routine pathology diagnostic service in the United States. Biotech Histochem, 92(6): 381–389. https://doi.org/10.1080/10520295.2017.1355476
Evans AJ, Bauer TW, Bui MM, et al., 2018, US Food and Drug Administration approval of whole slide imaging for primary diagnosis: A key milestone is reached and new questions are raised. Arch Pathol Lab Med, 142(11): 1383–1387. https://doi.org/10.5858/arpa.2017-0496-CP
Flotte TJ, Bell DA, 2018, Anatomical pathology is at a crossroads. Pathology, 50(4): 373–374. https://doi.org/10.1016/j.pathol.2018.01.003
Motomura K, Inaji H, Komoike Y, et al., 2000, Intraoperative sentinel lymph node examination by imprint cytology and frozen sectioning during breast surgery. Br J Surg, 87(5): 597–601. https://doi/org/10.1046/j.1365-2168.2000.01423.x
Flett MM, Going JJ, Stanton PD, et al., 1998, Sentinel node localization in patients with breast cancer. Br J Surg, 85(7): 991–993. https://doi.org/10.1046/j.1365-2168.1998.00746.x
Van Diest PJ, Torrenga H, Borgstein PJ, et al., 1999, Reliability of intraoperative frozen section and imprint cytological investigation of sentinel lymph nodes in breast cancer. Histopathology, 35(1): 14–18. https://doi.org/10.1046/j.1365-2559.1999.00667.x
Aref M, Youssef AB, Hussein AA, et al., 2022, Custom fluorescence imaging system exploiting hyperspectral camera to characterize and diagnose RNA breast cancer. Biointerface Res Appl Chem, 12(4): 5548–5566. https://doi.org/10.33263/BRIAC124.55485566
Welch AJ, Van Gemert MJ, 2011, Optical-Thermal Response of Laser-Irradiated Tissue Vol. 2. Berlin: Springer.
Bioucas-Dias JM, Plaza A, Camps-Valls G, et al., 2013, Hyperspectral remote sensing data analysis and future challenges. IEEE Geosci Remote Sens Magazine, 1(2): 6–36.
Adão T, Hruška J, Pádua L, et al., 2017, Hyperspectral imaging: A review on UAV-based sensors, data processing and applications for agriculture and forestry. Remote Sens, 9(11): 1110. https://doi.org/10.3390/rs9111110
Robles-Kelly A, Huynh CP, 2013, Imaging spectroscopy for scene analysis. In: Imaging Spectroscopy for Scene Analysis. Berlin: Springer. https://doi.org/10.1007/978-1-4471-4652-0
Lu G, Fei B, 2014, Medical hyperspectral imaging: A review. J Biomed Opt, 19(1): 10901. https://doi.org/10.1117/1.JBO.19.1.010901
Fabelo H, Ortega S, Lazcano R, et al., 2018, An intraoperative visualization system using hyperspectral imaging to aid in brain tumor delineation. Sensors (Basel), 18(2): 430. https://doi.org/10.3390/s18020430
Goto A, Nishikawa J, Kiyotoki S, et al., 2015, Use of hyperspectral imaging technology to develop a diagnostic support system for gastric cancer. J Biomed Opt, 20(1): 16017. https://doi.org/10.1117/1.JBO.20.1.016017
Regeling B, Thies B, Gerstner AO, et al., 2016, Hyperspectral imaging using flexible endoscopy for laryngeal cancer detection. Sensors (Basel), 16(8): 1288. https://doi.org/10.3390/s16081288
Aref MH, Youssef AB, Aboughaleb IH, et al., 2021, Prospective study for commercial and low-cost hyperspectral imaging systems to evaluate thermal tissue effect on bovine liver samples. J Spectral Imaging, 10: a5.
Aref MH, Aboughaleb IH, Youssef AB, et al., 2021, Hyperspectral image-based analysis of thermal damage for ex-vivo bovine liver utilizing radiofrequency ablation. Surg Oncol, 38: 101564. https://doi.org/10.1016/j.suronc.2021.101564
El-Sharkawy YH, Aref MH, Elbasuney S, et al., 2022, Oxygen saturation measurements using novel diffused reflectance with hyperspectral imaging: Towards facile COVID-19 diagnosis. Opt Quantum Electron, 54(5): 322. https://doi.org/10.1007/s11082-022-03658-z
Aref MH, Sharawi AA, El-Sharkawy YH, 2021, Delineation of the arm blood vessels utilizing hyperspectral imaging to assist with phlebotomy for exploiting the cutaneous tissue oxygen concentration. Photodiagnosis Photodyn Ther, 33: 102190. https://doi.org/10.1016/j.pdpdt.2021.102190
Aref M, Youssef AB, Hussein AA, et al., 2021, Custom fluorescence imaging system exploiting hyperspectral camera to characterize and diagnose RNA breast cancer. Biointerface Res Appl Chem, 2: 5548–5566.
Aref MH, Abbass MA, Youssef AB, et al., 2022, Optical Characterization of Biological Tissues in Visible and Near- Infrared Spectra. In: 2022 13th International Conference on Electrical Engineering (ICEENG). IEEE. p. 159–163.
Aboughaleb IH, Matboli M, Shawky SM, et al., 2021, Transcriptome spectral analysis using hyperspectral imaging for hepatocellular carcinoma detection. QJM Int J Med, 114(Suppl 1): hcab088-004.
Bashkatov AN, Genina EA, Kochubey VI, et al., 2005, Optical properties of human skin, subcutaneous and mucous tissues in the wavelength range from 400 to 2000 nm. J Phys D Appl Phys, 38(15): 2543–2555. https://doi.org/10.1088/0022-3727/38/15/004
Ostantini IR, Icchi RI, Ilvestri LU, et al., 2019, In-vivo and ex-vivo optical clearing methods for biological tissues : Review. Biomed Opt Express, 10(10): 5251–5267. https://doi.org/10.1364/BOE.10.005251
Tuchin VV, 1997, Light scattering study of tissues. Phys Uspekhi, 40(5): 495–515. https://doi.org/10.1070/pu1997v040n05abeh000236
Aref MH, Youssef AB, Aboughaleb IH, et al., 2021, Characterization of normal and malignant breast tissues utilizing hyperspectral images and associated differential spectrum algorithm. J Biomed Photon Eng, 7(2): 1–12. https://doi.org/10.18287/JBPE21.07.020302
Fajardo C, Solarte E, 2020, Optical properties of a simple model of soft biological tissue. J Phys Conf Ser, 1547: 012026. https://doi.org/10.1088/1742-6596/1547/1/012026
Pogue BW, Patterson MS, 2022, Review of tissue simulating phantoms for optical spectroscopy, imaging and dosimetry. J Biomed Opt, 11: 041102. https://doi.org/10.1117/1.2335429
Patterson MS, Wilson BC, Wyman DR, 1991, The propagation of optical radiation in Tissue I. Models of radiation transport and their application. Lasers Med Sci, 6: 155–168.
Vo-Dinh T, 2014, Biomedical Photonics Handbook: Biomedical Diagnostics. United States: CRC Press.
DiPerna RJ, Lions PL, 1989, Ordinary differential equations, transport theory and Sobolev spaces. Invent Math, 98(3): 511–547. https://doi.org/10.1007/BF01393835
Rinzema K, Murrer LH, Star WM, 1998, Direct experimental verification of light transport theory in an optical phantom. J Opt Soc Am A, 15(8): 2078–2088.
Yavari N, 2006, Optical spectroscopy for tissue diagnostics and treatment control. Transport, 760: 141–157. https://doi.org/10.1007/978-1-61779-176-5
Xie D, Guo W, 2020, Measurement and calculation methods on absorption and scattering properties of turbid food in Vis/NIR range. Food Bioprocess Technol, 13(2): 229–244.https://doi.org/10.1007/s11947-020-02402-3
Noor SS, Michael K, Marshall S, et al., 2017, Hyperspectral image enhancement and mixture deep-learning classification of corneal epithelium injuries. Sensors, 17(11): 2644. https://doi.org/10.3390/s17112644
Palmer GM, Ramanujam N, 2006, Monte Carlo-based inverse model for calculating tissue optical properties. Part I: Theory and validation on synthetic phantoms. Appl Opt, 45(5): 1062–1071.
Alexandrakis G, Farrell TJ, Patterson MS, 1998, Accuracy of the diffusion approximation in determining the optical properties of a two-layer turbid medium. Appl Opt, 37(31): 7401–7409. https://doi.org/10.1364/ao.37.007401
Prahl SA, van Gemert MJ, Welch AJ, 1993, Determining the optical properties of turbid media by using the adding-doubling method. Appl Opt, 32(4): 559–568.
Wan S, Anderson RR, Parrish JA, 1981, Analytical modeling for the optical properties of the skin with in vitro and in vivo applications. Photochem Photobiol, 34(4): 493–499.
Hourdakis CJ, Perris A, 1995, A monte carlo estimation of tissue optical properties for use in laser dosimetry. Phys Med Biol, 40(3): 351–364. https://doi.org/10.1088/0031-9155/40/3/002
Jacques SL, Wang L, 1995, Monte Carlo modeling of light transport in tissues. In: Optical-Thermal Response of Laser- Irradiated Tissue. Berlin: Springer. p. 73–100.
Peng L, Chen W, Zhou W, et al., 2016, An immune-inspired semi-supervised algorithm for breast cancer diagnosis. Comput Methods Programs Biomed, 134: 259–265. https://doi.org/10.1016/j.cmpb.2016.07.020
Hossain S, Mohammadi FA, 2016, Tumor parameter estimation considering the body geometry by thermography. Comput Biol Med, 76: 80–93. https://doi.org/10.1016/j.compbiomed.2016.06.023
Hossain S, Mohammadi FA, Nejad ET, 2011, Neural network approach for the determination of heat source parameters from surface temperature image. In: 2011 24th Canadian Conference on Electrical and Computer Engineering (CCECE). IEEE. p. 1109–1112.
Pradipta AR, Tanei T, Morimoto K, et al., 2020, Emerging technologies for real‐time intraoperative margin assessment in future breast‐conserving surgery. Adv Sci (Weinh), 7(9): 1901519. https://doi/org/10.1002/advs.201901519
Halicek M, Fabelo H, Ortega S, et al., 2019, In-vivo and ex-vivo tissue analysis through hyperspectral imaging techniques: Revealing the invisible features of cancer. Cancers, 11(6): 756. https://doi.org/10.3390/cancers11060756
McCormack DR, Walsh AJ, Sit W, et al., 2014, In vivo hyperspectral imaging of microvessel response to trastuzumab treatment in breast cancer xenografts. Biomed Opt Express, 5(7): 2247–2261. https://doi.org/10.1364/BOE.5.002247
Kim B, Kehtarnavaz N, LeBoulluec P, Liu H, et al., 2013, Automation of ROI Extraction in Hyperspectral Breast Images. In: 2013 35th Annual International Conference of the IEEE Engineering in Medicine and Biology Society (EMBC). IEEE. p. 3658–3661.
Pourreza-Shahri R, Saki F, Kehtarnavaz N, Leboulluec P, et al., 2013, Classification of ex-vivo Breast Cancer Positive Margins Measured by Hyperspectral Imaging. In: 2013 IEEE International Conference on Image Processing, ICIP 2013-Proceedings. p. 1408–1412. https://doi.org/10.1109/ICIP.2013.6738289
Sterenborg HJ, Kho E, de Boer LL, et al., 2014, Hyperspectral imaging for intraoperative margin assessment during breast cancer surgery. Opt InfoBase Conf Papers, 2016: 6–9. https://doi.org/10.1364/ACPC.2016.AF1K.6
Bakhshandeh M, Tutuncuoglu SO, Fischer G, et al., 2007, Use of imprint cytology for assessment of surgical margins in lumpectomy specimens of breast cancer patients. Diagn Cytopathol, 35(10): 656–659.
Coudray N, Ocampo PS, Sakellaropoulos T, et al., 2018, Classification and mutation prediction from non–small cell lung cancer histopathology images using deep learning. Nat Med, 24(10): 1559–1567.
Kim SH, Cornacchi SD, Heller B, et al., 2013, An evaluation of intraoperative digital specimen mammography versus conventional specimen radiography for the excision of nonpalpable breast lesions. Am J Surg, 205(6): 703–710. https://doi/org/10.1016/j.amjsurg.2012.08.010
Ha R, Friedlander LC, Hibshoosh H, et al., 2018, Optical coherence tomography: A novel imaging method for post-lumpectomy breast margin assessment-a multi-reader study. Acad Radiol, 25(3): 279–287. https://doi.org/10.1016/j.acra.2017.09.018
Wong TT, Zhang R, Hai P, et al., 2017, Fast label-free multilayered histology-like imaging of human breast cancer by photoacoustic microscopy. Sci Adv, 3(5): e1602168. https://doi.org/10.1126/sciadv.1602168
Moschetta M, Telegrafo M, Introna T, et al., 2015, Role of specimen US for predicting resection margin status in breast conserving therapy. G Chir, 36(5): 201–204. https://doi.org/10.11138/gchir/2015.36.5.201
Tang R, Saksena M, Coopey SB, et al., 2016, Intraoperative micro-computed tomography (micro-CT): A novel method for determination of primary tumour dimensions in breast cancer specimens. Br J Radiol, 89(1058): 20150581.
Schnabel F, Boolbol SK, Gittleman M, et al., 2014, A randomized prospective study of lumpectomy margin assessment with use of MarginProbe in patients with nonpalpable breast malignancies. Ann Surg Oncol, 21(5): 1589–1595. https://doi.org/10.1245/s10434-014-3602-0
Dixon JM, Renshaw L, Young O, et al., 2016, Intra-operative assessment of excised breast tumour margins using ClearEdge imaging device. Eur J Surg Oncol, 42(12): 1834–1840. https://doi.org/10.1016/j.ejso.2016.07.141
Ortega S, Halicek M, Fabelo H, et al., 2020, Hyperspectral imaging and deep learning for the detection of breast cancer cells in digitized histological images. Proc SPIE Int Soc Opt Eng, 11320: 206–214.